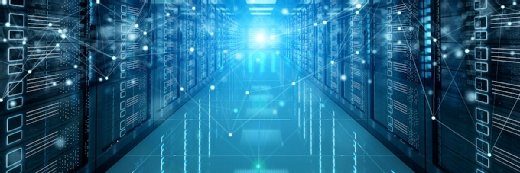
Getty Images/iStockphoto
Health Information Governance Strategies for Unstructured Data
Information governance becomes particularly important when exploring the use of unstructured data for healthcare analytics.
While electronic health records still have the potential to standardize care by enabling advanced analytics and informing clinical decision-making, much of the data held within these systems – and a large proportion of the data used in conjunction with these basic health IT tools – is currently unstructured, and likely to remain that way.
Unstructured data takes many forms, from x-ray images and faxed lab reports to email communications, clinical notes, and even patient phone calls. None of this data is easily corralled into a format that can be fed into algorithms and fed back to clinicians in an intuitive manner, yet providers desperately need better ways to understand and leverage these critical sources of information to care for their patients.
As the long march towards accountable care makes it increasingly important to integrate non-traditional data sources into population health management programs, risk stratification strategies, and patient engagement programs, healthcare organizations need to better understand the potential of these datasets and how to use these rich streams of information to enhance the patient experience.
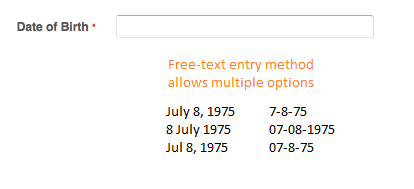
Where can healthcare organizations find unstructured data?
Unstructured data is any piece of information that does not adhere to a pre-defined model or organizational framework. Most types of information, including names, dates, diagnoses, and medications, can be represented in multiple ways and recorded in myriad formats.
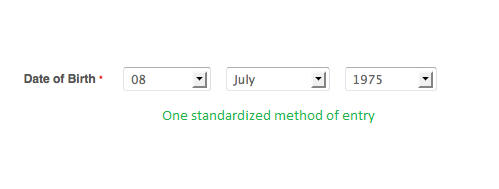
When implementing data systems, organizations can choose how they wish to capture and store these elements by employing standardized data fields for user input. This process is at the crux of any meaningful data governance plan.
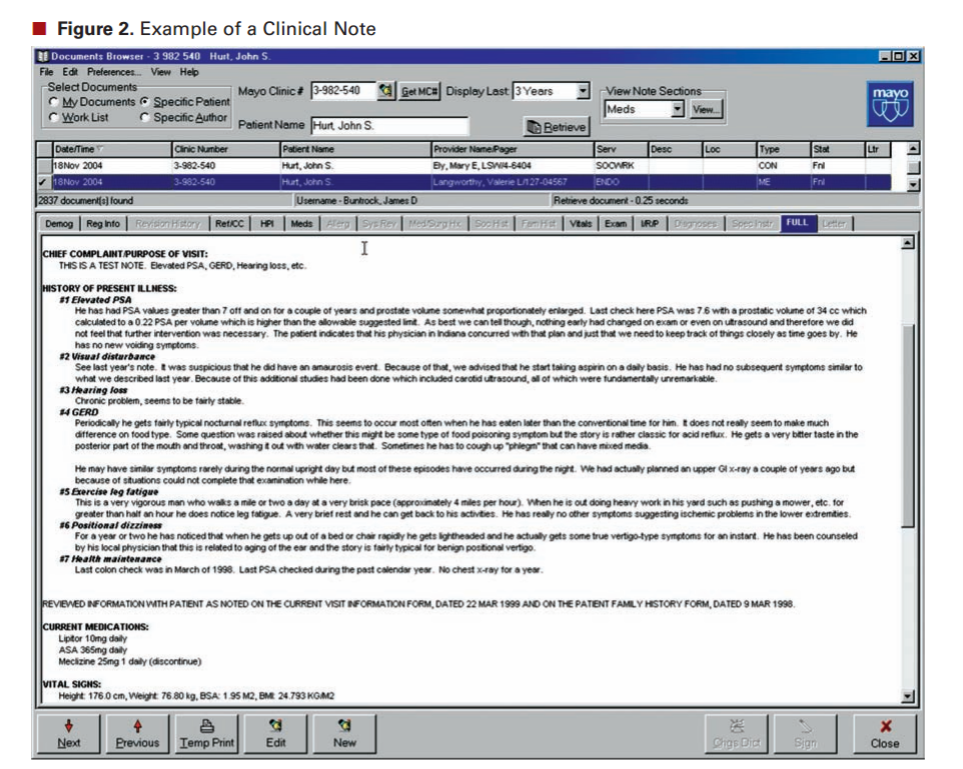
While structured input options, including drop-down menus, radio buttons, and check boxes, are often extremely helpful for basic data fields including birth dates, patient gender, and questions with yes or no answers, they can fall short for more complex data capture needs.
