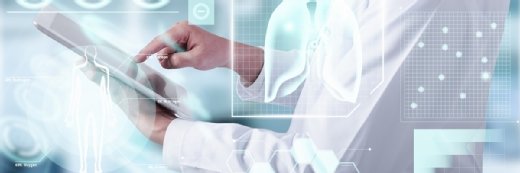
Getty Images
10 best practices for implementing AI in healthcare
To overcome hurdles and implement AI successfully, healthcare professionals must institute strong governance, routinely monitor progress, and develop secure and ethical models.
Healthcare's interest in artificial intelligence shows no obvious signs of slowing down. Major industry events have produced a steady stream of AI products and partnerships, particularly for popular use cases such as clinical documentation, process automation and data aggregation.
A vast majority of healthcare systems use AI, according to the "AI Adoption and Healthcare Report 2024" released late last year by the Healthcare Information and Management Systems Society in partnership with Medscape. The HIMSS survey of IT and medical professionals found that 86% of hospitals and health systems use AI and that 43% had been using the technology for at least one year. That's a significant increase from a 2022 American Hospital Association survey indicating that just 19% of hospitals were using AI.
Amid excitement about the technology's future are concerns about implementing AI in healthcare. "There's a high bar to meet basic administrative of business process needs," said Dr. Michael E. Matheny, a practicing internist and professor at Vanderbilt University. "It's important for all of us to consider the use of AI in a careful, measured way to respect the need to support patients and communities."
Healthcare leaders might not know what successful AI implementation looks like, said Dr. Saurabha Bhatnagar, a Harvard University professor, in a Harvard Medical School Trends in Medicine article. They might assume it's a matter of acquiring off-the-shelf software, he noted, or they could inadvertently focus on pilots that come with significant upfront costs.
To get over the hump, hospitals and health systems need to think carefully about what AI systems they want to implement, how AI models will be validated and monitored, and who will "own" the AI use case. While every organization's needs differ, there are 10 steps to implementing AI in healthcare.
1. Map out AI governance
The ideal AI governance structure will bring together expertise from IT, data science, the C-suite and bioethics, Matheny conjectured. The governance team will solicit proposals from business owners ranging from nursing leaders to subspecialty chairs to operational administrators. Proposals can come in different forms, said Dr. Brian Anderson, CEO of the Coalition for Health AI (CHAI). Some might propose the use of specific AI tools to solve a problem, while others identify inefficiencies or other challenges AI could solve.
2. Define goals and set expectations
The governance team's first goal in evaluating proposals is ensuring AI is appropriate. In certain cases, rules-based if-then logic could suffice. If AI is the answer, Anderson said, the next step is determining what type of AI is most appropriate, such as generative AI for content creation, deep neural networks for pattern recognition or traditional AI for data analytics. At this juncture, it's also the time to address the age-old build vs. buy question. Health systems with in-house data science expertise might be able to fine-tune an existing foundational model, Anderson explained, but if expertise is lacking, or if the solution set in question is complex, organizations likely will need to buy AI technologies.
3. Go to the market
For health systems looking to buy, Anderson recommended a formal, open request for proposal. He pointed to CHAI's registry for AI models as a starting point for evaluating vendors and making informed decisions. Business units, he added, need to be part of vendor evaluation and pay particular attention to how well an AI product aligns with the business unit's unique workflows. Procurement is a critical time for building vendor relationships, Anderson said, to make governance and performance monitoring easier down the line.
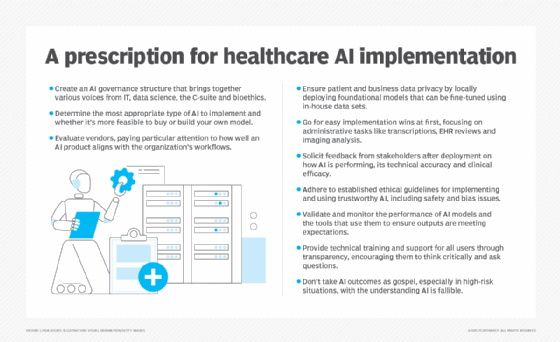
4. Ensure data privacy
There's controversy when it comes to using private patient and business data for AI models, Matheny noted. Algorithms need sufficient training data, but anonymizing data lowers its utility. Existing foundational models are widely available, but they share data with their commercial owners. To address this issue, Anderson recommended deploying foundational models locally so organizations can fine-tune models with their own data sets behind their firewalls.
