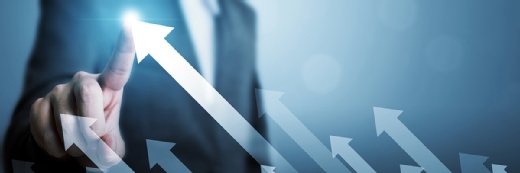
Getty Images/iStockphoto
How Machine Learning Can Guide COVID-19 Decision-Making
Developed by Regenstrief Institute investigators, a new machine-learning algorithm can help stakeholders make decisions on resources and policy regarding the COVID-19 pandemic.
As the United States continues to see high rates of COVID-19 hospitalizations, providers must make difficult decisions regarding allocating medical resources to patients who may need them most. While physicians can speculate about an individual's risk of hospitalization based on their co-morbidities, researchers are working on solidifying the process through a predictive analytics strategy using machine learning.
In a recent study by Regenstrief Institute and Indiana University, researchers showcased how machine learning models trained using clinical data from a statewide health information exchange can predict the likelihood of hospitalization for individuals with COVID-19.
“What we thought to do was to take advantage of this urgent need to create machine-learning solutions that could help healthcare decision-makers come up with broad population health-level decisions on the populations that they serve,” Suranga Kasturi, PhD, the study's first author and Regenstrief Institute research scientist, told HealthITAnalytics.
“The idea being with different types of the COVID virus coming out, and with the relaxations of various mandates, the need of care has changed quite a bit. We've gone from hospitals being very overburdened and constrained for resources to limited requirements of need.”
The study and what it found
For the study, the research team's goal was to develop an algorithm to effectively predict resource utilization for specific populations.
“Whether it be state, or county or some other region, can you effectively estimate for the next week or six weeks how much healthcare resources you are going to need,” Kasturi said.
The research team utilized clinical data from the Indiana Network for Patient Care (INPC) to train decision models that predicted healthcare resource utilization. They used data from 96,026 patients in all 957 zip codes in Indiana.
The INPC, a regional health information exchange developed by Regenstrief Institute and managed by the Indiana Health Information Exchange (IHIE), is the nation's largest inter-organization clinical data repository, containing over 14 billion pieces of patient data.
To assess the models for any potential biases, the team tested the models’ performances against subpopulations stratified by age, race or ethnicity, gender, and residence.
After proper training and testing, the research team concluded in their study that it is possible to develop decision models capable of predicting patient-level healthcare resource utilization.
However, researchers noted that the models present statistically significant variations in performance across different populations, highlighting the need for further research and development.
The study was supported by a Regenstrief Institute COVID-19 research pilot grant and Indiana University.
Machine learning helps make data actionable
When dealing with large amounts of data, Kasturi explained how machine learning could become a critical tool in decision-making practices.
By examining social determinants of health information and incorporating patient data into algorithms, machine learning can assist in identifying patients who are more likely to need medical resources such as ventilators due to COVID-19 hospitalization, Kasturi said.
Additionally, policymakers can use the algorithms to inform state and national public health decisions regarding mask mandates and reopenings based on population health data and community transmission.
“In the context of this study, having machine-learning solutions helps us leverage large quantities of data to help inform broad decisions that impact public and population health. Things that we just won't be able to do if there wasn't an AI-driven system to work with such large quantities of data,” Kasturi said.
Challenges of incorporating AI into medical decision-making
While AI can be a massive asset in medical decision-making, especially when handling large amounts of data, it is not without its challenges.
“We have invested a lot of resources on developing health IT infrastructure. That said, the large quantities of data that we collect aren't always readily available for analytical decision-making. They might be fragmented across different health systems. They might be stored within a broad health information infrastructure, but they just might not be available for actionable decision-making using machine learning,” Kasturi explained.
To overcome this issue, researchers used specialized analytics to put data into an examinable format.
Additionally, machine-learning algorithms must be adequately trained using diverse data to serve the patient population best and avoid algorithm bias. Before moving the technology into clinical practice, the research team must ensure the technology is working accurately and equitably.
“The models reported strong predictive performance. However, when we stratify them across subpopulations, there were certain disparities in performance across gender across the urban-rural divide. So yes, the models performed reasonably well, but there are things we need to do to improve that performance,” Kasturi said. “The next step would be addressing those disparities, ensuring that the model delivers fair and equitable care. Once that happens, we can have broader conversations in terms of how it can be integrated into existing public health workstreams.”
Continued testing of the algorithm and assisting the quality and diversity of the data is needed to ensure that it is trained accurately.
But, ultimately, the team's research can improve population health practices, according to Kasturi.
“The work we have done represents a significant potential to inform the future of population health. We are fortunate to have access to data from an entire state, and the work we are doing applies to the breadth of Indiana,” she said. “The methods that we created can be replicated across other states or even other broad US-wide initiatives. There's so much potential in terms of taking what we've done and moving forward with it.”