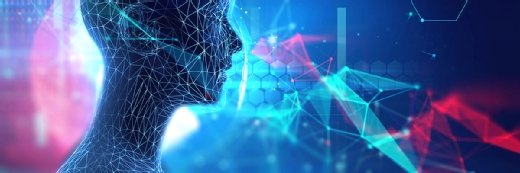
Getty Images
Using large language models to protect pediatric health information
Generative AI, such as large language models, may help tackle privacy and security concerns that can lead to pediatric data disclosures.
As generative AI continues to proliferate in healthcare, investigations into potential use cases and how these tools can be deployed in clinical settings are becoming more prevalent.
Listen to the full podcast to hear more details. And don’t forget to subscribe on iTunes, Spotify, or Google Podcasts.
While studies into the efficacy of the technologies are ongoing, many healthcare stakeholders have emphasized generative AI’s significant potential for risk stratification, medical imaging and reducing clinician burnout.
Large language models (LLMs), in particular, have received much attention since the release of ChatGPT in November 2022.
Some health systems are already utilizing LLMs to help clinicians draft messages to patients, but others are exploring how the tools could be used to promote health equity for vulnerable groups by addressing unintended data disclosures.
Pediatric patients – particularly adolescents – are at particular risk of unintentional disclosures of protected health topics.
A team from Stanford Medicine Children’s Health is investigating how an LLM could help prevent these disclosures by identifying confidential content within adolescents’ clinical notes, and health system leadership recently discussed the research on an episode of Healthcare Strategies.
PRIVACY, SECURITY CONCERNS IN PEDIATRIC CLINICAL DOCUMENTATION
Natalie Pageler, MD, chief health informatics officer and division chief of pediatric clinical informatics at Stanford Medicine Children’s Health, emphasized that there are a few major patient privacy and data security concerns that are related to clinical documentation for kids and adolescents.
“Pediatric data has increased regulatory requirements around it because of the vulnerable nature of the pediatric population. So, when you're accessing pediatric data for research purposes, there may be extra barriers to getting access to that data,” she explained.
While these barriers help protect patients’ privacy, they also require researchers to take additional data protection measures and can limit how that data is used.
Conversations about pediatric data become more complex in the clinical setting, as the rights of children and their guardians must be considered.
“In general, parents have a right to most of their child's data. However, as the child grows up and gains increasing autonomy, they suddenly start getting a right to some of their data,” Pageler explained, adding that state laws vary nationwide, with some allowing adolescents the right to access some of their data without parental permission.
“So, it gets very complex making sure that you're both protecting that vulnerable pediatric data, in general, and then also clinically navigating who gets what data while trying to empower both the patient and the family with as much data as possible, but being appropriately protective of privacy issues,” she continued.
Navigating these complexities presents a unique challenge for clinicians, one Pageler and her team are exploring in the context of LLMs.
USING LLMs TO PREVENT PEDIATRIC DATA DISCLOSURES
Pageler’s research involves using LLMs to prevent pediatric data disclosures by flagging confidential content in patients’ clinical notes. She indicated that AI and machine learning techniques have previously been deployed successfully to achieve this goal.
“Usually, our goal is to get as much data to the patient and family as possible to really empower both to take care and manage their health appropriately,” she explained. “But because of unique situations and variable state laws, we do need to make sure that we are appropriately preventing disclosures and protecting data appropriately.”
AI and machine learning are useful for this because of their ability to analyze a wealth of data, both structured and unstructured, in a patient’s medical record. These tools’ pattern recognition capabilities are very effective at determining what kind of information is present in clinical notes, and whether some of the data is confidential and, therefore, should not be disclosed without the patient's permission.
This is also useful in the context of patient portal messaging, as care teams must be mindful of what they disclose in each message in case it is the patient’s parent or guardian, rather than the patient themselves, on the other end.
Pageler emphasized that confusion during patient portal setup can contribute to accidental disclosures to a patient’s parent or guardian. Her team has successfully used AI and machine learning to address these issues.
This was before the advent of LLMs. Today, the enormous potential of these tools is leading researchers to explore their implementation in a variety of applications, including clinical documentation and the prevention of data disclosures.
Pageler noted that all tools have pros and cons, and despite their significant promise, LLMs have their trade-offs, as well.
