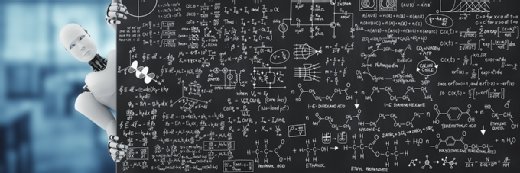
Blue Planet Studio - stock.adobe
Fostering health AI development with confidential computing
Can confidential computing streamline clinical algorithm development by providing a secure collaborative environment for data stewards and AI developers?
The development of AI solutions to tackle pain points in healthcare is an exciting prospect for many in the industry. While other industries are seeing rapid advances in algorithm development, healthcare AI innovation is often slowed by data privacy and cost concerns.
Confidential computing has been proposed to help secure healthcare data during analytics projects, but until recently, there have been limited applications outside of research.
In 2020, the University of California, San Francisco (UCSF) Center for Digital Health Innovation (CDHI), Fortanix, Intel and Microsoft Azure formed a collaboration to create a privacy-preserving confidential computing platform to accelerate clinical algorithm development and validation.
The work, which has since been spun out into a startup called BeeKeeperAI, aimed to provide a zero-trust environment within the platform to protect the privacy of healthcare data and the intellectual property (IP) of the algorithm being developed. The platform would also allow stakeholders to streamline workflows by enabling data access and transformation in a collaborative, secure environment.
Mary Beth Chalk, co-founder and chief commercial officer of BeeKeeperAI, sat down with Healthtech Analytics to discuss how confidential computing can help tackle common clinical algorithm development hurdles and how stakeholders can utilize the approach for real-world applications.
The promise of confidential computing
Chalk emphasized that the current clinical AI development landscape is riddled with challenges, most of which center around privacy, security, time and cost. On their own, each presents a unique problem to solve, but together, they present larger obstacles that prevent effective collaboration and workflow efficiency.
"From a health system perspective, [there are] two sides of the marketplace: the data steward side --which is responsible for data sovereignty and patient privacy -- and the algorithm developer side, which is working on creating healthcare AI," Chalk explained, noting that while these two might exist within the same organization, the workflow challenges make collaboration extremely difficult.
She further noted that prior to its spin-out from UCSF, the BeeKeeperAI team was learning how much the time and effort invested in the algorithm development process could negatively impact a project.
The contracting and approval processes needed to enable AI developers to gain access to sufficient quantities of high-quality, real-world data -- which are necessary to determine whether the algorithm is going to serve its intended purpose in improving clinical outcomes, capacity or productivity -- create a significant burden for healthcare and life sciences organizations.
"[BeeKeeperAI] began to see that time lag: it was taking a total of nine months, 12 months or 18 months to get the necessary approvals in place for what was, essentially, a two-month computing project," Chalk stated. "So, one can imagine the legal cost and the nonproductive use of scientific brain power just to do the sheer administrative chore of getting the necessary and important approvals."
She underscored that this approach isn't scalable, as time-to-market and obsolescence for software moves significantly more quickly than for medical devices or pharmacotherapy interventions.
Chalk noted that confidential computing can address some of these challenges by helping clinical algorithm developers "move at the speed of software."
"You can protect data at rest. You can protect intellectual property at rest. You can protect IP and data in transit, but the last frontier that confidential computing addresses is encrypted protection of both during [computing]," she explained.
Chalk further indicated that enabling computation within a confidential computing environment helps provide end-to-end encrypted protection. The full end-to-end encryption that confidential computing can offer for data and IP across the development process is key for secure, efficient clinical algorithm development.
Chalk emphasized that other approaches, like federated learning, encrypt data at rest and in transit, but during the computing phase, the data and the algorithm's IP are exposed, creating privacy and security concerns. She underscored that much of the contracting and approval process for algorithm development is centered on this potential for data and IP exposure.
"Confidential computing becomes an enabling core competency that allows you to assure that data sovereignty is protected, individual privacy is protected, and intellectual property remains protected at all times," she noted.
The confidential computing environment
A confidential computing approach can enable these protections and provide a streamlined, secure environment for multiparty clinical AI development.
Chalk likened healthcare AI research development to a "well-worn goat path," in which multiple parties, like an algorithm developer and a data steward, know the steps needed to complete a project.
"[The process is] pretty much a standard template, so if you use the Pareto principle, 80% of it is the same thing every single time," she stated. However, collaborating on these steps presents a challenge, as completing each step requires significant manual efforts on behalf of the stakeholders.
