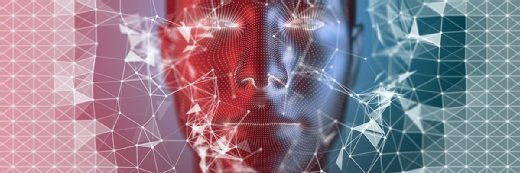
Getty Images
Arguing the Pros and Cons of Artificial Intelligence in Healthcare
Artificial intelligence in healthcare is a hot topic, sparking ongoing debate about the ethical, clinical, and human impacts the tech can have on patient care.
In what seems like the blink of an eye, mentions of artificial intelligence (AI) have become ubiquitous in the healthcare industry.
From deep learning algorithms that can read computed tomography (CT) scans faster than humans to natural language processing (NLP) that can comb through unstructured data in electronic health records (EHRs), the applications for AI in healthcare seem endless.
But like any technology at the peak of its hype curve, artificial intelligence faces criticism from its skeptics alongside enthusiasm from die-hard evangelists.
Despite its potential to unlock new insights and streamline the way providers and patients interact with healthcare data, AI may bring considerable threats of privacy problems, ethical concerns, and medical errors.
Balancing the risks and rewards of AI in healthcare will require a collaborative effort from technology developers, regulators, end-users, and consumers.
The first step will be addressing the highly divisive discussion points commonly raised when considering the adoption of some of the most complex technologies the healthcare world has to offer.
AI WILL CHALLENGE THE STATUS QUO IN HEALTHCARE
AI in healthcare will challenge the status quo as the industry adapts to new technologies. As a result, patient-provider relationships will be forever changed, and the idea that AI will change the role of human workers to some extent is worth considering.
Seventy-one percent of Americans surveyed by Gallup in 2018 believed that AI will eliminate more healthcare jobs than it creates, with just under a quarter indicating that they believe the healthcare industry will be among the first to see widespread handouts of pink slips due to the rise of machine learning tools.
However, more recent data around occupational shifts and projected job growth don’t necessarily bear this out.
A report published earlier this year by McKinsey & Co. indicates that AI could automate up to 30 percent of the hours worked by US employees by 2030, but healthcare jobs are projected to remain relatively stable, if not grow.
The report notes that health aides and wellness workers will have anywhere from 4 to 20 percent more of their work automated, and health professionals overall can expect up to 18 percent of their work to be automated by 2030.
But healthcare employment demand is expected to grow 30 percent by then, negating the potential harmful impacts of AI on the healthcare workforce.
Despite these promising projections, fears around AI and the workforce may not be entirely unfounded.
AI tools that consistently exceed human performance thresholds are constantly in the headlines, and the pace of innovation is only accelerating.
Radiologists and pathologists may be especially vulnerable, as many of the most impressive breakthroughs are happening around imaging analytics and diagnostics.
In a 2021 report, Stanford University researchers assessed advancements in AI over the last five years to see how perceptions and technologies have changed. Researchers found evidence of growing AI use in robotics, gaming, and finance.
The technologies supporting these breakthrough capabilities are also finding a home in healthcare, and physicians are starting to be concerned that AI is about to evict them from their offices and clinics. However, providers’ perceptions of AI vary, with some cautiously optimistic about its potential.
“Recent years have seen AI-based imaging technologies move from an academic pursuit to commercial projects. Tools now exist for identifying a variety of eye and skin disorders, detecting cancers, and supporting measurements needed for clinical diagnosis,” the report stated.
“Some of these systems rival the diagnostic abilities of expert pathologists and radiologists, and can help alleviate tedious tasks (for example, counting the number of cells dividing in cancer tissue). In other domains, however, the use of automated systems raises significant ethical concerns.”
At the same time, however, one could argue that there simply aren’t enough radiologists and pathologists — or surgeons, or primary care providers, or intensivists — to begin with. The US is facing a dangerous physician shortage, especially in rural regions, and the drought is even worse in developing countries around the world.
AI may also help alleviate the stresses of burnout that drive healthcare workers to resign. The epidemic affects the majority of physicians, not to mention nurses and other care providers, who are likely to cut their hours or take early retirements rather than continue powering through paperwork that leaves them unfulfilled.
Automating some of the routine tasks that take up a physician’s time, such as EHR documentation, administrative reporting, or even triaging CT scans, can free up humans to focus on the complicated challenges of patients with rare or serious conditions.
