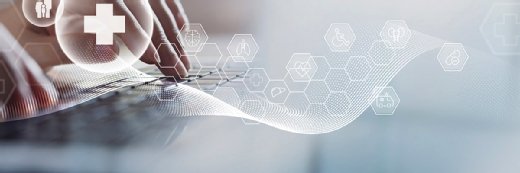
tippapatt - stock.adobe.com
Exploring ACR's AI quality assurance program for radiology
The American College of Radiology's national quality assurance program seeks to help providers safely and effectively implement medical imaging AI.
Medical imaging is one of the most promising use cases for AI, with the potential to significantly advance disease monitoring and diagnostics. To date, most of the over 900 AI- and machine learning-enabled medical devices authorized by the FDA are for use in radiology.
However, many hurdles prevent widespread deployment of these technologies in healthcare -- including AI governance.
Governance is critical for ensuring that AI models are safe and effective, but building AI governance infrastructure from the ground up remains unattainable for many healthcare organizations. To close this gap, industry stakeholders are working to create governance frameworks that providers can use.
The American College of Radiology (ACR) recently launched the ACR Recognized Center for Healthcare-AI (ARCH-AI), the first national AI quality assurance program for radiology providers.
Christoph Wald, M.D., Ph.D, MBA, FACR, vice chair of the ACR Board of Chancellors and chair of the ACR Commission on Informatics, detailed the challenges ARCH-AI is set to address and how the framework can help providers navigate future innovations in medical AI.
The challenges of integrating AI in radiology
A key aspect of ARCH-AI involves helping healthcare organizations address obstacles to AI integration in radiology. These obstacles are related to legacy infrastructure, functionality limitations and information exchange.
Conventional infrastructure
Wald emphasized that conventional infrastructure and solutions deployed in radiology departments, such as radiology information systems (RIS), picture archiving and communication systems (PACS), voice recognition and other systems, were created when AI tools were not yet widely available.
"Many of these systems were not designed to natively or easily integrate with the AI systems that provide specific outputs," he explained. "For example, it is not easy to make AI results visible in a traditional PACS system without first archiving the result in PACS together with the actual patient data."
"While this is a workaround to make a result visible on the PACS, this is not advisable," he continued. "Especially not when the result comes from a nondiagnostic, triage-only cleared AI algorithm designed and tested solely to prioritize workflow based on the presence of a certain imaging observation -- which do not equal a diagnosis." He further noted that many imaging practices have devised these workarounds and custom integration solutions in an effort to efficiently incorporate AI results into routine workflows.
"Those custom solutions for interaction with AI output are built around legacy technical obstacles but are not necessarily optimized -- yet -- for usability and efficiency, and [they] are not necessarily available to smaller practices or those without designated IT teams or development resources," Wald stated.
To combat this, many AI platform vendors are exploring how to tackle these shortcomings.
"We hope to see near-term product innovation of radiology production systems to incorporate results more easily," he said. "There are some clear signs that newer generative AI-based reporting systems and more modern architecture production systems and workflow orchestration solutions are now increasingly being built AI-ready to receive and facilitate interaction with results coming from AI sources."
Limited functionality
Another challenge revolves around the limited functionality of current AI solutions. Wald indicated that many AI systems currently available in the U.S. are designed with limited functionality and cleared for relatively narrow use cases.
He underscored that this is expected to change over time, as it has in other parts of the world with more robust regulatory frameworks for AI.
Wald also highlighted that AI is not "push-button technology."
"AI technology is different from other FDA-cleared devices we use in radiology departments. FDA clearance does not guarantee that the technology works as demonstrated in pre-market performance testing," he explained. "Performance very much depends on similarity between training data and input imaging data -- on which the technology runs -- used locally."
Therefore, local users must verify that an AI tool works as intended and make sure that performance does not degrade over time as radiology solutions and protocols continue to evolve.
Information exchange
Alongside these challenges, information exchange remains an issue. Wald noted that assessing whether an AI is able to correctly classify imaging observations depends on factors outside of the imaging data or the prevalence of the condition in question.
"It also depends on clinical information and a reasonable radiologist-generated 'ground truth' where applicable, for training and/or performance assessment," he said. "Comparing AI output, radiologist output and medical information requires a degree of 'semantic interoperability' of the data."
This semantic interoperability serves to establish a shared understanding that helps determine which diagnosis or condition is represented within the data and the AI system.
"The ability of disparate systems to unambiguously match disease, anatomic, and/or location concepts across these diverse medical data sources, and to exchange individual patient-relevant content seamlessly, remains perhaps the biggest challenge today," Wald stated. "Approaches like the Open Imaging Data Model try to address some of these historically developed shortcomings."
AI economics
The final challenge relates to what Wald calls "AI economics."
"Specific practice context determines whether the value proposition and return on investment for specific AI solutions are worth the expense," he said, indicating that tools like triage AI might be particularly useful for a busy emergency radiology practice, but may not be a significant value-add for a daytime outpatient imaging enterprise.
"Furthermore, the economics of AI implementation remain challenging as there is no consistent payment model and licensing fees, and implementation and maintenance costs can be substantial."
The role of ARCH-AI in AI governance
AI governance can help tackle many of these challenges, and Wald emphasized that ARCH-AI's governance approach is designed to provide a way of thinking about full AI lifecycle implementation at a local level.
"There are a number of operational and workflow considerations for incorporating AI into a local imaging practice," he explained. "There are use case-specific nuances that need to be worked out by the local users; for instance, which imaging studies are being routed to which AI algorithm, creating the logic behind and connections needed to determine how certain AI results affect prioritization of pending cases, or how the actual AI results are to be incorporated into radiology reports -- if at all -- at the local site."
