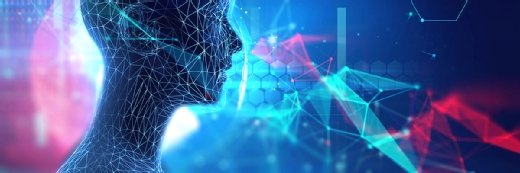
Getty Images
Leveraging Artificial Intelligence to Support Medication Adherence
Researchers are exploring how AI could enhance medication adherence, but considerations to prevent bias and system misuse are critical.
Medication adherence remains a persistent, complex challenge for the healthcare industry as drug costs rise and prescription adherence rates sink.
The Centers for Disease Control and Prevention (CDC) report that adherence to prescribed medications correlates to improved clinical outcomes and reduced mortality, especially for chronic disease patients. However, roughly one in five prescriptions in the United States are never filled. Of those filled, 50 percent are taken incorrectly.
Various strategies and approaches have been proposed to enhance medication adherence, including medication reviews, medication synchronization, adherence packaging automation, increased pharmacist involvement in medication management, and social determinants of health (SDOH) interventions.
In recent years, though, healthcare stakeholders are increasingly turning to digital tools to tackle prescription adherence, including artificial intelligence (AI). Sheana Bull, PhD, professor of community and behavioral health and director of the mHealth Impact Laboratory at the Colorado School of Public Health, is one of the researchers whose prior work and current research investigate how AI applications could be leveraged to enhance medication adherence. Bull spoke to HealthITAnalytics via email about the key considerations for stakeholders looking to leverage these tools.
CHALLENGES AND IMPACTS OF MEDICATION ADHERENCE
Bull noted that medication adherence has a significant impact not only on patients and their loved ones but also across the healthcare industry.
“Medication adherence has a substantial impact on both health outcomes for patients and system costs for providers and insurers,” she explained. “For patients with chronic cardiovascular conditions (which are among the most common chronic conditions in the US), nearly three of every four patients do not always take their medication. This is documented as a contributor to one-third of medicine-related hospitalizations, causes almost 125,000 deaths in the US annually, and nearly $300 billion in healthcare system costs.”
These costs, she added, aren’t going to disappear anytime soon.
“About 50 percent of US adults will develop at least one chronic condition by age 45; by age 65, [that number rises to over] 85 percent. The number of people in the US with chronic conditions is expected to grow, so these problems will likely grow as well,” Bull stated.
Despite these challenges, multiple strategies can help support improved medication adherence.
Bull indicated that the main reasons for non-adherence include forgetting if one took a dose, missing a dose, taking a lower-than-prescribed dose, not refilling current medications on time, not refilling a new prescription, or stopping the medication altogether. Simple interventions could help a majority of patients having issues with medication adherence, she explained.
“Research that includes reviews of medication adherence interventions show that programs focused on self-management of chronic illness can be very effective,” Bull noted. “The simple and low-cost interventions with demonstrated evidence around medication self-management include using pill boxes to store medications; setting reminders to take medication (e.g., on your phone or calendar); or having external reminders (e.g., from the pharmacy or a family member).”
The success of these more traditional interventions relies on several factors, some of which are easier to tackle than others.
LIMITATIONS OF TRADITIONAL MEDICATION ADHERENCE INTERVENTIONS
According to Bull, traditional medication adherence interventions have two major limitations: failure to time and structure strategies to help those who need them most and scaling interventions across large numbers of patients to amplify each intervention’s impact.
To address these challenges, Bull and her colleagues are investigating the use of AI and machine-learning technology to support medication adherence. Their current research, funded by the National Institutes of Health (NIH) National Heart, Lung, and Blood Institute (NHLBI), is looking specifically at medication refill adherence.
To address the first limitation, Bull and her team are working with three healthcare delivery systems: the Rocky Mountain Regional Veteran’s Administration, Denver Health and Hospital Authority, and the University of Colorado Health. Using patient data from these health systems, the researchers are investigating the efficacy of an AI chatbot.