Most AI experts believe that this blend of human experience and digital augmentation will be the natural settling point for AI in healthcare. Each type of intelligence will bring something to the table, and both will work together to improve the delivery of care.
Some have raised concerns that clinicians may become over-reliant on these technologies as they become more common in healthcare settings, but experts emphasize that this is unlikely to occur, as automation bias isn’t a new topic in healthcare, and there are existing strategies to prevent it.
Patients also appear to believe that AI will improve healthcare in the long run, despite some concerns about the technology’s use.
A research letter published in JAMA Network Open last year that surveyed just under 1,000 respondents found that over half believed that AI would make healthcare either somewhat or much better. However, two-thirds of respondents indicated that being informed if AI played a big role in their diagnosis or treatment was very important to them.
Concerns about the use of AI in healthcare appear to vary somewhat by age, but research conducted by SurveyMonkey and Outbreaks Near Me — a collaboration between epidemiologists from Boston Children's Hospital and Harvard Medical School — shows that generally, patients prefer that important healthcare tasks, such as prescribing pain medication or diagnosing a rash, be led by a medical professional rather than an AI tool.
But whether patients and providers are comfortable with the technology or not, AI is advancing in healthcare. Many health systems are already deploying the tools across a plethora of use cases.
Michigan Medicine leveraged ambient computing – a type of AI designed to create an environment that is responsive to human behaviors – to further its clinical documentation improvement efforts in the midst of the COVID-19 pandemic.
Researchers from Mayo Clinic are taking a different AI approach: they aim to use the tech to improve organ transplant outcomes. Currently, these efforts are focused on developing AI tools that can prevent the need for a transplant, improve donor matching, increase the number of usable organs, prevent organ rejection, and bolster post-transplant care.
AI and other data analytics tools can also play a key role in population health management. A comprehensive strategy to manage population health requires that health systems utilize a combination of data integration, risk stratification, and predictive analytics tools. Care teams at Parkland Center for Clinical Innovation (PCCI) and Parkland Hospital in Dallas, Texas are leveraging some of these tools as part of their program to address preterm birth disparities.
Despite the potential for AI in healthcare, though, implementing the technology while protecting privacy and security is not easy.
AI PRIVACY AND SECURITY CHALLENGES
AI in healthcare presents a whole new set of challenges around data privacy and security – challenges that are compounded by the fact that most algorithms need access to massive datasets for training and validation.
Shuffling gigabytes of data between disparate systems is uncharted territory for most healthcare organizations, and stakeholders are no longer underestimating the financial and reputational perils of a high-profile data breach.
Most organizations are advised to keep their data assets closely guarded in highly secure, HIPAA-compliant systems. In light of an epidemic of ransomware and knock-out punches from cyberattacks of all kinds, chief information security officers have every right to be reluctant to lower their drawbridges and allow data to move freely into and out of their organizations.
Storing large datasets in a single location makes that repository a very attractive target for hackers. In addition to AI’s position as an enticing target to threat actors, there is a severe need for regulations surrounding AI and how to protect patient data using these technologies.
Experts caution that ensuring healthcare data privacy will require that existing data privacy laws and regulations be updated to include information used in AI and ML systems, as these technologies can re-identify patients if data is not properly de-identified.
However, AI falls into a regulatory gray area, making it difficult to ensure that every user is bound to protect patient privacy and will face consequences for not doing so.
In addition to more traditional cyberattacks and patient privacy concerns, a 2021 study by University of Pittsburgh researchers found that cyberattacks using falsified medical images could fool AI models.
The study shed light on the concept of “adversarial attacks,” in which bad actors aim to alter images or other data points to make AI models draw incorrect conclusions. The researchers began by training a deep learning algorithm to identify cancerous and benign cases with more than 80 percent accuracy.
Then, the researchers developed a “generative adversarial network” (GAN), a computer program that generates false images by misplacing cancerous regions from negative or positive images to confuse the model.
The AI model was fooled by 69.1 percent of the falsified images. Of the 44 positive images made to look negative, the model identified 42 as negative. Of the 319 negative images doctored to look positive, the AI model classified 209 as positive.
These findings show not only how these types of adversarial attacks are possible, but also how they can cause AI models to make a wrong diagnosis, opening up the potential for major patient safety issues.
The researchers emphasized that by understanding how healthcare AI behaves under an adversarial attack, health systems can better understand how to make models safer and more robust.