5. Explore use cases with easy wins
The most common use cases for AI in healthcare, according to the HIMSS report, are augmenting administrative tasks such as transcribing notes and meetings, reviewing electronic health records (EHRs) and medical literature, and analyzing imaging studies. In the Harvard Trends in Medicine article, Bhatnagar indicated AI tools can be especially helpful for organizations transitioning to value-based care models. With so many variables to monitor, AI can automatically aggregate data and aid in analysis, enabling health system leaders to devote more time to improving outcomes.
6. Solicit stakeholder feedback
Both the AI governance group and business owners should convene regularly and discuss how AI tools are being used, Matheny said. Feedback on performance, technical accuracy and clinical efficacy are critical for determining an AI project's value. This ongoing review should become part of the organization's culture and structure, Matheny added, "especially as the breadth and depth of AI increases, the need to evaluate it isn't going to diminish."
7. Follow ethical standards
In summarizing ethical guidelines for AI from the U.S. and Europe, a Harvard Business Review analysis suggested several principles for implementing trustworthy AI in healthcare. In simple terms, AI systems should be safe, algorithms should be unbiased and account for the needs of vulnerable subpopulations, and patients should be informed when AI is being used and have the option to opt out. But bias can be tricky, Anderson noted. Some models are built with "justified bias," such as a breast cancer risk model for middle-aged black women, who, research has shown, are more likely to be diagnosed with more aggressive forms of cancer and at an earlier age. "You need to continually monitor the performance of these models against their intended use and also against other populations for unjustified bias," Anderson advised.
8. Validate and monitor models
Validating the performance of AI models and the tools that use them should include feedback from clinical and technical stakeholders. Organizations need to ensure products were implemented properly, the model is ingesting the right data and the model's outputs are meeting expectations, Matheny said. External validation of models could be necessary, he added, if in-house expertise is lacking. At the same time, increasingly sophisticated models could be better suited for internal testing because changes in the way a model's algorithms are weighed might not translate well to a different institution's test environment.
9. Provide training and support
To help clinical users determine whether they should use AI tools, Anderson proposed two questions: "Is this tool appropriate for the patient I see in front of me? How do I know?" For administrative and operational users, the questions should focus on the process rather than the patient. Organizations should encourage users to think critically and ask questions, Anderson suggested. Transparency is key. Users should have access to models and be aware of how the models were trained.
10. Understand the limitations of AI tools
When healthcare professionals encounter mature technologies with rigorous validation, they're unlikely to question an output. Matheny pointed to blood test results that have well-established and accepted benchmarks. The same can't be said for many AI tools, so end users need to be reminded that AI is fallible. "It may be wrong sometimes, and you may need to override it," Matheny said. The consequences of being wrong also need to be considered: An AI system booking two appointments at 11:30 is far different from an AI system prematurely shutting off a patient's ventilator.
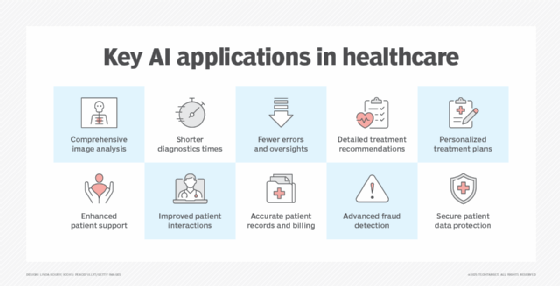
Don't let history repeat itself
Implementing AI in healthcare has improved productivity and reduced staff hours but has not yet reduced costs, according the HIMSS. The cost issue partly stems from AI technology being in its early stages. The initial AI infrastructure investment can be expensive, and it can take time to calculate and realize ROI.
As adoption accelerates, organizations would be wise to avoid the mistakes of EHR implementation, Drs. Christian Rose and Jonathan H. Chen of Stanford University wrote in the British weekly scientific journal Nature. The negative impacts of poor usability made many wonder if EHR systems were worth the trouble. For AI systems to avoid that fate, "[t]he effective integration of AI in medicine," Rose and Chen wrote, "depends not on algorithms alone, but on our ability to learn, adapt and thoughtfully incorporate these tools into the complex, human-driven healthcare system."
Therefore, organizations should take a pragmatic approach to AI implementation that emphasizes the importance of governance, routinely seeks stakeholder feedback and continually monitors AI tools for performance and accuracy.
Brian Eastwood is a Boston-area freelance writer who has been covering healthcare IT for more than 15 years. He also has experience as a research analyst and content strategist.