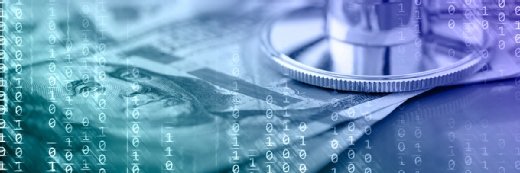
Viorika/istock via Getty Images
Investigating electronic phenotyping’s role in clinical analytics
Electronic phenotyping has significant potential to drive EHR-based data mining and clinical research, but patient privacy remains a major concern.
As healthcare organizations gather more clinical data, extracting value from that information has become a top priority. However, to make this data actionable for research and development, health systems must collect, process and mine it to identify trends and patterns that could advance strategic goals.
The data mining process is key to generating insights that drive healthcare analytics projects, but it is only the beginning. After datasets are created and mined, stakeholders may also want to pursue electronic phenotyping — a process that can help bolster clinical and translational research efforts by allowing users to query healthcare data.
This primer will explore electronic phenotyping, its potential and pitfalls in healthcare and potential use cases.
WHAT IS ELECTRONIC PHENOTYPING?
Penn Medicine indicates that “electronic phenotyping involves querying electronic health record systems (EHRs) and other clinical information systems and databases to identify patients who meet a specific set of clinical characteristics.”
In clinical research, teams can use electronic phenotyping to support hypothesis generation, research planning, grant preparation, data gathering, hypothesis testing, clinical trial recruitment and research registry development, among other use cases.
Querying information systems allows users to identify patients with shared phenotypes — observable traits determined by genetic and environmental factors. In electronic phenotyping, the phenotype of interest is typically the presence of a particular disease or condition.
The National Institutes of Health (NIH) Pragmatic Trials Collaboratory notes that “in the context of [EHRs], a ‘computable phenotype,’ or simply ‘phenotype,’ is a clinical condition or characteristic that can be ascertained by means of a computerized query to an EHR system or clinical data repository using a defined set of data elements and logical expressions. These queries can identify patients with particular conditions and can be used to support a variety of purposes, including population management, quality measurement, and observational and interventional research.”
The significant potential of electronic phenotyping has led to the development of a host of EHR-based automatic phenotyping approaches for use on both structured and unstructured data.
However, before pursuing an electronic phenotyping project, stakeholders should be prepared to consider the opportunities and shortcomings the approach presents.
PROS AND CONS
Electronic phenotyping is a desirable strategy because it can take advantage of one of healthcare’s most valuable data sources: EHRs. Patients’ records provide a wealth of insights that, if utilized effectively, can bolster efforts to improve care delivery and health outcomes.
But EHRs contain many types of data in various formats, from medical images to clinicians’ notes. Sifting through this information is resource- and time-intensive, but the advent of phenotyping algorithms helped overcome these challenges.
Researchers writing in the Annual Review of Biomedical Data Science in 2018 explained that “finding patients with specific conditions or outcomes, known as phenotyping, is one of the most fundamental research problems encountered when using these new EHR data. Phenotyping forms the basis of translational research, comparative effectiveness studies, clinical decision support and population health analyses using routinely collected EHR data.”
Despite this, electronic phenotyping has limitations.
The NIH Pragmatic Trials Collaboratory further emphasizes that computable phenotypes are proxies for establishing the absence or presence of a characteristic, and they are based on observations made by a patient’s care team.
The organization notes that the information found in a patient’s EHR may reflect disease status, but the data is generated via the perceptions and interpretations of the clinician observing the patient. Thus, the data is inherently limited by those constraints, often making it incomplete or biased.
Further, EHRs with a wealth of data are only available for individuals willing and able to access healthcare. This excludes a large number of people, many of whom are part of minoritized groups, from being included in EHR-based research unless health systems work actively to improve care access and serve those individuals.
This phenomenon has far-reaching impacts on health equity and is part of a broader set of concerns about underrepresentation in biomedical research. Addressing this problem is necessary to promote improved outcomes for all, and incorporating data from these populations has been shown to do so.
Anatomy- and gender-based EHR integrations can boost care quality and clinical decision support for transgender and gender-diverse patients, while sexual orientation and gender identity (SO/GI) EHR documentation practices improve documentation and health equity.
However, electronic phenotyping can contribute to unintentional data disclosures, presenting privacy concerns for gender-marginalized patients and others from vulnerable groups.
In an October 2023 interview with HealthITAnalytics, leadership from the University of Pennsylvania and Children's Hospital of Philadelphia detailed how electronic phenotyping can reveal sensitive patient characteristics not disclosed to a clinician, such as transgender identity.
Such revelations raise ethical concerns around data use, patient consent and clinician bias, but capturing this information plays an important role in improving care and tackling health disparities.
Access to high-quality, gender-affirming care demonstrably improves health outcomes, but the politicization of healthcare for members of the LGBTQ+ community adds another layer to the conversation around the risks of electronic phenotyping.
In an effort to improve care and reduce potential harm to transgender and other gender-marginalized patients, experts recommend involving members of the community when considering an electronic phenotyping project.
High-quality care delivery for these patients also requires providers to exhibit empathy and cultural competence, which healthcare organizations must actively foster through policy, training and advocacy to combat poor patient experiences.
Despite these hurdles, electronic phenotyping has been deployed for various applications.
USE CASES
Electronic phenotyping is particularly useful for identifying groups of patients with shared medical conditions for research purposes.
Stroke classification
A 2020 study published in Stroke demonstrated the utility of the approach for classifying ischemic stroke.
The authors noted that differentiating between stroke types is key for successful risk stratification, as oral anticoagulation is indicated for cardioembolic strokes but not strokes of other types. However, manual stroke classification is time-intensive, necessitating more efficient approaches.
The researchers successfully developed an electronic phenotyping methodology for this application, using administrative codes and echocardiogram data from within EHRs to develop algorithms to flag cardioembolic stroke.
Identifying HIV
A team writing in JMIR Formative Research in 2021 detailed the development of an electronic phenotyping algorithm to identify individuals with human immunodeficiency virus (HIV) and improve risk assessment.
The researchers indicated that flagging these patients is critical to better understanding HIV-related outcomes and bolstering care quality. While phenotyping algorithms for this purpose exist, the authors emphasized that the tools rely on a combination of lab test results, HIV diagnostic guidelines and International Classification of Disease codes.
Yet these tools missed a significant portion of HIV patients when tested.
To address this, the research team built HIV-Phen, an electronic phenotyping algorithm that significantly outperformed existing tools by incorporating HIV-specific laboratory tests and medication data.
Informing pragmatic clinical trials
Pragmatic clinical trials (PCTs) present a unique opportunity to bridge the gap between knowledge generation and improved care. This type of research focuses on embedding clinical trials within the healthcare delivery system rather than having separate standalone systems for research and care, which reflects the current framework for randomized controlled trials (RCTs).
PCTs and RCTs both serve to create generalizable knowledge around biological or mechanistic hypotheses, such as medical treatments and interventions. However, PCTs have the potential to speed advancements in healthcare delivery in a way that traditional RCTs cannot by being conducted in real-world settings.
Since PCTs rely on real-world patient data, these trials take advantage of EHRs to inform research. Electronic phenotyping can help make the use of this data more efficient, allowing researchers to identify computable phenotypes and create study cohorts.