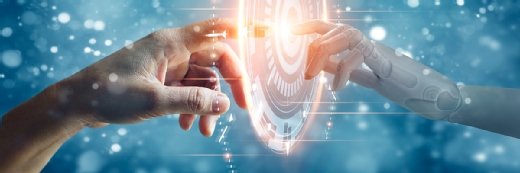
Getty Images
Exploring generative artificial intelligence in healthcare
As the hype around generative AI continues, healthcare stakeholders must balance the technology’s promise and pitfalls.
Artificial intelligence is set to transform healthcare, bolstering both administrative and clinical workflows across the care continuum. As these technologies have rapidly advanced over the years, the pros and cons of AI use have become more apparent, leading to mixed perceptions of the tools among providers and patients.
Despite this, many health systems are increasingly prioritizing AI initiatives, with experts predicting that generative AI will continue to make a splash in healthcare. A recent survey commissioned by Wolters Kluwer Health found that physicians are cautiously optimistic about generative AI, while a report from John Snow Labs revealed that healthcare and life sciences organizations are increasingly investing in the tools.
In this primer, Healthtech Analytics will outline the basics of generative AI, its potential use cases and its pitfalls in healthcare.
WHAT IS GENERATIVE AI?
Harvard conceptualizes generative AI as “a type of artificial intelligence that can learn from and mimic large amounts of data to create content such as text, images, music, videos, code, and more, based on inputs or prompts.”
These prompts come in the form of machine-readable inputs, such as text, images or videos. Through extensive training on large datasets, generative AI tools use these inputs to create new content. The algorithms rely on other AI approaches, such as machine learning, deep learning and natural language processing (NLP), to perform these tasks.
The history of generative AI spans decades, beginning with the development of ELIZA, the first chatbot, by MIT researchers in 1966. Basic chatbots of this nature continued to evolve well into the 2000s, but more advanced conversational agents would not be developed until 2010, when IBM’s Watson competed on the game show Jeopardy! In 2021, more familiar generative AI chatbots came onto the scene.
Today, there are various types of generative AI: large language models (LLMs), recurrent neural networks, transformer models, foundation models, autoregressive models, generative adversarial networks and variational autoencoders.
Generative AI captured public attention in November 2022 with the release of OpenAI’s ChatGPT, and since then, the tools have been increasingly deployed across industries. Healthcare is no exception, as stakeholders continue investigating how AI could make the sector more efficient.
THE PROMISE OF GENERATIVE AI IN HEALTHCARE
Because generative AI is trained on vast amounts of data to generate realistic, high-quality outputs in various mediums, its potential is significant. To date, researchers and healthcare organizations have investigated a plethora of use cases for the technology in administrative and clinical settings.
Some of the most promising applications for generative AI are related to electronic health records (EHRs) and workflow optimization. EHR vendors are utilizing the technology to summarize patient information, speed up patient portal messaging, generate hospital discharge summaries and streamline clinical documentation.
Generative AI tools are also creating a buzz in revenue cycle management and health insurance.
On the clinical side, researchers are exploring how the technology could support improved care and patient engagement.
In April, a research team from Florida State University was awarded a $1 million grant from the US Department of Health and Human Services (HHS) Agency for Healthcare Research and Quality (AHRQ) to create a generative AI tool capable of helping older patients better understand their lab results.
Researchers writing recently in the Journal of Medical Systems demonstrated that ChatGPT may enhance geriatric polypharmacy management and deprescription by providing clinical decision support to primary care physicians.
Generative AI-based chatbots of various types have been deployed in virtual care, including for applications in patient triage, online symptom checking, patient education and mental healthcare.
In a recent Healthcare Strategies episode, leadership from Stanford Medicine Children’s Health detailed how investigators explore using an LLM to prevent data disclosures for adolescent patients and protect pediatric health information.
Despite the significant promise of generative AI, there are a number of roadblocks preventing its widespread adoption.
BARRIERS TO ADOPTION
Like any technology, generative AI presents multiple potential pitfalls alongside its possibilities.
Issues like bias and trust are at the forefront of conversations about how to safely implement AI in healthcare, and these issues are particularly relevant for tools as complex as generative AI.
In the January 2024 issue of The Lancet Digital Health, researchers determined that GPT-4 exhibited gender and racial bias in multiple clinical tasks, such as differential diagnostic reasoning and medical plan recommendations.
Research into tackling these biases is ongoing, but concerns about potential model bias are part of a much larger debate around the use of black box AI in healthcare.
Critics allege that black box tools — in which the decision-making process is hidden or inscrutable — cannot be easily assessed for problems like bias or model drift. Some argue that the inability to determine how these models generate their outputs could also erode patient and provider trust.
To address this, many have called for a focus on “explainable” AI, which could shed light on an algorithm’s accuracy, transparency and fairness.
However, other stakeholders assert that explainability cannot effectively solve the black box AI problem, while some posit that black box models present less of an issue in lower-stakes applications, such as administration, and therefore should not be entirely avoided in healthcare.
Many underscore that as AI becomes more complex, black box models may become unavoidable in spite of explainability efforts. An advanced algorithm capable of processing vast amounts of data will remain inscrutable to humans due to its complexity.
Alongside these issues, a March 2024 study in the Journal of Medical Internet Research revealed that generative AI poses major security and privacy risks that could threaten patients’ protected health information. Particularly, the authors emphasized that algorithmic bias, system vulnerability and clinical integration challenges were some of the most significant hurdles to successful generative AI deployment in medical settings.
To address some of the concerns around the use of AI in healthcare, experts from the Brookings Institution recommend that stakeholders focus on transparency, informed consent protections and breaking up data monopolies via health information exchanges (HIEs).
Healthcare organizations and other groups have also drafted guidelines to help providers and payers navigate these challenges. Recently, the National Academy of Medicine released its AI Code of Conduct, which brought together researchers, patient advocates and others to outline the national architecture needed to promote the responsible, equitable use of these technologies in healthcare.
Despite the significant challenges associated with generative AI, the technology's allure is unlikely to fade anytime soon. By understanding these tools, including their pros and cons, healthcare stakeholders can more effectively navigate the ever-changing generative AI landscape.