Providers often turn first to their clinical notes or other EHR free-text input fields when discussing non-standardized data, and with good reason. Much of the patient story is included in these narratives, and providers often note down key lifestyle factors, socioeconomic data, clinical suspicions, patient preferences, and other information that cannot be recorded in the EHR any other way.
The problem is that without a hard-coded way to represent this information, it is often lost to analytics algorithms that can only incorporate standardized representations of ideas.
Medical coding systems, including the Current Procedural Terminology (CPT) and International Classification of Diseases (ICD) code sets, were created to solve this problem by providing clinicians, payers, and revenue cycle management staff with a widely accepted methodology for representing core clinical concepts.
Ideally, every piece of clinically relevant information found in a free-text note can and should be translated into a standard code, which can then be used for claims, billing purposes, and clinical analytics.
In reality, however, code sets have their limitations. And as the definition of “clinically relevant” expands with the acceptance of the fact that the vast majority of a patient’s health has nothing to do with provider-based care, those limitations are becoming increasingly pronounced.
The growing need to know more and more about the patient, her environment, her choices, and her needs means that the problematic free-text note is just the tip of the unstructured data iceberg.
As patients become more involved in their care and analytics capabilities continue to advance, the type and scope of data available for clinical decision-making is growing at an unprecedented rate, and may include:
Patient-generated health data
Whether it’s readouts from Internet of Things devices, hand-written comment cards, wellness diary entries recorded on the patient portal, or a scrap of paper with a list of vitamin supplements and over-the-counter medications, patients produce a huge volume of data that is not always easy to capture in the traditional EHR.
These alternative data sources can help organizations understand the entirety of the patient experience, both inside the clinic and during the rest of their lives. Developing strategies for recording patient-generated health data in a standardized way, and delivering that information to clinicians at the point of care, has become a top priority for many providers.
Imaging test results
Imaging analytics is an emerging area of interest for cutting-edge data scientists, and for good reason. While radiologists do an excellent job of scanning x-rays and MRIs for targeted abnormalities, these test results may also include additional information about a patient’s health status, including emerging conditions that might escape a human focused on diagnosing an unrelated condition.
Using machine learning and pattern recognition techniques, some organizations are working to extract the untapped information in these files to support precision medicine projects, clinical decision support tools, and more proactive, preventive care.
Phone calls, photographs, and video telehealth consults
Patients dialing into call centers may be very familiar with the idea that their call could be recorded for training purposes, but those audio files could also be helping healthcare organizations improve their administrative efficiency.
Wait times, staff courtesy levels, ease of navigating the system, and outcomes of the conversation are all significant measures of patient satisfaction. And as organizations invest more in afterhours nursing lines or triage services to expand access and keep patients out of the office, these calls take on additional importance for understanding how, when, and why patients interact with their providers.
The growing popularity of telehealth adds another dimension to this type of data: audio, video, and still images from remote consults can contain critical insights into patient wellness or the development of a disease, and could be mined in a similar manner to formal imaging studies for details about diagnostics and patient care.
PDFs, faxes, paper records, and snail-mail letters
Lab reports, visit summaries from specialists, patient records from non-EHR users, payer authorization letters, and numerous other communications are often attached as PDFs to emails, mailed or sent by courier, faxed between providers, or even carried into the office by patients themselves.
A physician or nurse might leaf through these paper documents or scan the static images for relevant information, but chances are that they will not sit down and take notes on every page of a new patient’s file when she brings a stack of manila folders over from her old clinician…the one she had been seeing three times a year for the past two decades.
