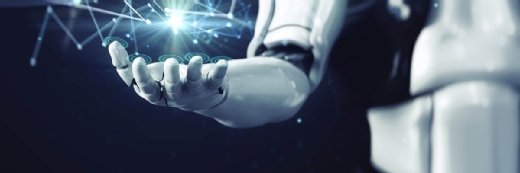
Getty Images
How Big Data Analytics Can Support Preventive Health
Big data analytics are being incorporated into all aspects of healthcare, but how can they be used to bolster preventive medicine and care?
The healthcare sector is an increasingly complex system that incorporates various stakeholders outside of clinicians and patients. This complexity generates significant amounts of data across the continuum of care. To promote health outcomes and prevent disease, this data must be collected, stored, shared, and analyzed effectively. But the sheer amount of data makes this a formidable challenge.
To address this issue, many healthcare stakeholders are turning to big data analytics. Big data analytics provide enhanced data-related capabilities to healthcare providers, which they can use to support large-scale goals and health outcomes.
Currently, big data analytics are being used extensively to enhance preventive health.
IDENTIFYING BIOMARKERS
A biomarker is defined by the US Food & Drug Administration (FDA) as “a defined characteristic that is measured as an indicator of normal biological processes, pathogenic processes, or responses to an exposure or intervention, including therapeutic interventions.” There are various types of biomarkers, but many are used to predict and prevent disease.
In an ongoing study that began earlier this year, researchers from the Cleveland Clinic aim to identify brain disease biomarkers early to prevent neurological illness before any symptoms occur.
The researchers note that one in every six people worldwide has a neurological disorder, and these diseases are often difficult to predict early on. Clinicians are also unable to stop or slow the progression of most brain disorders, providing strong incentives to develop early prediction and prevention methods.
The Cleveland Clinic Brain Study contributes to these efforts by gathering data from 200,000 neurologically healthy patients over 20 years to detect brain disease biomarkers. Researchers hypothesize that these biomarkers and other targets may help prevent or cure neurological disorders.
Study volunteers will participate in annual assessments that can help shed light on their cognitive function, including bloodwork, eye retina scans, electroencephalograms (EEGs), brain MRIs, sleep studies, and neurological examinations.
These data will then be fed to advanced data analytics tools that can identify genetic risk factors or “disease fingerprints” that will guide preventive and diagnostic strategies.
FINDING GAPS IN CHRONIC DISEASE MANAGEMENT CARE
Effective chronic disease management requires clinicians to use a wide range of healthcare data to monitor patient health, allocate resources, and measure outcomes. Big data analytics make this information more accessible by bringing it together at the point of care.
By using big data analytics for chronic disease management, clinicians may be able to identify high-risk patients and intervene to prevent chronic disease occurrence or progression. Artificial intelligence (AI), for example, is being researched for use in guiding treatment decision-making through gathering, categorizing, and analyzing the massive amounts of data generated by chronic disease treatments.
In one study published by the University of Southern Queensland, researchers evaluating the effectiveness of big data analytics in care management of type 2 diabetes mellitus (T2DM) found that treatment of the condition generated a plethora of structured, semi-structured, and unstructured data. The structured data was mostly demographic information, while the semi-structured data included prescriptions, reports, symptoms, and diagnoses, among other factors. The unstructured data included video, audio, and other multimedia data.
The researchers posited that AI could have a major impact on T2DM management if used to deal with these large amounts of data.
However, gaps in the research about the factors that impact the usability of big data analytics for T2DM remained. To combat this, the researchers developed a model to identify these factors and measure their usability as they relate to T2DM management. The model is still being studied, but the researchers indicated that the validation and implementation of the model could have significant potential for clinical use.
ENHANCING POPULATION HEALTH
Population health management is a challenge in large part because of its scale. Successful strategies require that populations in need of care are identified, previously provided care is measured, and future care delivery is evaluated. Here, the implementation of big data analytics seems like an obvious choice, but knowing how to use the vast, highly varied sets of data can prove challenging.
Some healthcare providers are focusing their big data efforts on understanding patient metrics and developing risk scores for use in prediction models. In doing so, providers can gain insight into patient needs and use that information to guide resource allocation and management.
Others are moving toward value-based care. Value-based care aims to enhance patient experience, population health, and costs of care, which can lead to successful, more effective wellness and prevention strategies. There is evidence that real-time data improve value-based care. Data can also be used to assess value-based care and help with the staffing model shifts that may be needed to accommodate a value-based care strategy.
Big data also plays a role in addressing social determinants of health (SDOH). SDOH are factors outside those traditionally evaluated in the healthcare setting that impact an individual's health. Providers increasingly consider factors like the environment, social isolation, or food insecurity when treating patients.
By measuring these social determinants, a provider can better understand how they impact health in a given population and capture information that can help improve outcomes. Some payers and employers have utilized SDOH data to improve wellness programs, which have significant potential to support preventive health initiatives led by providers.
SUPPORTING GENOMICS
Genomic sequencing is becoming more common as healthcare providers seek to enhance routine clinical care. Like cholesterol checks and cancer screenings, DNA screening has the potential to advance precision medicine and improve health outcomes by identifying patients at high risk for certain conditions.
In May 2018, Geisinger announced that it would incorporate genomic sequencing into routine clinical care to increase the amount of relevant data used to support disease prevention and management. Geisinger has since expanded the program, launching it in additional clinics and testing for a wider array of genes.
As of November 2019, Geisinger reported that 2 to 4 percent of patients tested will present a genetic mutation that causes heart disease, cancer, or another disorder. Healthcare consumers appear interested in AI and genetic testing for precision medicine, but concerns about the costs have been raised.
Genomic sequencing programs that are implemented into routine clinical care, like at Geisinger, have the potential to reduce these costs. However, making DNA screening more easily accessible does not necessarily translate to clinicians being able to deliver accurate genetic counseling. Healthcare providers that offer genomic sequencing as part of routine care must also ensure that clinicians can effectively interpret and communicate results with their patients to use this data for preventive health successfully.