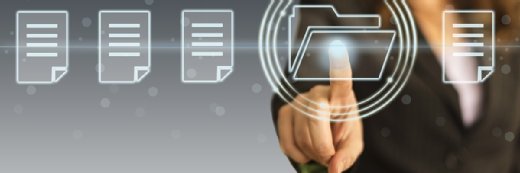
Getty Images/iStockphoto
Leveraging Synthetic Data for COVID-19 Research, Collaboration
Researchers at Washington University are using synthetic data to accelerate COVID-19 research and facilitate collaboration among healthcare institutions.
While the spread of COVID-19 has presented healthcare with many challenges, the sheer amount of data generated by the pandemic has been one of the biggest tests the industry has faced so far.
For more coronavirus updates, visit our resource page, updated twice daily by Xtelligent Healthcare Media.
Attempting to make sense of a global health crisis in a way that will be helpful to particular communities and populations is a task that organizations have struggled with since the virus hit the US.
Producing truly actionable insights from patient data to improve care and inform potential treatments has also been a major hurdle. With privacy protections and data aggregation issues, healthcare researchers often don’t have the means to move forward as quickly as they need.
“When we try to either aggregate or share large amounts of patient-derived data, we’re faced with the challenge of producing enough data to be able to ask and answer our primary questions, while simultaneously protecting the privacy and confidentiality of those patients,” Philip Payne, PhD, associate dean for health information and data science and chief data scientist at Washington University, told HealthITAnalytics.com.
“In the past, we've done that by removing certain identifiers, changing dates, and making other modifications so that it’s hard to reidentify the patients from whom the data is derived. However, this impedes a lot of the analysis that we want to undertake because the data that we might remove to protect privacy are the very same data we need to analyze.”

Standard methods of data access and collection can also hinder researchers from uncovering meaningful insights, Payne noted.
“If you were to go through the traditional way to gain access to patient data for research, you might have to go through additional training. You'd have to wait for someone to provision that data to you. Oftentimes, the data isn’t exactly the dataset that you want. This may or may not require you to go back and repeat the regulatory approval process, which can take weeks, months, or even years for very complex projects,” he said.
“When you think about modern data science methods, this process is the opposite of what we need. Most of those methods involve running an analysis, looking at the results, and then optimizing and rerunning your analysis until you arrive at a useful conclusion. The traditional way of thinking about accessing data for research is not well aligned with modern data science methods.”
Payne and his colleagues, together with government research centers and other healthcare systems, are working to overcome these challenges using synthetic data. Through the MDClone platform, institutions are leveraging synthetic copies of healthcare data collected from actual patient populations that is not linked to personally identifiable information.
“The platform basically looks at the distribution of all the different features for a cohort of patients, and then produces a new set of synthetic patients. If you perform a statistical analysis or you train a machine learning algorithm on the synthetic data, the results that you will produce in aggregate will be the same as if you had used the source data,” Payne explained.
“However, if you were to drill down in that computationally derived synthetic data, there's no way to link those individual records to the identities of the people from whom that data was informed. That means we can produce that data quickly, and we can share it more readily.”
With synthetic data, researchers and providers can come to actionable conclusions faster than they would using traditional methods.
“It's safer for our patients. And the time to insights is greatly reduced because we don't have to go through all the laborious regulatory processes that are normally associated with analyzing potentially identifiable patient data,” Payne said.
“We take that process that previously took weeks, months, or maybe a year, and we turn it into minutes or hours. That's incredibly important when we try to use these advanced data analytics methods to arrive at important conclusions about the data that we have and to produce timely results.”
In the midst of the current health crisis, the use of synthetic data could prove transformative, Payne stated.
“The COVID-19 pandemic is unfortunately a fantastic use case for this, because our metrics for success in terms of producing data analytical results in the research arena aren't measured in weeks or months. They're measured in hours and days because of the severity of the pandemic, the number of patients we're seeing, and how critically ill those individuals are,” he said.
“It's a great example of the value of this type of platform and its ability to accelerate data insights for both research and operational purposes.”
Even after the pandemic is over, these new methods could pave the way for improved collaboration and data sharing among healthcare organizations.
“COVID-19 is teaching us that there's huge value in collaboration across and between sites to examine larger datasets and to ask and answer questions that each individual institution does not have enough data on their own to address,” Payne said.
“As synthetic data platforms continue to mature and we become more experienced with them, we're opening the door to that type of collaboration. The current circumstances will accelerate the uptake of these types of technologies and really accelerate the formation of larger data sets that span traditional organizational boundaries.”
The COVID-19 pandemic has catalyzed change in the industry, most of which will hopefully last longer than the crisis does.
“This is an example of what happens when academic health centers, integrated delivery networks, and partners in the private sector come together with a shared interest of leveraging the technologies and capabilities we already have to build bigger, better, and more impactful data sets,” Payne said.
“Healthcare is moving away from building more and more infrastructure to thinking more pragmatically about how to use the data and tools and technologies we already have in more impactful ways. Forming these collaborative networks is a central feature of that.”