Important information may be hiding among those routine care summaries and illegibly scribbled copies of prescriptions, but the man-hours required to extract those nuggets of data render the task unrealistic on a large scale.
Preventing Big Data Pain Points During a Healthcare Encounter
The Difference Between Big Data and Smart Data in Healthcare
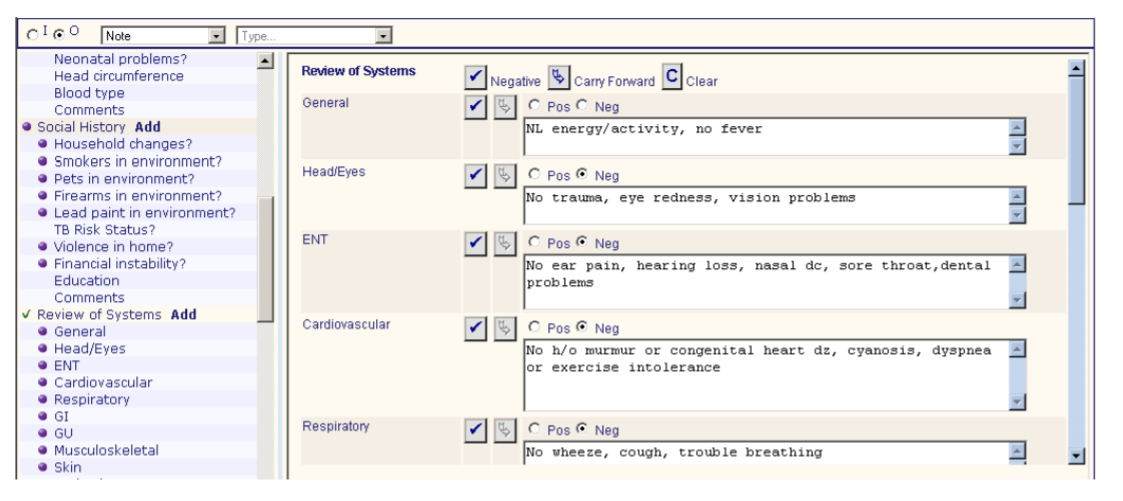
Options for structuring clinical data in the EHR
Just because a dataset is currently unstructured doesn’t mean it has to stay that way. As organizations develop their EHR workflows, they can take advantage of customization options that may help them balance structured and free-text input according to their providers’ needs.
EHRs typically offer the ability to develop templates to standardize common tasks and collect data for quality reporting purposes.
While some physicians may balk at the idea that the patient story should be reduced to click boxes and dropdown menus, others argue that templates can guide providers during patient assessments, help them conduct complete and thorough examinations, and make the results of every visit more accessible for organizational improvement efforts down the line.
Well-designed templates can offer the best of both worlds by blending some free-text options with structured input fields, allowing users to capture unique patient features alongside basic data.
In an example provided by the Agency for Healthcare Quality and Research (AHRQ), a template for pediatric patient visits uses both types of data fields to create a meaningful record.
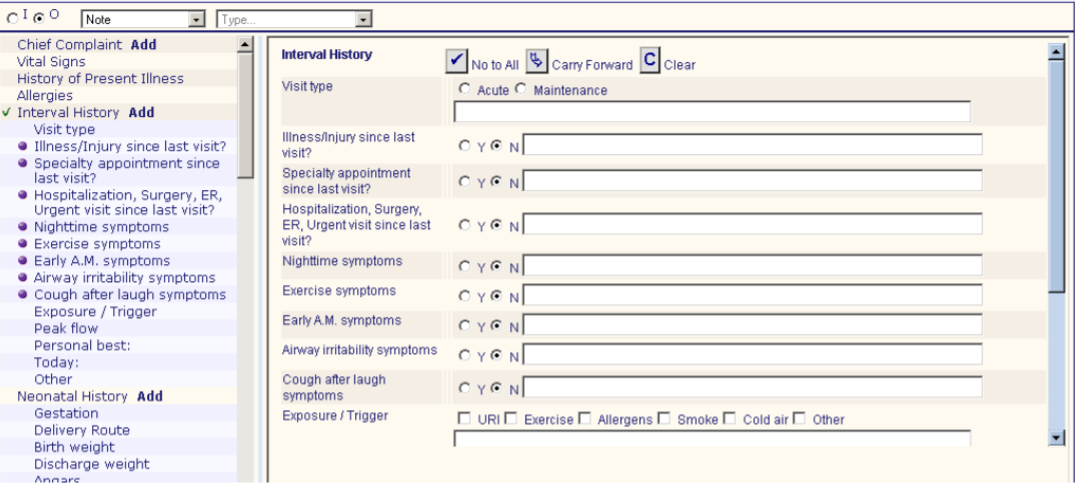
Templates can cover general wellness visits or condition-specific situations, such as a visit related to the child’s asthma.
