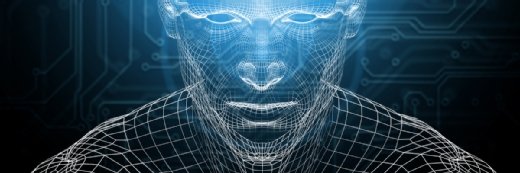
Getty Images
Overcoming struggles to define algorithmic fairness in healthcare
Medical ethicists posit that there is no one-size-fits-all definition of fairness for healthcare AI, necessitating a more nuanced, collaborative approach.
Potential use cases for AI in healthcare continue to grow as the technology rapidly advances. However, the potential for AI to enhance clinical decision support, chronic disease management and population health efforts has been checked by concerns over pitfalls like model bias and fairness.
In an era where health systems in the US are increasingly pursuing health equity, the question of whether an AI is fair is key to advancing patient outcomes. But, this question sheds light on a conundrum for researchers and ethicists: defining fairness is not straightforward.
This is the crux of a November 2023 opinion article published in PLOS Digital Health by a group of researchers from Stanford and Emory University. In it, the authors posit that defining algorithmic fairness from an American perspective is politically and ethically fraught for various reasons.
The article maintains that a one-size-fits-all definition of algorithmic fairness remains elusive despite prominent healthcare organizations emphasizing the importance of fairness in AI models. Approaches to improve fairness exist, but they are limited in various ways.
The researchers argue that this lack of a universal definition makes regulating healthcare AI difficult and necessitates that algorithmic fairness be understood and operationalized within specific use contexts.
To achieve fairness in these context-specific models, the authors suggest that collaboration between patients, providers and model developers be fostered and maintained from the outset of model development.
John Banja, PhD, corresponding author of the article, professor in the Department of Rehabilitation Medicine, and medical ethicist at Emory University’s Center for Ethics, recently sat down with HealthITAnalytics to discuss the considerations for navigating algorithmic fairness.
COLLABORATING TO PROMOTE FAIRNESS
The article highlights that existing ethical guidelines on the deployment of healthcare AI often gloss over fairness or provide definitions of the term that are too vague to meaningfully inform model development.
Other qualitative, statistical approaches to achieve fairness have been proposed in medical literature, and while these may seem more robust than a simple definition, they, too, have significant limitations.
This led Banja and colleagues to conclude that “fairness comprehensions and applications reflect a vast range of use contexts,” requiring those impacted by the model’s use to come together to determine how fairness should be conceptualized and operationalized during algorithm development.
These collaborations would serve not only to increase transparency within model development but also to ensure that provider and patient perspectives are incorporated to improve fairness.
Collaborative efforts to bring together providers, developers, patients, and others are already underway in the healthcare sector, with initiatives like the National Institutes of Health’s AI/ML Consortium to Advance Health Equity and Researcher Diversity, the World Health Organization’s Global Initiative on AI for Health and the Coalition for Health AI.
While some have expressed skepticism about the role groups like these will play in the industry as companies across sectors seek to profit from the AI boom, Banja indicated that they can help bring together stakeholders to debate issues like transparency, fairness and justice.
Even those looking to make money from innovations in AI will likely be forced to consider the moral and ethical implications of the technology’s use in healthcare, Banja explained.
“If you're an AI developer, you might not have a moral molecule in your body. You may be just in it for the money,” he noted. “But here's the thing: nobody wants to put out a model that is unfair or discriminatory because what happens there is you risk reputational loss, and everybody cares about that, whether they’re ethical or not.”
This pressure, alongside efforts from industry groups and the federal government to advance fairness and equity in AI development, contributes to an environment ripe for collaboration. Banja stated that model developers will need those affected by the AI to provide valuable insights and additional considerations.
On the other hand, for health systems and hospitals considering leasing or purchasing an AI tool, having the proper personnel and tools can make evaluating the solution easier.
“That hospital needs to have a good informatics team who's going to be able to look at this model to test it, to evaluate whether or not it's accurate within various [patient] populations,” he said.
However, Banja added that many health systems are taking a “wait and see" approach to AI because they lack the necessary resources to deploy it successfully or are wary about the technology’s pitfalls.
Some are concerned that clinicians’ responsibilities associated with AI tools — such as contributing significantly to fairness efforts will create more work for already overburdened providers.
BURNOUT AND LIABILITY
Burnout is a persistent challenge for the healthcare industry, and efforts to ease clinical workloads have had mixed success. EHRs promised to revolutionize clinical documentation, but documentation burdens remain a major driver of burnout.
Clinical documentation improvement is one area where proponents say AI could help optimize clinician workflows.
“The hope, when we talk about electronic health records, is that we're going to see AI relieve a lot of the documentation burden of doctors and nurses,” Banja explained, predicting that advanced tools like generative AI will soon become vital documentation workflow solutions. “In the not terribly distant future, we're going to have these large language models that will create a clinical note for a doctor. They’ll create a bill, they’ll send the bill to the insurance company, and they’ll keep track of the bill. If the bill isn't paid, they'll remind the insurer, ‘Hey, we're waiting on payment.’ When payment is received, the AI model will deposit the reimbursement in the appropriate account.”
While AI technologies present significant opportunities to streamline administration and billing, much of the hype around healthcare AI centers on how the technologies could be applied in clinical settings.
