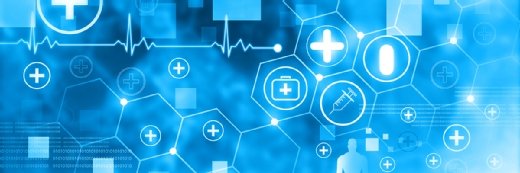
Rasi Bhadramani/istock via Getty
Applying Artificial Intelligence to Chronic Disease Management
Artificial intelligence tools can help providers navigate the complexities of chronic disease management, leading to more effective, quality treatments.
When it comes to healthcare, it seems Americans spend more to receive less.
An aging population, expensive pharmaceuticals, and administrative waste result in sky-high medical costs, while health outcomes remain poorer than those in other high-income countries.
While many elements influence the country’s rising healthcare spending, the prevalence of chronic disease is perhaps the most significant factor at play.
Chronic diseases are the leading causes of death and disability in the US, as well as the main drivers of the country’s $3.5 trillion in annual health costs.
Conditions like diabetes, cancer, and kidney disease take a massive toll on healthcare spending and patient outcomes, making chronic disease management and prevention top priorities for providers across the nation.
In the midst of a global health crisis, these efforts have become even more consequential. Evidence has shown that underlying chronic diseases can lead to poorer outcomes in people infected with COVID-19, putting further strain on the healthcare system.
However, managing and preventing chronic conditions in patient populations is a time-consuming, challenging task. The development and treatment of chronic diseases typically involves multiple features – features that differ from patient to patient.
Genetics, lifestyle choices, and environmental factors can all contribute to the onset of chronic disease, as well as a patient’s or provider’s ability to properly manage these conditions.
With all of this data to consider, chronic disease management is a task perfectly suited for innovative technologies like artificial intelligence.
Organizations are increasingly leveraging AI to improve chronic disease management, drive down costs, and enhance patient health – eventually resulting in more personalized, data-driven care.
Gathering data to uncover new insights
In order to effectively manage or prevent chronic conditions, providers have to engage patients in care coordination and outreach as soon as possible. However, identifying patients at high risk of disease development is incredibly complex without the right tools in place.
This issue is exacerbated in conditions like chronic kidney disease (CKD), which can present in individuals without any real warning.
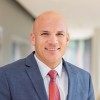
“The majority of dialysis patients first find out that they need dialysis once they end up in the hospital with advanced CKD. Unfortunately, that's not uncommon if you look at national numbers,” Ray Morales, executive operations director of Intermountain Healthcare System Kidney Services, told HealthITAnalytics.
The problem was prevalent at Intermountain, where 70 percent of patients would suddenly find themselves in end-stage renal disease. In 2019, the organization partnered with MDClone to organize and prioritize patients in early stages of CKD, aiming to reduce hospitalizations and boost outcomes.
Leaders implemented analytics algorithms that were able to accurately identify CKD patients by stage and presence of acute kidney injury, as well as stratify these patients by gaps in care and time from last encounter.
A critical reason for the success of these algorithms was the analysis of multiple sources of data, Morales noted.
“Mapping out the patient's journey was really critical to our ability to identify where we could intervene and begin to better manage these patients. When you look at the total cost of care for an individual with advanced chronic kidney disease or end-stage renal disease, about 70 percent of their cost of care is not associated with their kidney disease or dialysis care,” he said.
“Most of the expenses are encompassed in managing their comorbidities – hypertension, diabetes – conditions that drive up the cost of care and the complexity of care. When you look at the patient holistically, there are a lot of opportunities to better manage the patient.”
After examining these data sources, the algorithms were able to proactively identify patients in need of kidney care.
“In our initial query of these patients, we examined some of the claims data and found over 2000 patients that needed to be seen by a nephrologist but hadn't been seen,” said Morales.
“We have a team of kidney care navigators that are actively reaching out to those individuals and working with the primary care physicians. It's about prioritizing the work, and understanding that there's a lot of work that needs to be done in the later stages of CKD.”