Patient privacy can also be at risk in health systems that engage in electronic phenotyping via algorithms integrated into EHRs. The process is designed to flag patients with certain clinical characteristics to gain better insights into their health and provide clinical decision support. However, electronic phenotyping can lead to a series of ethical pitfalls around patient privacy, including unintentionally revealing non-disclosed information about a patient.
However, there are ways to protect patient privacy and provide an additional layer of protection to clinical data, like privacy-enhancing technologies (PETs). Algorithmic, architectural, and augmentation PETs can all be leveraged to secure healthcare data.
Security and privacy will always be paramount, but this ongoing shift in perspective as stakeholders get more familiar with the challenges and opportunities of data sharing is vital for allowing AI to flourish in a health IT ecosystem where data is siloed and access to quality information is one of the industry’s biggest obstacles.
ETHICS, RESPONSIBILITY, AND OVERSIGHT
The thorniest issues in the debate about AI are the philosophical ones. In addition to the theoretical quandaries about who gets the ultimate blame for a life-threatening mistake, there are tangible legal and financial consequences when the word “malpractice” enters the equation.
Artificial intelligence algorithms are complex by their very nature. The more advanced the technology gets, the harder it will be for the average human to dissect the decision-making processes of these tools.
Organizations are already struggling with the issue of trust when it comes to heeding recommendations flashing on a computer screen, and providers are caught in the difficult situation of having access to large volumes of data but not feeling confident in the tools that are available to help them parse through it.
While some may assume that AI is completely free of human biases, these algorithms will learn patterns and generate outputs based on the data they were trained on. If these data are biased, then the model will be, too.
There are currently few reliable mechanisms to flag such biases. “Black box” artificial intelligence tools that give little rationale for their decisions only complicate the problem – and make it more difficult to assign responsibility to an individual when something goes awry.
When providers are legally responsible for any negative consequences that could have been identified from data they have in their possession, they need to be certain that the algorithms they use are presenting all of the relevant information in a way that enables optimal decision-making.
However, stakeholders are working to establish guidelines to address algorithmic bias.
In a 2021 report, the Cloud Security Alliance (CSA) suggested that the rule of thumb should be to assume that AI algorithms contain bias and work to identify and mitigate those biases.
“The proliferation of modeling and predictive approaches based on data-driven…techniques has helped to expose various social biases baked into real-world systems, and there is increasing evidence that the general public has concerns about the societal risks of AI,” the report stated.
“Identifying and addressing biases early in the problem formulation process is an important step to improving the process.”
The White House Blueprint for an AI Bill of Rights and the Coalition for Health AI (CHAI)’s ‘Blueprint for Trustworthy AI Implementation Guidance and Assurance for Healthcare’ have also recently provided some guidance for the development and deployment of trustworthy AI, but these can only go so far.
Developers may unknowingly introduce biases to AI algorithms or train the algorithms using incomplete datasets. Regardless of how it happens, users must be aware of the potential biases and work to manage them.
In 2021, the World Health Organization (WHO) released the first global report on the ethics and governance of AI in healthcare. WHO emphasized the potential health disparities that could emerge as a result of AI, particularly because many AI systems are trained on data collected from patients in high-income care settings.
WHO suggested that ethical considerations should be taken into account during the design, development, and deployment of AI technology.
Specifically, WHO recommended that individuals working with AI operate under the following ethical principles:
- Protecting human autonomy
- Promoting human well-being and safety and the public interest
- Ensuring transparency, explainability, and intelligibility
- Fostering responsibility and accountability
- Ensuring inclusiveness and equity
- Promoting AI that is responsive and sustainable
Bias in AI is a significant negative, but one that developers, clinicians, and regulators are actively trying to change.
Ensuring that AI develops ethically, safely, and meaningfully in healthcare will be the responsibility of all stakeholders: providers, patients, payers, developers, and everyone in between.
There are more questions to answer than anyone can even fathom. But unanswered questions are the reason to keep exploring — not to hang back.
The healthcare ecosystem has to start somewhere, and “from scratch” is as good a place as any.
Defining the industry’s approaches to AI is a significant responsibility and a golden opportunity to avoid some of the past mistakes and chart a better path for the future.
It’s an exciting, confusing, frustrating, optimistic time to be in healthcare, and the continuing maturity of artificial intelligence will only add to the mixed emotions of these ongoing debates. There may not be any clear answers to these fundamental challenges at the moment, but humans still have the opportunity to take the reins, make the hard choices, and shape the future of patient care.