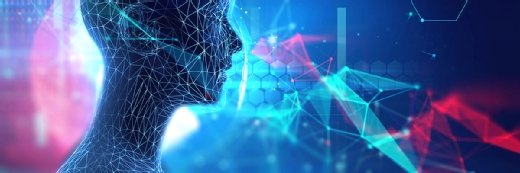
Getty Images
Navigating the black box AI debate in healthcare
How concerned should healthcare stakeholders be about the complexity and lack of transparency in black box artificial intelligence tools?
Artificial intelligence (AI) is taking the healthcare industry by storm as researchers share breakthroughs and vendors rush to commercialize advanced algorithms across various use cases.
Terms like machine learning, deep learning and generative AI are becoming part of the everyday vocabulary for providers and payers exploring how these tools can help them meet their goals; however, understanding how these tools come to their conclusions remains a challenge for healthcare stakeholders.
Black box software — in which an AI’s decision-making process remains hidden from users — is not new. In some cases, the application of these models may not be an issue, but in healthcare, where trust is paramount, black box tools could present a major hurdle for AI deployment.
Many believe that if providers cannot determine how an AI generates its outputs, they cannot determine if the model is biased or inaccurate, making them less likely to trust and accept its conclusions.
This assertion has led stakeholders to question how to build trust when adopting AI in diagnostics, medical imaging and clinical decision support. Doing so requires the healthcare industry to explore the nuances of the black box debate.
In this primer, HealthITAnalytics will outline black box AI in healthcare, alternatives to the black box approach and the current AI transparency landscape in the industry.
CONCERNS ABOUT BLACK BOX AI
One of the major appeals of healthcare AI is its potential to augment clinician performance and improve care, but the black box problem significantly inhibits how well these tools can deliver on those fronts.
Research published in the February 2024 edition of Intelligent Medicine explores black box AI within the context of the “do no harm” principle laid out in the Hippocratic Oath. This fundamental ethical rule reflects a moral obligation clinicians undertake to prevent unnecessary harm to patients, but black box AI can present a host of harms unbeknownst to both physicians and patients.
“[Black box AI] is problematic because patients, physicians, and even designers do not understand why or how a treatment recommendation is produced by AI technologies,” the authors wrote, indicating that the possible harm caused by the lack of explainability in these tools is underestimated in the existing literature.
In the study, the researchers asserted that the harm resulting from medical AI's misdiagnoses may be more serious, in some cases, than that caused by human doctors’ misdiagnoses, noting that the “unexplainability” feature of such systems limits patient autonomy in shared decision-making and black box tools can create significant psychological and financial burdens for patients.
Questions of accountability and liability that come from adopting black box solutions may also hinder the proliferation of healthcare AI.
To tackle these concerns, many stakeholders across the healthcare industry are calling for the development and adoption of ‘explainable’ AI algorithms.
THE PUSH FOR EXPLAINABLE AI
Explainable AI (XAI) refers to “a set of processes and methods that allows human users to comprehend and trust the results and output created by machine learning algorithms,” according to IBM. “[Explainability] is used to describe an AI model, its expected impact and potential biases. It helps characterize model accuracy, fairness, transparency and outcomes in AI-powered decision making.”
Having insights into these aspects of an AI algorithm, particularly in healthcare, can help ensure that these solutions meet the industry’s standards.
Explainability can be incorporated into AI in a variety of ways, but clinicians and researchers have outlined a few critical approaches to XAI in healthcare in recent years.
A January 2023 analysis published in Sensors indicates that XAI techniques can be divided into categories based on form, interpretation type, model specificity and scope. Each methodology has pros and cons depending on the healthcare use case, but applications of these approaches have seen success in existing research.
A research team from the University of Illinois Urbana–Champaign’s Beckman Institute for Advanced Science and Technology writing in IEEE Transactions on Medical Imaging demonstrated that a deep learning framework could help address the black box problem in medical imaging.
The researchers’ approach involved a model for identifying disease and flagging tumors in medical images like X-rays, mammograms and optical coherence tomography (OCT). From there, the tool generates a value between zero and one to denote the presence of an anomaly, which can be used in clinical decision-making.
However, alongside these values, the model also provides an equivalency map (E-map) — a transformed version of the original medical image that highlights medically interesting regions of the image — which helps the tool “explain” its reasoning and enables clinicians to check for accuracy and explain diagnostic findings to patients.