Considering factors like fairness and other ethical concerns at this relatively early stage of AI deployment helps set the stage for a smooth transition. Banja emphasized that stakeholders must think about how providers will use these tools and what the consequences of those use cases might be.
One consideration that has sparked significant debate is liability. Policies and regulations regarding when providers are held liable for adverse outcomes are essential for ensuring that clinicians uphold the standard of care expected of them while protecting patient safety.
But in the case of AI, the potential to analyze mountains of data to make more accurate, nuanced care recommendations could come at the cost of care quality if the models are biased or their accuracy dips over time. The results could see health disparities perpetuated and adverse outcomes increasingly befalling patients.
The potential risks and rewards of using these tools are significant, leading prominent medical organizations to weigh in on how liability should be conceptualized in the context of AI use.
The Federation of State Medical Boards (FSMB) recently published AI governance guidelines asserting that clinicians are responsible for their use of AI tools and should be held accountable for any harm caused as a result. This contrasts with the stances of other organizations — like the American Medical Association — on AI-related liability and malpractice.
A key aspect of this debate centers on concerns about the fairness and biases of these models and clinicians' hesitant trust in AI outputs. But could a focus on model developer-clinician user collaborations in model fairness help address some of the concerns around who’s liable in the event of an AI-related adverse event?
Banja indicated that handling these liability issues, at least in part, requires assessing the role of the AI tool and to what extent it augments or replaces a clinician. Additional considerations come into play if the AI and the clinician disagree.
“[If a model] tells us, with regard to a particular patient, that there's no cancer following a mammography — and it turns out that it’s wrong, it missed a cancer — well, the developer should probably be liable for that, if that model has replaced the doctor,” he said.
But Banja explained that this issue will become increasingly complex as AI models improve and clinicians come to trust them, noting that even a relatively high-quality, trustworthy model is not immune to making mistakes.
“When you and I go for our physical, and there's blood drawn, they send it off to diagnostics, and then what tools do they use? They use all kinds of technology, and then they send the report back,” he stated. “Well, we depend on that technology to be accurate, and it is usually 99% of the time.”
But the 1% of the time when the model isn’t accurate — whether the inaccuracy stems from fairness issues or other factors — presents a conundrum.
Banja suggested that collaboration between providers and medical groups provides an opportunity to tackle these issues, as well.
He suggested, “If you're a radiologist, work with the American College of Radiology, work with the Radiology Society of North America; work with your professional groups, bring the lawyers in, to establish the clinical guidelines or the standards of care” so that, in the event of disagreement between an AI and a clinician, those guidelines can be used to adjudicate.
“[Using these guidelines,] do you take it to the patient? Do you go along with the model? If the model is very accurate, how do you navigate a situation like that?” he said.
While the culmination of these efforts remains to be seen, Banja indicated that groups can begin the process by assessing the current standard of care, what fairness concerns exist, and how those shift as AI is implemented into clinical workflows.
These considerations also underscore the importance of transparency during model development and deployment.
TRANSPARENCY
Banja and colleagues argued in their article that transparency is crucial in the pursuit of model fairness.
This raises a number of questions that healthcare stakeholders have posed in the black box AI debate: Should model developers be required to make an AI’s decision-making process opaque to foster trust and mitigate concerns about fairness and bias? Is it even possible to make a complex machine learning model, which may be capable of analyzing millions or billions of data points, transparent?
In its recent recommendations discussing AI governance and clinician liability, the FSMB asserts that black box models shouldn’t necessarily be avoided altogether but that providers using them “should still be expected to offer a reasonable interpretation of how the AI arrived at a particular output (i.e., recommendation) and why following or ignoring that output meets the standard of care.”
Proponents of transparency posit that a transparent model allows users to see its inner workings and gauge how it arrived at a decision, but others hold that while one may be able to look inside a model, the tool may be so complicated that a human would not be able to gain insights into its decision-making.
Banja acknowledged that as models become more sophisticated, AI may become powerful enough to analyze data and identify patterns too complex for humans to see. This raises questions about how to build trust and alleviate concerns about model fairness.
The challenge presented by the deployment of these black box models in clinical workflows is particularly prickly. The implication is that patients and providers must trust these models based on their past performance records rather than by understanding how they work to achieve results.
Banja illustrated the issues this scenario presents in the context of a standard patient-provider interaction: if a patient questions how a clinical decision support AI comes to its conclusions, their provider may only be able to offer a limited explanation based on what is known of the model’s development process and its performance history.
“Suppose that physician is sued, and the plaintiff’s lawyer gets that doctor on the witness stand and says, ‘So, doctor, do you mean to say that you made a decision based on a model, and you didn't know how the model made this decision? You just went along with what it said.’ And that's going to leave the physician in the lurch,” he stated.
Banja further noted that such a situation isn’t outside of the realm of possibility, as large AI developers may push back against regulatory efforts because compliance, especially in healthcare, is extremely expensive.
“If I'm an IT company, maybe I'm going to spend a lot of money on lobbyists, but I'm going to want those lobbyists to lobby for regulations that are in my favor,” he explained. “And maybe those regulations are not going to be patient-centered regulations. They may be business-centered, ‘protect my bottom line above all’ [regulations], which would actually mean that we're going to see companies being anti-regulatory.”
As AI becomes more prevalent in the healthcare industry, the relationship between fairness, liability, ethics, transparency, and regulation is likely to become more complex. Banja emphasized that stakeholders will need to collaborate to navigate each issue and ensure that models advance patient outcomes and health equity.