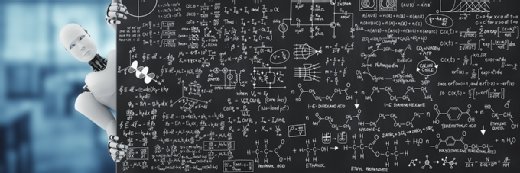
Blue Planet Studio - stock.adobe
Advancing transparency, fairness in AI to boost health equity
Fairness in clinical algorithms is key to mitigating race-based health inequity. Are efforts driven by AI and machine learning up to the task?
The use of race in clinical algorithms has increasingly come under fire as healthcare organizations have begun to pursue health equity initiatives and push back against the practice of race-based medicine. While the recognition that race is a social construct, rather than a biological one, is not new, the move toward race-conscious medicine has gained traction in recent years.
At the same time, evidence pointing to the real and potential harms of race-based algorithms has created significant concerns about how these tools -- many of which are currently in use -- will widen existing health disparities and perpetuate harm.
These worries are exacerbated by the rising use of AI and machine learning (ML), as these technologies are often black box models that remain inscrutable to human users despite the potential for bias.
At the recent "Together to Catalyze Change for Racial Equity in Clinical Algorithms" event -- hosted by the Doris Duke Foundation, the Council of Medical Specialty Societies and the National Academy of Medicine -- healthcare leaders came together to discuss how the industry can embrace the shift away from the use of race as a biological construct in clinical algorithms.
A selection of featured panelists gathered to detail race's use in clinical algorithms to date, with an eye toward addressing its harmful use and advancing health equity. To that end, multiple speakers presented ongoing work to mitigate potential harms from AI and ML tools by prioritizing transparency and fairness.
Building transparency into clinical algorithms
The pursuit of health equity has led many to question the transparency and fairness strategies needed to ensure that clinical algorithms reduce, rather than promote, disparities.
Rapid advances in AI technology have made these considerations critical across the industry, with public and private stakeholders rushing to catch up, as evidenced by guiding principles for ML-enabled devices recently issued by FDA Center for Devices and Radiological Health (CDRH).
"The FDA put out a call for transparency for machine learning-enabled medical devices," explained Tina Hernandez-Boussard, MD, Ph.D., MPH, associate dean of research and associate professor of biomedical informatics at Stanford University. "They're looking at the who, the why, the what, the where and the how for machine learning practices, so when we talk about transparency: transparency for who? Why do we need it to be transparent? What needs to be transparent?"
Much of this work, she indicated, is centered on how transparency can be embedded into clinical algorithms via automated methods to produce information on a tool's training data, the metrics used to validate it and the population to which it is designed to be applied.
However, Hernandez-Boussard emphasized that integrating transparency in this way requires the development of rigorous standards.
"We need standards and tools for transparency because when I say transparency, my definition might be completely different from somebody else's," she noted. "Industry has a different definition of transparency than other entities. So, we need to think about standards and tools for systematically generating this [transparency] information."
She also underscored the need for distributed accountability in order to drive responsible data and model use. Under such a framework, model developers would be responsible for reporting information about the tools they are building, while model implementers would be responsible for determining how to set up continuous monitoring for their clinical AI.
Further, Hernandez-Boussard indicated that assessing the role of patient outcomes in this accountability framework is essential. She also pointed out a need to require participation in the framework to systematically ensure that algorithms are transparent.
She explained that the recently issued final rule under Section 1557 of the Affordable Care Act (ACA) -- which "prohibits discrimination on the basis of race, color, national origin, age, disability, or sex (including pregnancy, sexual orientation, gender identity, and sex characteristics), in covered health programs or activities," per the U.S. Department of Health and Human Services (HHS) -- is key to these efforts, as its mandates require covered entities to identify and mitigate discrimination related to the use of AI or clinical decision support algorithms.
Hernandez-Boussard highlighted that the ongoing efforts to promote transparency and tackle discrimination are crucial for not only creating accountability but also spreading it across multiple stakeholders rather than just model developers.
"Broad scoping rules on discrimination set the stage for where we're going and how we think about these clinical decision support tools, how we need to evaluate them and how we think about deploying them across populations," she stated. "We need to be promoting health."
Sharing the responsibility of AI transparency also creates an environment in which industry stakeholders can collaborate, instead of compete, to advance the use of equitable clinical tools.
Building consensus on responsible health AI
Currently, experts pursuing transparency and accountability efforts for clinical algorithms are challenged by a lack of consensus around what responsible AI looks like in healthcare.
The Coalition for Health AI (CHAI) is working to develop this consensus by bringing together roughly 2,500 clinical and nonclinical member organizations from across the industry, according to its president and CEO, Brian Anderson, MD.
"There's a lot of good work being done behind closed doors in individual organizations [to develop] responsible AI best practices and processes, but not at a consensus level across organizations," Anderson stated. "In a consequential space like healthcare, where people's lives are on the line… that's a real problem."
He explained that the health systems that founded CHAI saw this as an opportunity to bring collaborators from every corner of the industry to develop a definition for responsible healthcare AI. However, willingness to collaborate on a responsible AI framework does not mean that defining concepts like fairness, bias and transparency are straightforward.
While there is agreement on metrics like area under the curve, for example, it's not easy to come to full consensus. This is because the stakes are high, Anderson said. Not only do providers, payers and model developers need to come together, he said, but patients' perspectives must also be part of the conversation, adding another layer of complexity.
As part of these consensus-building efforts, CHAI is homing in on a technical framework to help inform developers about what responsible AI looks like throughout the development, deployment, maintenance and monitoring steps of a model's life cycle.