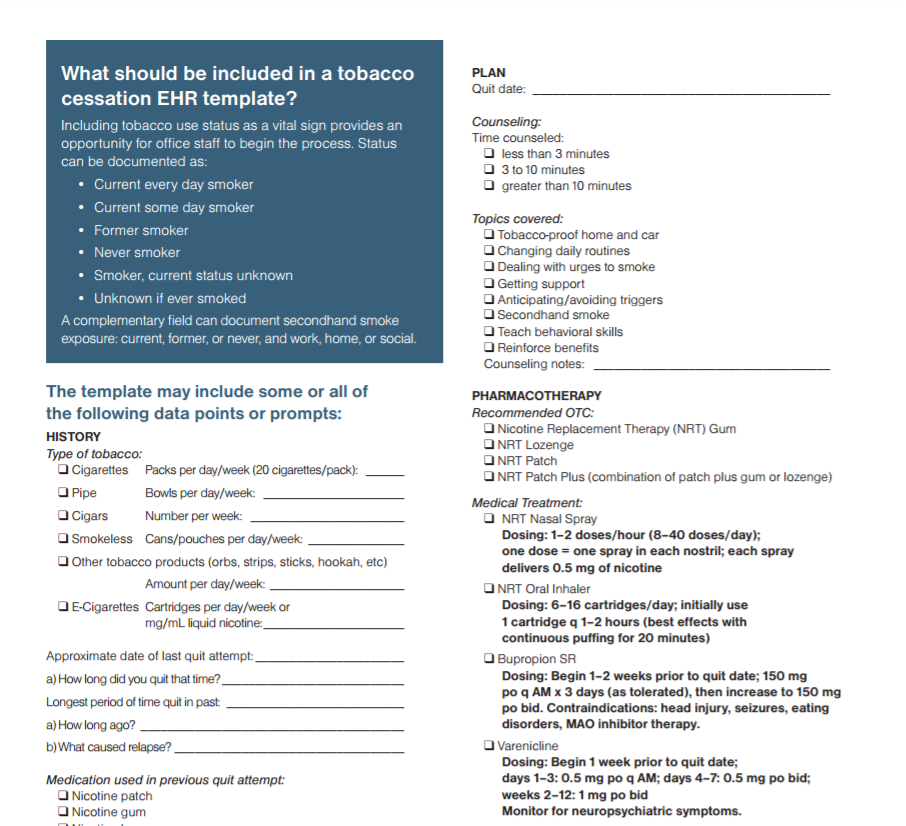
Since asthma control metrics appear often in the various clinical quality measure sets used to gauge provider performance, organizations may benefit from ensuring that their clinicians are following standardized protocols for asthma care.
Analysts can easily extract the structured data from the template to generate performance reports, allowing organizations to benchmark their providers and target quality improvement efforts.
The American Academy of Family Physicians (AAFP) also promotes checklists and templates as a way to standardize care processes and collect clean data for performance reporting.
Smoking cessation, for instance, is an important population health metric that can have wide-ranging impacts on a patient’s health – it is also an optional quality measure for meaningful use.
While it may seem difficult to condense a patient’s long-term struggles with quitting cigarettes into a simple checklist, key concepts don’t tend to vary enormously from individual to individual.