“On the one hand, [LLMs’] proficiency in processing complex language patterns is unmatched, and so we recognize that maybe there was an opportunity to test them on this work that we were already doing to gain efficiency and accuracy,” she said.
Part of her research thus far has involved evaluating the efficacy of existing LLMs to flag confidential information in clinical notes, as the approach had the potential to be significantly less resource-intensive than building custom AI and machine learning algorithms, which Pageler and her team had done in the past.
LLMs not only showed that they were efficient and accurate, but they also demonstrated the potential to advance health equity.
PROMOTING HEALTH EQUITY IN PEDIATRICS
Health equity has become a growing priority for health systems in recent years, with many efforts focused on improving health outcomes for historically marginalized and vulnerable populations.
Pediatric populations are just one of a host of groups that health equity initiatives are concerned with, and questions around bias and fairness in AI models have led stakeholders to call for robust testing and validation of these tools.
“One of the considerations I like to put forward is that when you're thinking about AI and large language models and equity, one of the equity lenses that we need to be looking through is that pediatric lens: are we appropriately considering the equity issues, the ethical applications of these tools in the pediatric population? Of course, it gets incredibly complicated, partly because there are extra protections around the pediatric data, and partly because there's this complex relationship of who can even consent to use the data,” Pageler noted.
Questions around whether a child or their guardian needs to consent and how much of a child’s data a guardian can consent to release over time present conundrums for health equity researchers.
These are further complicated by the size of many pediatric datasets. Pageler indicated that because children are generally healthy, pediatric datasets are often much smaller than similar ones for adult populations. This makes pooling pediatric datasets from a variety of sources necessary for research.
She further noted that pediatric populations are not monoliths. Differentiating between subgroups – such as neonates and teenagers – is necessary in studies, but doing so makes the data pools smaller. These factors can make it challenging to develop tools like LLMs, which typically rely on vast amounts of data.
“One concern is, as we're pulling out these new tools, do they even represent pediatrics? And do [the tools] have access to the data that's important for this population? Then, on top of that, we need to think about – either with large language models or other custom AI and machine learning algorithms – are they being tested appropriately in pediatric populations?” Pageler added.
She indicated that there are some use cases in which an algorithm created using an adult dataset can also be applied to pediatric data, but others require that the algorithm be trained specifically using pediatric datasets.
“So, it's incredibly critical that we figure out how to test these algorithms appropriately, in pediatrics and in different age groups, to determine whether or not they should be used in the pediatric populations,” Pageler stated.
She added that, when thinking about health equity, stakeholders must address questions about how the algorithm was developed. This includes whether it contains biases or inequities, how it should be applied and if doing so could increase disparities.
“Oftentimes, when you create these algorithms, what they pick up on are social determinants of health, that might prevent a patient or a family from getting to clinic. And so depending on how you use that algorithm, you can improve or exacerbate health disparities,” she continued.
Pageler illustrated this point by discussing the use of an algorithm to predict appointment no-shows. She explained that if such a tool was used to ensure that the clinic is full, then that may lead to already disadvantaged patients being sent to an overbooked clinic. This could have a negative impact on care quality.
Conversely, if that algorithm is used to identify patients who may need extra support to ensure that they can get to the clinic, the tool could help create opportunities to tackle access barriers and improve care delivery.
This example highlights that in the pursuit of health equity, AI tools and other technologies must not only be developed with equity in mind and tested appropriately but also applied to maximize equitable outcomes.
Pageler underscored that the deployment of AI in pediatrics is full of potential, but health systems must proceed down the path of implementation with caution.
“It's important to think about where are the potential opportunities for disparities, or for unintended consequences in pediatrics? [Health systems] want to build robust validation processes for any algorithm that they’re going to use and think about which populations across that pediatric age spectrum that it needs to be trained on,” she stated.
She also emphasized that being vigilant about potential privacy concerns and considering the nuances of the regulatory environment and the child-guardian relationship can help health systems adapt their AI approach appropriately.
Further, children’s hospitals are uniquely situated to pave the way for innovations in this area, as they already focus more heavily on the clinical informatics and information systems needs of pediatric populations. Pageler added that collaboration among children’s hospitals is key to ensuring that children everywhere benefit from the use of technologies like LLMs.