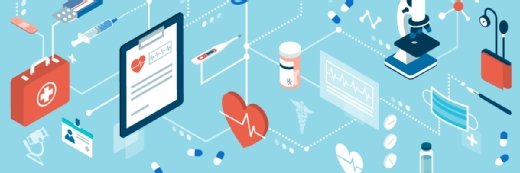
Getty Images
How NorthShore Uses AI, NLP to Tackle SDOH in the Emergency Department
NorthShore – Edward-Elmhurst Health is using natural language processing in the emergency department to minimize care disparities and improve health equity.
Identifying and tackling social determinants of health (SDOH) are crucial aspects of any health equity strategy. But establishing how best to capture patients\u2019 SDOH needs and develop interventions in the clinical setting remains a significant challenge for many healthcare organizations.
The United States Department of Health and Human Services (HHS) defines SDOH as \u201cthe conditions in the environments where people are born, live, learn, work, play, worship, and age that affect a wide range of health, functioning, and quality-of-life outcomes and risks.\u201d
Common social determinants of population health include education, health literacy, housing and neighborhood, food security, crime and violence, English language proficiency, transportation access, and social isolation.
However, additional considerations, such as climate change and environmental factors, adverse childhood experiences, and political determinants of health, are becoming more prevalent in conversations around SDOH and health equity.
Health systems looking to address disparities and advance equity in their patient populations must find an adequate way to combat these factors and avoid the weathering phenomenon, which states that discrimination can lead to adverse health impacts.
Some of the most effective SDOH interventions are centered around filling gaps created by social determinants needs, while others focus on broader population health management strategies. Best practices for developing an SDOH strategy recommend leveraging data analytics.
NorthShore \u2013 Edward-Elmhurst Health employs a combination of these strategies, but one of the most unique aspects of the health system\u2019s approach uses artificial intelligence (AI) and natural language processing (NLP) to flag the SDOH needs of emergency department (ED) patients.
Nirav S. Shah, MD, medical director of quality innovation and clinical practice analytics at NorthShore \u2013 Edward-Elmhurst Health, spoke with HealthITAnalytics via Zoom to provide insights into how this approach was developed and deployed, in addition to results the organization has seen since the implementation.
FINE-TUNING NLP TOOLS TO ADDRESS SDOH
One of the biggest challenges to tackling SDOH in the ED is that EDs are a critical front door to the hospital designed to stabilize and triage patients. However, lack of care access means that EDs are often tasked with addressing other needs as well, Shah explained.
\u201cThe fact that we have a huge access problem means that increasingly we're seeing more and more patients who are coming, not with the typical run-of-the-mill ED conditions, which need to be stabilized and then triaged, but more because there's social determinants of health that are preventing them from getting their care at a primary care office or an urgent care office,\u201d he said. \u201c[These patients are] showing up to the ED.\u201d
In short, EDs, already burdened with stabilization and triage, are now expected to deal with additional care needs like SDOH. While EDs are gradually getting more resources to tackle these issues, Shah underscored that they are not an ED\u2019s primary area of focus. Therefore, most are not built to fully address them.
To help combat some of these issues, NorthShore \u2013 Edward-Elmhurst Health leverages ED social workers, supporting them with AI capabilities. Historically, if an ED nurse identified a potential SDOH issue facing a patient, the nurse would document that concern, which would then be pulled into a list for the ED social workers. From there, a social worker would visit that patient and attempt to help rectify the SDOH gap, Shah said.
The health system built predictive algorithms to help identify patients at high risk of readmission or mortality. Social workers may also visit patients flagged by these algorithms to help determine if an unmet SDOH need is driving that patient\u2019s high risk.
However, these tools were not specific to social determinants needs or identifying SDOH gaps, which means that some gaps may be missed, leading to worse patient outcomes.
To address this, NorthShore \u2013 Edward-Elmhurst Health partnered with software company Linguamatics to leverage a rule-based NLP tool specific to SDOH. The tool uses named entity recognition (NER) to detect and categorize the key subjects, or named entities, in text.
Shah noted that while the tool came with pre-built queries that allowed the health system to understand and identify SDOH issues, hospital stakeholders wished to improve upon these further. Thus, the hospital created a gold-standard dataset to improve the algorithm and fine-tune the tool for Northshore\u2019s patient population.
The health system identified patients that fell into at least one of six SDOH-related domains: housing instability, food insecurity, transportation issues, mental health issues, anxiety and depression, and domestic violence.
The tool was able to pinpoint which patients have unmet SDOH needs and alert an ED social worker to help guide these patients toward appropriate interventions and resources.
\u201cSo, in the past, frequently, the ED social workers would be tuned into maybe a nurse picking up on something [or one of] these other high-risk conditions, which may or may not have an addressable SDOH need,\u201d Shah said. \u201cNow they're really focusing on the areas where there's key text in the notes indicating that, historically, there's been some kind of SDOH need.\u201d
\u201c[The ED social workers are] finding that they're able to better use their time, they're better able to target patients,\u201d he continued. \u201cOne ED social worker said this is a game changer because [it allows them] to really optimize their time to really focus on the patients that have addressable needs that they can focus on.\u201d
LEVERAGING EXPLAINABLE AI AND PRIORITIZING HUMAN INPUT
Pulling robust data about a patient population is key to effectively leveraging analytics to improve SDOH and population health, but health systems must work to ensure that these technologies don\u2019t further perpetuate care disparities or undermine health equity.