Alongside these technical standards, the coalition is pursuing a national network of AI assurance labs. These labs would serve to bridge the gap between the development of clinical AI evaluation metrics and the application of such metrics to assess current and future tools, Anderson noted. The results of these evaluations would then be added to a national registry that anyone could use to gauge the fairness and performance of a clinical AI tool.
"I am a Native American, I live in the Boston area, I go to [Massachusetts General Hospital (MGH)], and I want to be able to go to this registry and look at the models that are deployed at MGH and see how they perform on Native Americans," Anderson said. "I want to be empowered to have a conversation with my provider and say, 'Maybe you shouldn't use that model because look at its AUC score on people like me.' That's what we're trying to enable with this kind of transparency."
He indicated that being able to engage with such a national registry could help overcome the lack of education for both healthcare stakeholders and the public around the industry's use of AI.
When asked how a patient could take advantage of CHAI's registry without being aware of what specific models were being applied to them by their healthcare provider, Anderson explained that part of CHAI's work to build its assurance labs involves requiring that each model's entry in the national registry lists the health systems at which the tool is deployed.
CHAI recently sought public feedback on a draft framework presenting assurance standards to evaluate AI tools across the lifecycle in the wake of Congressional criticism regarding the coalition's relationship with the FDA.
These efforts might be further hampered by additional challenges posed by efforts to measure AI fairness.
The challenges of defining and measuring AI fairness
Despite the rapid development of AI and work to build consensus around fairness in algorithms, Shyam Visweswaran, MD, Ph.D., vice chair of clinical informatics and director of the Center for Clinical Artificial Intelligence at the University of Pittsburgh, warned that it might be premature to focus on AI tools -- many of which won't be ready for clinical use for some time -- rather than existing statistical algorithms used for clinical decision-making.
He asserted that performance metrics must be developed for both current statistical algorithms and future AI tools, particularly those that utilize race variables in their calculations. Visweswaran stated that efforts like CHAI's move the needle, but the struggle to define algorithmic fairness goes beyond agreeing on a one-size-fits-all approach.
He emphasized that the main difference between a statistical algorithm and an AI tool is the number of data points and variables used to develop each. AI and ML tools typically require vast amounts of data, whereas statistical models can be developed using a significantly smaller pool of information.
Further, derivation and performance data for statistical algorithms are typically published, and the tools themselves are in extensive clinical use. With AI, information about the model might be largely unavailable.
"There are over 500 FDA-certified health AI algorithms out there, and I don't think I can get my hands on any one of them in terms of their performance metrics," Visweswaran said. "So, as a core tenet of transparency, we have to be able to fix that going forward. [AI tools] are currently not in extensive clinical use, but they will be as we go forward, and the efforts to evaluate bias in them are just beginning."
He further underscored that currently, it's unclear how many existing healthcare algorithms are racially biased, aside from the handful that have been researched recently. To address this, Visweswaran and colleagues developed an online database to catalog information about currently deployed race-based algorithms.
He noted that when looking at which of these tools might be biased, starting with those that already incorporate race or ethnicity as an input variable is a good first step, as these explicitly produce different outputs for different racial categories.
However, he indicated that continually updating the online database and evaluating algorithms that don't explicitly incorporate race is necessary to reduce disparities and improve outcomes.
"There are devices which are biased in terms of racial categories, [like] pulse oximetry … it was noticed that for darker-skinned people, the tool was not well-calibrated," Visweswaran stated. "By the time patients came to the hospital, they were actually pretty sick."
The same is true for devices like infrared thermometers and electroencephalograms (EEGs), which he noted do not work as well on patients with thick hair. This causes a disproportionate number of poor-quality readings for Black patients, which often leads to diagnostic issues down the line.
Further, poor-quality EEG readings cannot be used to develop algorithms, meaning that marginalized patient data might not be incorporated into a clinical decision support tool.
"Almost all the EEG data sets out there for research purposes don't have African-American data in them because it gets thrown out," Visweswaran explained, leading to the potential development of biased models.
This problem is exacerbated by the fact that the version history of an algorithm typically isn't available for researchers looking to assess a model's performance and fairness over time.
"When a new version of an algorithm comes, the old version disappears, [but] we need to track all these versions as we go along," he asserted. "We need a story for each of these algorithms -- which is freely available -- so that when researchers or developers go in, they don't have to start from scratch: they can go and look at versions of the algorithm, see the problems with a previous version and why the new version was developed. Sometimes, it's not quite clear that the newer version is actually better than the older version."
Alongside the need to track information about clinical algorithms, Visweswaran stated that stakeholders need to be mindful of how they conceptualize fairness. As part of the ongoing work to enhance its algorithm-tracking database, his team is developing "fairness profiles," which use fairness metrics -- like differences in sensitivity between groups -- found in the literature to assess each tool.
However, these are group fairness metrics, which evaluate measures across groups or populations.
"These are statistical measures, and they're in common use, but they don't guarantee that for a particular person, the algorithm actually is doing a good job," Visweswaran said. "All it guarantees is that for that particular group, on average, it does okay."
This knowledge has contributed to growing conversations around the role of individual fairness, which posits that similar individuals should receive similar treatments, and in turn, experience similar outcomes.
"The problem is that defining similarity between individuals is tricky, and right now, we don't have any standard measures which are available to measure individual fairness … The key challenge is to derive the appropriate similarity metric by which to decide who is the peer group that we are going to use for this particular person," Visweswaran noted.
A focus on coming up with one fairness approach that everyone can agree on might undercut the possibility that there is no single set of fairness metrics that will work well for each patient.
"Having this grand idea of getting to a fairer situation is great, but some of the devil is going to be in the details, and there might be math out there which says you can't do some of these things that you actually want to do," Visweswaran cautioned.
Shania Kennedy has been covering news related to health IT and analytics since 2022.