He further noted that these considerations will depend on local standards of care, preferences of the imaging experts and the needs of the referring physicians, who will receive image interpretation results from radiologists utilizing AI.
"ARCH-AI addresses all of these aspects and gives programmatic guidance to imaging departments around which aspects of the AI ecosystem to address while giving leeway to the local experts to work out the details of the implementation," he said.
This contributes to national standardization efforts around the way radiology practices approach AI implementation, allowing them to reap the benefits of the technology while maximizing patient safety and improving health outcomes.
"The program also serves as a bidirectional vehicle for communication between ACR as an independent convener and organizations involved in the formulation of practice parameters," Wald added.
The ACR Data Science Institute (DSI) intends to use results and feedback from participating sites to guide the evolution of the ARCH-AI and Assess-AI frameworks, alongside informing future practice parameters on the use of AI in medical imaging.
To that end, Wald stated that ARCH-AI is a living framework that provides a platform for dialogue among current users of the technology to share best practices and inspire others to follow suit.
"AI holds tremendous promise, but it is different from legacy technology [clinicians] are familiar with and, therefore, needs to be done right," he noted.
"We are planning to use the ensuing [dialogue] with net new applicant sites and initial sites to evolve our understanding of what represents good practice in AI implementation in imaging, which is an ever-evolving area of practice as commercial offerings change, new technology arrives on the scene and more experience is collected in real-world implementations. By design, this is an iterative process, and the current manifestation is expected to morph over time."
By participating in the program, ARCH-AI sites can use the framework to assess whether they are currently addressing all building blocks of quality imaging AI implementation, he underscored. For sites that are not, the framework supports gap analysis that stakeholders can use to round out their AI integration programs.
Radiology facilities that are compliant with ARCH-AI's site criteria -- which center around governance, model selection, acceptance testing, local performance monitoring and participation in ACR's AI registry -- can attest to their compliance to become publicly recognized.
The initial two clinical use cases for the registry are detection of intracranial hemorrhage and pulmonary embolism, and it is expected that many more radiology-driven AI use cases will be adopted into the registry following the ARCH-AI pilot period.
Keeping pace with innovation in medical imaging AI
As AI in healthcare continues to advance rapidly, stakeholders are faced with questions of how to keep up. The use of AI is currently limited to those healthcare organizations with the technical and economic capability to support workflow integration, but frameworks like ARCH-AI could help.
"The ARCH-AI framework is all-encompassing and does not discriminate by type of practice, geography or type of patient. At the same time, major components of the framework, specifically the Assess-AI registry, are made available to ACR members and nonmembers at very low cost, which levels access to this important technology across practices with more or less financial means, essentially economically democratizing access to the program and its specific functionality," Wald explained.
While ARCH-AI cannot directly influence access to healthcare AI technology, the framework can help improve access to the quality assurance practices and tools necessary to manage the use of AI locally.
"Over time, we expect that the network effect from many sites joining Assess-AI might lead to a future state in which developers would have an easier time [accessing] more diverse training data from a more diverse network of practices than is currently technically and economically feasible," he continued.
ACR's existing connections with thousands of radiology practices to establish accreditation programs and data registries could present a foundation to build equitable access to AI governance and development activities over time.
The hope is that these activities will eventually benefit underrepresented patient groups, Wald highlighted. Such efforts could also bolster health equity initiatives.
"Aggregation and comparative analysis of performance data for AI -- coming from diverse practices and geographies -- also facilitates recognition of potential inbuilt biases if inferior performance of models is noted in certain populations or locales," he said. "This could help identify scenarios where AI needs to be adapted to better function in certain underserved areas or populations which may not have been captured by traditional AI development and training methods."
In this way, ARCH-AI and the Assess-AI registry could provide the basis for an early warning system to alert stakeholders of the need to better adapt their AI tools through further training using data from local populations or subtypes of populations that might not have been well-represented in the original dataset.
While ARCH-AI provides a robust framework for AI governance, Wald indicated that the ACR DSI team expects to update it as feedback and learnings from its real-world implementation come in.
"Performance assessment of AI is in and of itself a complex undertaking. Not least since for some AI results, there exists a human-generated 'ground truth,' whereas for other types of AI it is hard to immediately establish if the output of the algorithm is 'correct' or 'accurate,'" he stated.
"This will require careful development over time of optimal and new ways in which to best perform real-world performance monitoring. So, from a technical and a process standpoint, it is still early days, and end-users as well as experts are constantly evolving their understanding of the technology and its application to imaging practice."
He further underscored that there are many technical and scientific challenges in terms of assessing AI performance. One of these is defining what 'high performance' looks like. When assessing a tool, users must compare the expected and observed outputs.
The ability to do so is largely dependent on a host of factors, including the prevalence of the target condition in the population in which the AI technology is used.
"As such, the initial implementation -- especially of the registry Assess-AI -- will reveal the many opportunities for improved interfacing for use-case specific best practices in determining performance," Wald noted. "This version of the AI registry certainly has limitations, but begins to establish some of the principal data pipes and infrastructure needed to eventually achieve a more comprehensive post-market surveillance program."
As AI becomes more prevalent in radiology and healthcare more broadly, Wald emphasized the need for a measured approach to the technology.
"Here is our call to action for our colleagues in the medical imaging community: Be curious, interface with the technology where it makes sense in your own practice, get educated about the benefits and limitations of AI, [and] learn about the basic building blocks of implementation and management of the use of AI in your practice to be ready for a future where this technology may play an increasing role in how imaging care is delivered," he said.
"Keep an open mind toward future changes. We have not yet figured it all out. Become an active participant in shaping how we use and monitor [AI]. Enter into the dialogue with practices and vendors -- especially on which technology and use cases are and are not useful to actual practice."
Shania Kennedy has been covering news related to health IT and analytics since 2022.