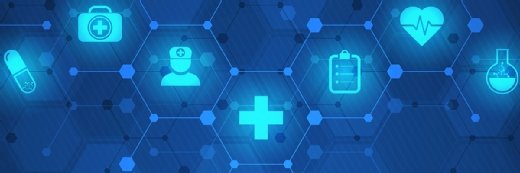
bestbrk/istock via Getty Images
Exploring 3 types of healthcare natural language processing
Natural language processing, understanding and generation might help healthcare stakeholders make better use of their wealth of unstructured, text-based data.
Healthcare generates massive amounts of data as patients move along their care journeys, often in the form of notes written by clinicians and stored in EHRs. This data is valuable to improve health outcomes, but is often difficult to access and analyze.
Natural language processing (NLP) technologies provide a potential solution, as these tools can help care teams and researchers sift through mountains of data and generate meaningful insights for applications in population health management and clinical decision support.
The concept of NLP use in healthcare has been the subject of much hype as clinician burnout and frustrations with existing EHR systems plague the industry, but the two major components of NLP -- natural language understanding (NLU) and natural language generation (NLG) have garnered less attention.
This primer will take a deep dive into NLP, NLU and NLG, differentiating between them and exploring their healthcare applications.
Differentiating NLP, NLU and NLG
NLP, NLU and NLG are related, but distinct concepts. Broadly, NLU and NLG are subsets of NLP.
NLP utilizes methods taken from computer and data science, language modeling, linguistics and AI to help computers understand verbal and written forms of human language. Using machine learning (ML) and deep learning techniques, NLP converts unstructured language data into a structured format via named entity recognition (NER).
NER is a type of information extraction that allows named entities within text to be classified into predefined categories, such as people, organizations, locations, quantities, percentages, times and monetary values.
Through NER and the identification of word patterns, NLP can be used for tasks like answering questions or language translation.
As a component of NLP, NLU focuses on determining the meaning of a sentence or piece of text. NLU tools analyze syntax -- the grammatical structure of a sentence -- and semantics -- the intended meaning of the sentence. NLU approaches also establish an ontology, or structure specifying the relationships between words and phrases, for the text data on which they are trained.
Syntax, semantics and ontologies are all naturally occurring in human speech, but analyses of each must be performed using NLU for a computer or algorithm to accurately capture the nuances of human language.
NLU is often used in sentiment analysis by brands looking to understand consumer attitudes, as the approach allows companies to more easily monitor customer feedback and address problems by clustering positive and negative reviews.
Healthcare applications for NLU often focus on research, as the approach can be used for data mining within patient records. In 2022, UPMC launched a partnership to help determine whether sentinel lymph node biopsy is appropriate for certain breast cancer cohorts by using NLU to comb through unstructured and structured EHR data.
While NLU is concerned with computer reading comprehension, NLG focuses on enabling computers to write human-like text responses based on data inputs.
NLG tools typically analyze text using NLP and considerations from the rules of the output language, such as syntax, semantics, lexicons and morphology. These considerations enable NLG technology to choose how to appropriately phrase each response.
NLG is used in text-to-speech applications, driving generative AI (GenAI) tools like ChatGPT and Gemini to create human-like responses to a host of user queries.
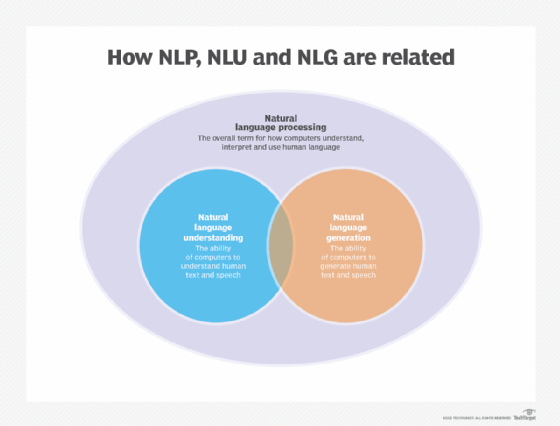
Healthcare use cases
The potential benefits of NLP technologies in healthcare are wide-ranging, including their use in applications to improve care, support disease diagnosis and bolster clinical research.
One of the most promising use cases for these tools is sorting through and making sense of unstructured EHR data, a capability relevant across a plethora of applications.
Using data extracted from EHRs, NLP approaches can help surface insights into vascular conditions, maternal morbidity and bipolar disorder.
On the administrative side, NLP is also valuable for medical coding and patient safety event reports. The methodology has also demonstrated promise in streamlining patient feedback analysis.
Currently, a handful of health systems are using NLP tools. NorthShore -- Edward-Elmhurst Health deployed the technology within its emergency departments to tackle social determinants of health, and Mount Sinai has incorporated NLP into its web-based symptom checker.
NLU has been less widely used, but researchers are investigating its potential use cases, particularly those related to chatbots for healthcare communication.
In particular, research published in Multimedia Tools and Applications in 2022 outlines a framework that relies on ML, NLU and statistical analysis to facilitate the development of a chatbot for patients to find useful medical information.
Like NLP more broadly, NLG has significant potential for use in healthcare-driven GenAI applications, such as clinical documentation and revenue cycle management.
Barriers to adoption
Despite the promise of NLP, NLU and NLG in healthcare, these technologies have limitations that hinder deployment.
Many of these are shared across NLP types and applications, stemming from concerns about data, bias and tool performance.
Researchers writing in the Canada Communicable Disease Report noted that NLP shares one major limitation with AI, ML and other advanced analytics technologies: data access and quality. The availability of appropriate and high-quality data is key to training NLP tools, and while accessible biomedical data sets exist, they can be limited by data type or research area.
The authors further indicated that failing to account for biases in the development and deployment of an NLP model can negatively impact model outputs and perpetuate health disparities. Privacy is also a concern, as regulations dictating data use and privacy protections for these technologies have yet to be established.
The researchers noted that, like any advanced technology, there must be frameworks and guidelines in place to make sure that NLP tools are working as intended. However, these frameworks and guidelines also have yet to be developed.
In addition to these challenges, one study from the Journal of Biomedical Informatics stated that discrepancies between the objectives of NLP and clinical research studies present another hurdle.
NLP tools are developed and evaluated on word-, sentence- or document-level annotations that model specific attributes, whereas clinical research studies operate on a patient or population level, the authors noted. While not insurmountable, these differences make defining appropriate evaluation methods for NLP-driven medical research a major challenge.
NLP technologies of all types are further limited in healthcare applications when they fail to perform at an acceptable level.
Technologies and devices utilized in healthcare are expected to meet or exceed stringent standards to ensure they are both effective and safe. Like other AI technologies, NLP tools must be rigorously tested to ensure that they can meet these standards or compete with a human performing the same task.
Despite these limitations to NLP applications in healthcare, their potential will likely drive significant research into addressing their shortcomings and effectively deploying them in clinical settings.
Shania Kennedy has been covering news related to health IT and analytics since 2022.