Understanding the progressive stages of chronic diseases is key to treating these conditions. This is especially true in the case of Parkinson’s disease, a neurodegenerative disorder in which progression is not easy to define.
“Parkinson's disease is very heterogeneous in that the symptoms may affect both motor and non-motor function. This can make it difficult to assess where a patient may be at a certain point in time,” said Kristen Severson, postdoc researcher at IBM.
To better understand how Parkinson’s disease progresses, IBM Research is collaborating with the Michael J. Fox Foundation to develop a machine learning algorithm using data from patients on medications. As is always the case, the more data obtained to train these kinds of algorithms, the better.
“As we gain access to larger and larger datasets, we could perhaps better understand page to treatment protocols that are effective for certain types of patients. There's a lot of effort around collecting patient data, so I hope that as we have success, these types of studies might become more integrated into patient care,” she said.
“We also might think about how we can better share data across institutions. Particularly if you think that there are subtypes of the disease, you need to see a lot of patients before you can tease out those patterns.”
When training algorithms to generate healthcare insights, it’s not just the amount of data that matters – the transparency of these tools matters as well, said Eugene Santos Jr., PhD, professor of engineering at Dartmouth College.
“The clinical data is the data that is closest to ground truth – it starts at the patient level. Although there can be some problems with the recording of that data, clinical data is provided by direct observation,” he said.
“In contrast, other database information is an aggregation of previous research – generalizations and knowledge that people have extracted. So, a lot of data out there is incomplete, as well as potentially incorrect and conflicted. This is where humans still excel over AI: If you feed an opaque AI system conflicted information, when it gives you an answer you won’t know why that answer came about.”
Researchers at Dartmouth Engineering are working to develop an artificial intelligence system, called the Pathway Hypothesis Knowledgebase (PHK), that analyzes patients’ clinical and genomic data and the relationship between biochemical pathways that drive cancer development.
The goal is to make PHK a transparent AI system so that the reasoning behind the computation is easily explainable to providers.
“The PHK tool takes a lot of different sources of information, like clinical data and biomedical data, and it builds this knowledge base that shows how these sources fit together. That could be cause and effect, it could be correlation, but it explicitly shows how it combines the information,” said Santos.
“Ideally, the dream we have for the PHK tool is that it can start from any level and be able to derive how the human body works.”
Evaluating the near-term impacts of AI on chronic disease
Effectively managing chronic conditions is a long-term, overarching healthcare goal. But with the right analytics tools in place, researchers and provider organizations can meet patients’ immediate and near-term needs.
“Machine learning has the ability to explore a lot more than a human medical researcher can explore. If nothing else, if we treat it at the lowest level as a tool that can say, ‘Okay, let's try this, try this, try this,’ it’s clear that AI offers a more efficient way to explore different solutions,” said Santos.
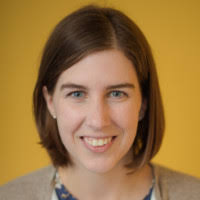
In Santos’s case, the principle aim is to develop a system that can add to providers’ knowledge of cancer.
“The biggest thing is that for us, the PHK tool tries to fill in the missing links. When AI comes in, you can be much more systematic about finding those needles in the haystack. That's what we're shooting for.”
For Severson and her team, the first step is assessing the impact of certain drugs on Parkinson’s disease.
“In this setting, we were particularly interested in modeling medication effects. And I think that is very challenging in and of itself. And this is just an initial piece of work in a long line of work,” said Severson.
“In the case of Parkinson's, it's largely thought that medications don't alter the underlying progression of the disease, but can treat symptoms. With that, we have some ideas to how we might go about modeling the effects.”
Going forward, the machine learning algorithm could provide additional insight into treating other complex disorders, as medication use presents challenges in several chronic diseases where drugs are used to mitigate symptoms.