“We have developed a machine-learning algorithm that identifies patients through pharmacy record systems who have a delay of seven days or more in refilling a cardiovascular medication,” Bull said. “Once identified as having a gap in medication refill, we are conducting a randomized trial to examine whether persuasive and engaging text message reminders or a text message reminder with additional support from an artificially intelligent chatbot can provide the information and motivation to patients to get medications refilled in a timelier manner than patients who either don’t get any reminders or patients who get a generic text message reminder.”
The chatbot can also engage with patients to assess their primary reasons for not refilling medication and direct them to resources, she stated.
Over 9,000 patients across the three health systems have been enrolled in the study. This illustrates that her team’s automated systems can reach large numbers of patients — more than typical for medication adherence interventions — and successfully tackle the second limitation to achieving improved medication adherence, Bull noted.
The researchers expect to have the results of their study ready to disseminate in the coming months.
REDUCING BIASES, DISPARITIES IN MEDICATION ADHERENCE AI
In helping to develop the AI tool used in the study, Bull had to consider health equity and model fairness, as the possibility of bias is one of the major concerns surrounding the use of AI systems in healthcare.
If algorithms are not rigorously developed, tested, validated, and re-evaluated, they can misclassify patients or suggest interventions that aren’t needed, which can negatively impact patient outcomes.
“We need to carefully consider algorithms used in AI systems to make sure they aren’t making any prior assumptions about whether patients are adherent or non-adherent and their reasons for non-adherence,” Bull said. “We need to make sure [before] using AI systems [that] people cannot be penalized for their health behavior — so, for example, we do not want insurance carriers to be able to raise rates or co-pays for people who have been identified as non-adherent previously.”
In their study, Bull and her fellow researchers leveraged multiple strategies to ensure that the AI model would avoid bias and mitigate health disparities, including regularly reviewing the algorithm to make sure that patients identified as having medication refill gaps had verifiable gaps.
“Sometimes patients are prescribed a new medication, and the earlier medication is still indicated on their chart. If they do not fill the earlier medication, they may be flagged as non-adherent when, in fact, their provider has changed their prescription,” Bull explained.
To avoid perpetuating disparities in medication refill behaviors, the AI chatbot offers solutions for patients that address the more structural reasons people cannot fill their medications.
“For example, we link patients to information on how to find lower cost and generic medications, on how to utilize mail-order pharmacy services when transportation to refill medication is a problem, on how to qualify for additional financial support for medication,” Bull said.
The chatbot’s content and suggestions were informed by conversations with patients in each of the three participating health systems, which Bull noted helped identify the specific barriers those patient populations face in refilling medications.
The study also leverages a community advisory group of patients from each health system, which provides regular feedback on all aspects of the project, including its approaches to limit bias and perpetuation of disparities.
However, Bull emphasized that researchers don’t yet know everything they need to about bias and the potential for system misuse. For instance, there is growing consensus that the research community needs to consider factors like SDOH to address disparities.
She further noted that current best practices in research focused on reducing disparities in chronic disease management include fully integrating underserved populations in the design, development, and deployment of any disparity-reduction intervention.
But once potential biases within a model are addressed, AI systems can provide a wealth of crucial data that can bolster medication adherence efforts, Bull stated.
“One advantage of the system we describe and other systems that may deploy AI is that they can be linked to electronic medical records to document impact on medication refills, subsequent hospitalizations, and co-morbidities that are associated with medication non-adherence,” she said. “We can also document costs from continued non-adherence or cost savings from improved adherence. If we see that people can be correctly identified as eligible for engagement with an AI chatbot to help with adherence, we should document if this engagement leads them to refill their medications more quickly.”
The capture and continued monitoring of these data points are just the beginning of how a properly developed and validated AI tool can drive value for patients and providers, Bull concluded.
“AI systems can be carefully constructed to account for things like cost of medication, limited access to insurance and care, limited language literacy or proficiency in their engagement with patients,” she stated. “We have the capacity to utilize technology to make communications with patients highly tailored to individual circumstances, so we should leverage that and deliver more personalized, relevant messages to persons struggling to adhere to their medications.”