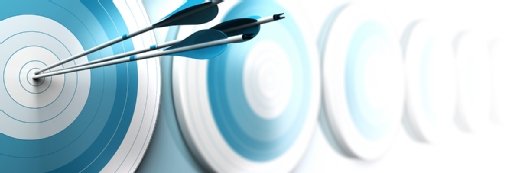
Olivier Le Moal - stock.adobe.co
Responsible AI Deployment in Healthcare Requires Collaboration
Leaders from Duke, Mayo Clinic, and DLA Piper discuss the need for cross-functional collaboration and industry standards to enable responsible AI deployment in healthcare.
Responsible, secure, and ethical artificial intelligence (AI) deployment in healthcare requires an informed, multi-disciplinary, and collaborative approach. But a lack of industry standards and consensus on how to responsibly deploy AI technologies has left many healthcare decision-makers looking for guidance.
Law firm DLA Piper, the Duke Institute for Health Innovation, and Mayo Clinic (among others) launched the Health AI Partnership in late December 2021 to help organizations navigate the AI software market and establish best practices for responsible AI deployment.
"There is so much excitement around AI and so much potential for doing good with AI in healthcare," David Vidal, a vice chair at Mayo Clinic's Center for Digital Health, who oversees the center's AI quality and regulation operations, explained in an interview.
"But at the same time, there's so much that people don't understand about it. Even the name AI means so many different things to different people, and there's such a rush to adopt and even a pressure to adopt when people don't know yet how to tell good AI from bad AI."
To deploy AI in a way that mitigates risk, key stakeholders must understand AI's use cases in healthcare, consider risks surrounding security, privacy, and ethics, and commit to collaboration.
Artificial Intelligence in Healthcare
Researchers and clinicians have applied AI and machine learning (ML) algorithms to everything from chronic disease management to mental healthcare and medical imaging. AI and ML have also prompted advances in predictive analytics and big data analytics.
Mark Sendak, a clinical data scientist at the Duke Institute for Health Innovation who plays an active role in the Health AI Partnership, spoke to the countless use cases for AI in healthcare that he has observed in his work. From kidney disease to community-based palliative care to heart disease, healthcare organizations can apply AI algorithms to a multitude of care settings.
Sendak also noted AI's usefulness in improving chronic disease management, monitoring inpatient deterioration, and weaving elements of specialty care into primary care settings.
Despite its benefits, AI technology has room to grow in terms of reliable standards and processes.
"There's so much that clinicians are trying to do with their care and the technology. Streamlining the workflow here or creating this efficiency there can be impactful for the clinician's ability to care for their patients," Vidal said.
"So with that, the AI field has been growing significantly. The benefit to patient care is a good consequence. I think the drawback, though, is the lack of process around the build and application or deployment of the AI."
In addition to a lack of structured industry standards, researchers have also noted instances of bias, often resulting from a lack of representative data. Inequities in data collection may lead to skewed outcomes.
"AI is different from other technology because it's not only a tool — in some ways, it is expected to replace human judgment," Danny Tobey, a partner at DLA Piper, suggested in the interview.
"That's a risky proposition, and we have to look carefully at where the division of responsibility between people and machines is going to land. And that's going to be on a product-by-product and technology-by-technology basis."
Considering Ethics, Security, and Safety in AI Deployment
As useful as AI and machine learning (ML) algorithms can be in healthcare, stakeholders must consider the numerous ethical concerns, along with security, safety, and privacy risks.
The Cloud Security Alliance (CSA) released a report detailing the many benefits and challenges of AI in healthcare. From an ethical standpoint, clinicians and developers must consider the potential for cognitive or algorithmic bias.
"Statistically, it is a systematic distortion of a statistic as a result of a sampling procedure," CSA explained.
"It results in a degree to which the result deviates from the truth. AI bias is an anomaly in the output of AI algorithms. Bias can contribute to harmful patient outcomes, resulting in differential treatment. The presumption is that bias is present throughout AI systems, and the challenge is identifying, measuring, and managing it."
Since AI relies on massive amounts of data to produce insights, its functionality and accuracy hinges on the validity of the dataset. Cognitive biases can seep into AI algorithms via developers unknowingly introducing bias or using an incomplete training dataset.
Along with ethical concerns, healthcare decision-makers must consider patient privacy. Healthcare organizations must be transparent about how they intend to use patient data and the possibility of bias or software issues.
AI technology often falls into a regulatory gray area, further emphasizing the need for transparency. Regulators did not introduce HIPAA with AI and ML in mind, and patients should know how their data will be used and safeguarded.
Any piece of technology with large amounts of protected health information (PHI) is a viable target for threat actors looking to hold that data for ransom or sell it on the dark web. With that in mind, organizations looking to integrate AI algorithms into care delivery and analytics must consider security.
"AI is a prime target for bad actors because people are used to relying on AI without understanding how it gets to its answers, which makes it easier for people with bad intentions to fly below the radar," Vidal suggested.
"So, how do you secure these systems, especially with the need for interoperable and transportable patient data? We need to let the good guys in and keep the bad guys out."
Part of the Health AI Partnership will revolve around assessing and creating best practices for AI security, including establishing standards around penetration testing. Stakeholders must work across functions to manage risks and maintain ethical standards for AI deployment.
Who’s Responsible for Safe, Secure AI Deployment in Healthcare?
"It's not just regulatory, and it's not just data science, and it's not just machine learning. The more collaboration we have, the better we can help people figure this out as both regulations and industry standards evolve," Tobey emphasized.
"My experience in this field is that everybody wants to do the right thing. They're just looking for a little bit of guidance about what that right thing means."
While the Health AI Partnership's primary audience is stakeholders within healthcare organizations making procurement decisions, Tobey predicted that a secondary audience would be product developers.
To deploy AI in healthcare responsibly, everyone from the developers to the providers must be on board. AI is advancing rapidly, but it is not a completely self-sufficient, hands-off process.
"Health systems have experience with doing due diligence on externally built technologies. And we need to draw upon that for this new generation of technologies," Vidal added.
The need for collaboration has been exacerbated further by a lack of regulatory guidance.
"A lot of people just point fingers to [ the Food and Drug Administration (FDA)] and say more needs to be done on regulation," Sendak stated.
"There may be certain things that FDA can promote, but part of it is also going to be identifying who will have what types of responsibility in this ecosystem."
Tobey reasoned that the FDA serves as a good role model for AI regulation.
"I think they are an example of a federal agency that has been ahead of the curve in developing guidelines and tools for helping the people producing AI to make the right decisions in advance," Tobey asserted.
Essentially, no single party in the development, procurement, or regulation stages of AI deployment in healthcare is responsible for its safety and effectiveness.
"We need to make sure that the people taking that data and running with it know what it means and how to use it," Tobey added.
"It's not just training people how to choose the right AI; it's training them on how to use the AI properly."
Developers need to prevent bias in their algorithms, decision-makers at each organization have to evaluate the safety and security of each product, and regulators must provide industry guidelines to ensure responsible AI deployment in healthcare.