“We're hoping to do a more thorough investigation of applying this model to Parkinson's disease for additional clinical insights in collaboration with neurologists. And we hope that it could certainly be applied more broadly to chronic diseases, particularly those that don't have medications that alter the course of the disease, such as diabetes or ALS,” said Severson.
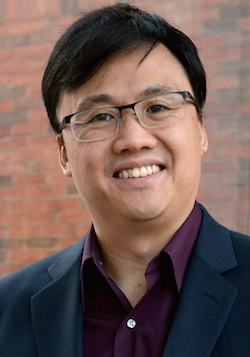
Analytics tools can help organizations make sense of which patient populations to prioritize as well. At Intermountain, these systems led to early intervention and an 86 percent avoidance in unplanned admissions.
“Having all of the information pulled into a data lake, where we could then look at these patients longitudinally, really highlighted the main issue for us. We were able to engage the patients at the right time and project the likelihood of those individuals progressing in their disease, allowing us to intervene,” said Morales.
The effort has improved both care and costs, Morales stated.
“Since this program, we've been able to get over 50 percent of our patients on a home therapy. This is phenomenal, because it represents better clinical care and higher satisfaction for the patient, as well as cost savings to our system where we can provide those services at a fraction of the cost,” he said.
“The clinical aspect of this is most important, but what we find is that as we do the right thing clinically for our patients, we're also able to save significant money to the system.”
Morales and his team have plans to start detecting high-risk patients earlier, leading to even more proactive care and better outcomes.
“We need to move further upstream and identify the earlier stage CKD patients so that we can intervene sooner and begin to provide those patients with some of the lighter-touch type of services, like education around managing their comorbidities, medications, and their diet,” Morales said.
“Those types of things are where this program is going and where really our focus needs to be as we look at future work.”
The future of AI and chronic disease treatment
Right now, the use of AI and machine learning in routine clinical care is still a relatively novel notion. While it may take some time for the technology to make the leap from the research arena into the day-to-day world of healthcare, the future of analytics tools in medicine is promising.
The expectation is that AI can move the industry from reactive to proactive care delivery, leading to more individualized treatments.
“Ultimately, we would love to get to personalized healthcare. When a patient comes in, no matter how common or rare the situation they're suffering from, this is where the AI could really help out. If nothing else, the technology could just identify the problem. And then the next step is, how can we come up with treatments? Can we transfer what we've learned from a treatment of one type of disease to another type of disease?” Santos said.
“This is where the AI and machine learning deal with all the complexities of the human body and all the complexities of the information that's out there. It's a symbiotic relationship where the AI and machine learning solve and help deal with a really complex problem, and come back and push for the different directions that human clinicians could explore.”
Severson noted that while the technology is still evolving, it holds significant potential for chronic disease management.
“I don't know of too many studies that are largely leveraging AI. It's a new and growing field, but we're hopeful that it could play more of a role. To me, there's just so many ways it could help. Particularly for complex chronic diseases, the hope is that machine learning could help provide some additional understanding,” she said.
Severson added that she hoped AI could accelerate the development of personalized treatments as well.
“In Parkinson's disease, we think that machine learning could help us define subtypes or stages of the condition. There's some thought that perhaps there's actually different variants of the disease, or maybe we can discover different subtypes of disease. And by forming those subpopulations, we could do better patient management, as well as more targeted therapeutic discovery,” she stated.
Although there is no denying the detrimental impact of chronic diseases on the healthcare system and individuals across the country, the hope is that analytics tools could help providers get ahead of poor patient outcomes.
At Intermountain, leaders are expanding the CKD program to other clinical programs in the form of comorbidity management.
“We're excited about the program and what we've been able to do. As we look forward, we're actually beginning to engage a number of our other clinical programs and clinical services within Intermountain Healthcare, including our cardiology clinical program and our endocrinology group in this work,” said Morales.
“As we begin to identify more opportunities to smooth out the transitions of care and the clinical care pathways that these patients are taking, and to ensure that it's comprehensive and inclusive of all of these other conditions that exist within these patients, we’re optimistic. We're excited about that the future work ahead of us.”