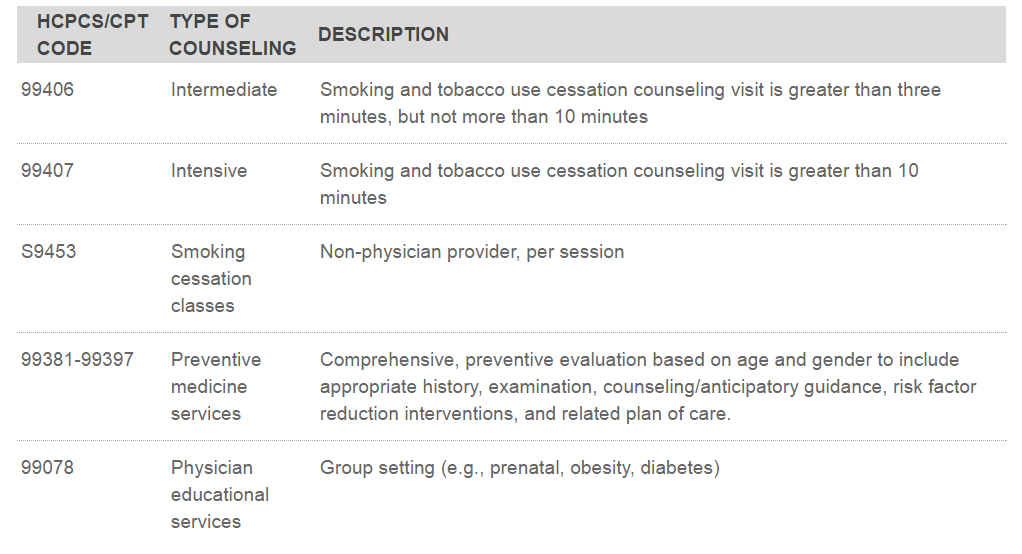
Designing a template based on these suggestions – and using drop-down menus or similar hard-coded selection options for the dates and other numerical values – can simplify quality reporting for incentive programs or value-based care initiatives.
It can also speed up the claims submissions process. The AAFP’s breakdown correlates exactly to the tobacco-related CPT codes used for billing purposes.
Using structured data in these instances can same time and effort by reducing the need for coders to open up queries that can slow claims processing and delay reimbursement for patient services.
What Is EHR Optimization, How Does It Start?
Why a Thin Line Separates EHR Optimization, EHR Replacement
Tools to extract information from unstructured datasets
But EHR templates will not help providers with data that isn’t created within the EHR. Those PDFs, images, hand-written documents, and audio recordings cannot be easily transformed into usable data without a set of automated tools that can extract and translate information into a new format.
Natural language processing (NLP) is a rapidly developing area of machine learning that can help to solve the unstructured data problem. NLP tools can identify key syntactic structures in free text and extract the meaning behind the narrative. The results can be used to generate new documents, like a clinical visit summary, or can be translated into codes for billing purposes.
NLP is also used in speech recognition software to allow providers to dictate clinical notes that can be turned into text documents or mapped to standardized data elements for documentation and coding.
Optical character recognition (OCR) software can supplement the standardization process by turning static images, like PDFs or paper documents, into machine-readable text. OCR tools can usually recognize handwriting as well as computer fonts, allowing providers to extract the important parts of patient’s history from notes generated during the pre-EHR era without spending hours poring over the pages themselves.
The unique content and complexity of clinical documentation can be challenging for many NLP developers, but keen interest in emerging machine learning and artificial intelligence strategies are helping to refine the industry’s information processing capabilities.
The market for natural language processing and other pattern recognition tools is predicted to grow at a steady rate over the next four to five years, reaching a value of $2.65 billion by 2021, says a recent estimate by ReportsnReports.
Imaging analytics tools, which also fall under the pattern recognition and machine learning umbrella, represent a similarly promising opportunity for health IT developers. In 2015, IBM spent $1 billion to acquire medical imaging company Merge Healthcare, and has since joined a multi-stakeholder collaborative focused on bringing imaging analytics to the provider environment.
Other corporate investors, including Microsoft and GE, are also working with providers and industry partners to develop tools that can enhance the diagnostic capability of medical imaging and deliver clinical decision support to users.
Eventually, the untapped wealth of information in images, audio, video, narrative text, environmental data, and other unstructured datasets will be able to feed advanced artificial intelligence programs that could provide critical insights into a wide variety of patient care concerns.
To prepare for a healthcare environment driven by machine learning and unstructured data, providers may wish to begin developing the data governance principles that will allow them to succeed with quality reporting now and allow them to move into more advanced healthcare analytics in the near future.
The Role of Healthcare Data Governance in Big Data Analytics
How Healthcare Can Prep for Artificial Intelligence, Machine Learning