Shah indicated that these considerations are crucial for any healthcare organization looking to implement AI tools. Thus, NorthShore \u2013 Edward-Elmhurst Health has taken a thoughtful approach to its NLP SDOH solution.
After looking at the pre-built queries that came with the tool, the hospital\u2019s research coordinators conducted a retrospective validation in which patient data from across the health system was gathered and fed into the tool.
This served as the first phase of the effort to assess the solution\u2019s accuracy and potential biases, Shah explained. During this phase, research coordinators reviewed clinical notes and other data from patient records to determine if the insights generated by the NLP tool were relevant and robust.
Any issues flagged were addressed and reviewed by human research coordinators and data scientists until the model performed well on Northshore\u2019s patient population.
The second phase of the process involved further fine-tuning the model through prospective validation. This step tested the model\u2019s performance by looping in an ED social worker, who would take the information given by the model, speak with the patient, and verify whether the patient had the SDOH gaps the model said they did.
Evaluating the tool\u2019s accuracy and fine-tuning it in this way allowed Northshore to make it explainable to those using it, increasing trust in the model and highlighting its role as an assistive technology to human healthcare workers.
\u201cThe tool that we have is not a black box,\u201d Shah emphasized. \u201cIt's text phrases that you can use to identify certain text blocks in the [clinical] notes. Because it's not a black box, we can identify if there's any issues that may come up. It's not like this black box that's churning out and spitting out all these answers, and we don't know why. We actually do know why, and we can home in on that.\u201d
To illustrate this point, Shah described how even relevant queries within the model needed to be adjusted during prospective validation to make its insights more accurate.
\u201cWe noticed that for one of our mental health depression queries, one of the sub-queries from this... was gaining weight. So, weight gain, weight loss, these are some of the criteria we look at to determine if someone has depression,\u201d Shah explained. \u201cWhat was happening was we were flagging patients who were pregnant because they were gaining weight. So, we quickly picked that up and were able to alter the algorithm so that we stopped picking up pregnant women and assessing that every single pregnant woman was depressed.\u201d
Building trust in the tool through rigorous validation and human input is essential for health systems as questions around AI\u2019s role in healthcare and worries that AI may replace doctors become more salient.
"I think trust is so fundamental,\u201d Shah stated. \u201cOur ED social workers now, because they're part of this process [and] they're validating it, that insight is getting pushed back into the algorithms to make them better.\u201d
He noted that Northshore\u2019s ED social workers and data scientists meet regularly to discuss how the model is performing, potential issues, and future improvements to the overall strategy to improve SDOH in the ED.
This is a crucial aspect of tackling social determinants needs because it helps foster understanding and trust across stakeholders using the tool, which furthers interest in its continued use and improvement over time.
When NorthShore \u2013 Edward-Elmhurst Health was initially looking into technologies to help tackle SDOH in the ED, Shah stated that the health system explicitly wanted a tool for that use case but also one that could be applied in a wider array of use cases.
\u201cWe wanted something where it wasn't a black box, and we wanted something that was general, too,\u201d he said. \u201cAt that time, most of the things that were coming out were very specific deep-learning models or machine-learning models just on one specific area.\u201d
Thus, Northshore leadership opted for a more general tool that could be utilized in multiple areas while still capturing important nuances found in clinical text and phrases within EHRs.
By fine-tuning a general model rather than choosing a highly specific tool, NorthShore \u2013 Edward-Elmhurst Health has been able to tailor SDOH interventions to the needs of its population.
TAILORING SDOH INTERVENTIONS AND FLAGGING UNMET NEEDS
Since SDOH can vary significantly based on factors like education and geography, interventions must also be varied and personalized. Using insights from the NLP tool, Northshore has better informed its strategy to address SDOH gaps among patients.
Like other health systems, NorthShore \u2013 Edward-Elmhurst Health targets SDOH interventions in multiple categories, including housing, food insecurity, transportation, and domestic violence.
The hospital also leverages a tool that helps match patients with SDOH gaps to local non-governmental organizations (NGOs) and other resources to help bridge those gaps. Northshore has helped fund some SDOH-related programs in the communities it serves and leverages a community investment fund to establish some of these resources and meet additional needs.
In conjunction with the NLP tool, ED social workers also help flag areas where social determinants needs may exist that aren\u2019t getting enough attention.
One example is domestic violence and abuse, which ED social workers brought attention to when hospital leadership asked for feedback on how well the NLP model and the overall SDOH strategy were working.
Shah noted that these insights are critical. SDOH, like domestic violence, is often not captured when the patient meets with a nurse, as these interactions tend to be more focused on the clinical aspects of a patient's visit. Without the ED social workers and their expertise, many of these SDOH needs could go unidentified and unmet.
\u201cI think this is, like our ED social worker said, a game changer,\u201d Shah stated.
Moving forward, NorthShore \u2013 Edward-Elmhurst Health has a much broader vision for the AI tool since it is based on a generalized NLP model.
Shah described the expansion of the tool within Northshore\u2019s network as a "roadshow," in which the solution will be deployed in primary care for population-level SDOH analytics, noting that there\u2019s also a need to better leverage social needs data in that area. From there, the tool will help bolster a primary care-based approach that helps clinicians and social workers tackle SDOH, much like the approach being piloted in the ED.