A confidential computing environment can alleviate this by providing a platform to help facilitate each step of the process without requiring significant manual input.
Chalk explained that for BeeKeeperAI, the solution's user interface helps achieve this. An algorithm developer can use the interface to upload the project protocol, which is transferred to the data steward's interface to provide details about the model, its clinical importance and its data specifications.
The data steward can then use this information to identify whether they have relevant clinical data for that model and curate that data to the AI developer's specifications.
By streamlining the collaborative process, the confidential computing environment allows users to more readily test the model in sandbox environments and tweak it if needed.
The solution is built on automated workflows, but because these exist in an encrypted environment, stakeholders can take advantage of improved collaboration without sacrificing security.
"So we've taken this well-worn goat path and created an application software layer that allows the users [to collaborate within] a very familiar environment and process," Chalk explained. "Then what we've done is hinged multiple privacy-enhancing technologies underneath that workflow," including checks for nefarious activity and data exfiltration within the project enclave.
Further, the confidential computing enclave is operated within the data steward's environment, meaning that data never leaves the steward's control. Because the solution is software-as-a-service, data stewards do not need significant expertise in confidential computing to take advantage of the platform.
"[BeeKeeperAI maintains the software], we manage it, but we never take possession of the data. We never see the data or the algorithm," Chalk said, emphasizing that the confidential computing environment helps AI developers and data stewards continue doing what they've always done.
By creating an environment in which stakeholders can collaborate securely, confidential computing tools can streamline or eliminate administration-heavy aspects of the clinical AI development process, such as business associate agreements and institutional review board approvals.
In doing so, confidential computing has the potential to spur a host of real-world research and development projects.
Real-world applications
Accelerating medical innovation is a major goal for many healthcare stakeholders, but available approaches are limited.
One major roadblock to innovation is knowing what type of healthcare data to use. Chalk noted that choosing whether to use synthetic, de-identified, or real-world data depends on the type of AI being developed and where stakeholders are in the AI life cycle.
"Within that life cycle -- starting with de novo model training, hypothesis creation, [or] ferreting out signal versus noise from data in those early days -- synthetic data is perfectly appropriate," she explained. "The challenge is for clinical AI: the minute you begin to move into demonstrating its efficacy, its impact on clinical outcomes or its safety within a clinical setting, you have to be operating on real-world data."
The problem for many AI development teams lies in this "data cliff," in which synthetic data might be useful early on, but then models remain in the research and development stage due to issues around accessing appropriate, high-quality, real-world data for model validation.
Chalk further indicated that using real-world data is crucial as researchers look to advance precision medicine.
She explained that, to date, research into disease treatment has been forced to rely on a bell curve paradigm, in which researchers work to find interventions and medicine that work for most patients. Under this approach, these treatments become part of the standard of care.
But precision medicine presents an opportunity to investigate the mechanisms underlying why some patients are on the tails of the bell curve, either reacting better than most to a medical intervention or facing adverse outcomes as a result. A confidential computing approach could help research teams explore precision medicine while keeping the necessary patient data secure.
"With confidential computing, I can go drill into those [bell curve] tails and see what was unique about those patients without exposing who those patients were and violating their privacy," Chalk stated.
Currently, applications of confidential computing in healthcare might not be receiving the same hype as technologies like AI, but Chalk indicated that many healthcare and life sciences organizations are exploring a handful of use cases.
"We're seeing a couple of use cases, but the broader theme is around improving the precision of detecting disease [and] predicting a disease trajectory for an individual patient," she stated, underscoring that doing so can provide valuable treatment insights and clinical decision support. "It's that broader theme of precision -- being able to predict with high assurance that a particular treatment protocol for one patient will be amazingly successful [or] lifesaving while it could kill another unintentionally."
The knowledge gathered from these efforts has the potential to significantly inform future treatment approaches or to repurpose existing orphaned pharmacotherapy interventions.
Chalk emphasized that many of these efforts are taking place in the realms of neurology, mental health, oncology and rare disease -- fields that often require the use of real-world genomic data from relatively small patient populations.
"There's a very high risk of re-identification, whether the data is synthetic or de-identified. So, those are the areas that we're seeing the initial opportunity of delivering the greatest value with confidential computing," she added.
Shania Kennedy has been covering news related to health IT and analytics since 2022.