Other approaches to shed light on AI’s decision-making have also been proposed.
In a December 2023 Nature Biomedical Engineering study, researchers from Stanford University and the University of Washington outlined how an auditing framework could be applied to healthcare AI tools to enhance their explainability.
The approach utilizes a combination of generative AI and human expertise to assess classifiers — an algorithm used to categorize data inputs.
When applied to a set of dermatology classifiers, the framework helped researchers identify which image features had the most significant impact on the classifiers’ decision-making. This revealed that the tools relied on both undesirable features and features leveraged by human clinicians.
These insights could aid developers looking to determine whether an AI relies too heavily on spurious data correlations and correct those issues before deployment in a healthcare setting.
Despite these successes in XAI, there is still debate over whether these tools effectively solve the black box problem or whether black box algorithms are a problem.
THE CURRENT AI TRANSPARENCY LANDSCAPE
While many in the healthcare industry maintain that black box algorithms are a major concern and discourage their use, some have raised questions about the nuances of these assertions. Others posit that the black box problem is an issue but indicate that XAI is not a one-size-fits-all solution.
One central talking point in these debates revolves around the use of other tools and technologies in healthcare that could be conceptualized as black box solutions.
“Although [the black box AI] discussion is ongoing, it is worth noting that the mechanism of action of many commonly prescribed medications, such as Panadol, is poorly understood and that the majority [of] doctors have only a basic understanding of diagnostic imaging tools like magnetic resonance imaging and computed tomography,” explained experts writing in Biomedical Materials & Devices.
While not all healthcare tools are necessarily well-understood, such solutions can be contentious in evidence-based medicine, which prioritizes the use of scientific evidence, clinical expertise and patient values to guide care.
“Some have suggested that the ‘black-box’ problem is less of a concern for algorithms used in lower-stakes applications, such as those that aren’t medical and instead prioritize efficiency or betterment of operations,” the authors noted.
However, AI is already being used for various tasks, including decision support and risk stratification, in clinical settings, raising questions about who is responsible in the event of a system failure or error associated with using these technologies.
Explainability has been presented as a potential method to ease concerns about responsibility, but some researchers have pointed out the limitations of XAI in recent years.
In a November 2021 viewpoint published in the Lancet Digital Health, researchers from Harvard, the Massachusetts Institute of Technology (MIT) and the University of Adelaide argued that assertions about XAI’s potential to improve trust and transparency represent “false hope” for current explainability methods.
The research team asserted that black box approaches are unlikely to achieve these goals for patient-level decision support due to issues like interpretability gaps, which characterize an aspect of human–computer interaction wherein a model presents its explanation, and the human user must interpret said explanation.
“[This method] relies on humans to decide what a given explanation might mean. Unfortunately, the human tendency is to ascribe a positive interpretation: we assume that the feature we would find important is the one that was used,” the authors explained.
This is not necessarily the case, as there can be many features — some invisible to humans — that a model may rely on that could lead users to form an incomplete or inaccurate interpretation.
The research team further indicated that model explanations have no performance guarantees, opening the door for other issues.
“[These explanations] are only approximations to the model's decision procedure and therefore do not fully capture how the underlying model will behave. As such, using post-hoc explanations to assess the quality of model decisions adds another source of error — not only can the model be right or wrong, but so can the explanation,” the researchers stated.
A 2021 article published in Science echoes these sentiments, asserting that the current hype around XAI in healthcare “both overstates the benefits and undercounts the drawbacks of requiring black-box algorithms to be explainable.”
The authors underscored that for many applications in medicine, developers must use complicated machine learning models that require massive datasets with highly engineered features. In these cases, a simpler, interpretable AI (IAI) model couldn’t be used as a substitute. XAI provides a secondary alternative, as these models can approach the high level of accuracy achieved by black box tools.
But here, users still face the issue of post-hoc explanations that may make them feel as though they understand the model’s reasoning without actually shedding light on the tool’s inner workings.
In light of these and other concerns, some have proposed guidelines to help healthcare stakeholders determine when it is appropriate to use black box models with explanations rather than IAI — such as when there is no meaningful difference in accuracy between an interpretable model and black box AI.
The debate around the use of black box solutions and the role of XAI is not likely to be resolved soon, but understanding the nuances in these conversations is vital as stakeholders seek to navigate the rapidly evolving landscape of AI in healthcare.