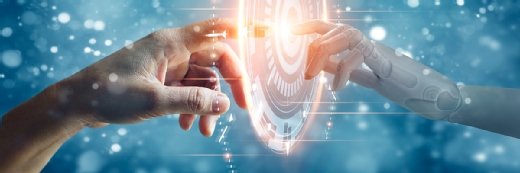
Getty Images
How Limitations in AI, Wearables Impact Depression Research
Utilizing artificial intelligence and wearables in the mental health space, particularly for depression and anxiety, is an exciting prospect for many, but multiple hurdles still stand in the way.
Artificial intelligence (AI) and wearables have seen an uptick in interest from the healthcare sector in recent years. With the rise in demand for telemental healthcare during the pandemic, the push for digital transformation in mental health to address care gaps has also grown.
But to bridge any care gap effectively and achieve whole-person patient care, healthcare providers need access to high-quality data. These data must be gathered from various sources, as patients usually do not receive all their care from one provider. Bringing these data together in the EHR is often accomplished through the use of AI, but AI’s impact on healthcare is much more far-reaching.
The expansion of healthcare AI and push for digital solutions in mental healthcare has begged the question: what role, if any, can AI play in mental health, especially for conditions such as depression or anxiety?
HealthITAnalytics sat down with Rosalind Picard, founder and director of the Affective Computing Research Group at the Massachusetts Institute of Technology (MIT) Media Lab and principal investigator at MIT’s Jameel Clinic, to explore this question as it relates to depression. Picard’s work has focused on providing emotion AI technologies and wearable sensors and analytics to improve health, and her current research is concerned with leveraging AI to assess the severity of depressive symptoms.
USING AI TO ASSESS DEPRESSION
Depressive symptoms are typically monitored using self-administered and interview-based assessment methods conducted by clinicians, both in-person and via telehealth. However, dwindling resources and the clinician shortage have severely limited or delayed this type of monitoring, according to Picard.
Picard and her team seek to evaluate passive recording of behavioral data, or gathering patient information without their direct input, as a potential long-term monitoring method. Specifically, the team is looking at a combination of wearable sensor technology and machine learning to measure behaviors and predict depression.
In the project’s pilot study, the researchers monitored chronically depressed patients over the course of eight weeks using wristband sensors. These sensor data, along with phone-based passive measurements, were used to create a model that could successfully estimate depression severity. For the project’s next study, the research team is planning to monitor 100 adults with major depression disorder who just started treatment. The goal is to develop an algorithm capable of detecting depression and predicting patient response to treatment.
THE COMMERCIALIZATION OF HEALTH-BASED WEARABLES
One of the major differences between the wearables Picard works with and more familiar ones like a Fitbit or Apple Watch, is that hers were designed specifically for medical research and applications from the outset, rather than incorporating health-related features later on.
The wearables that Picard and her team help evaluate and design are also not commercialized in the same way as other wearables, if commercially available at all. Empatica, the company that Picard co-founded to provide wearable sensors and analytics aimed at improving health, has commercialized a few such wearables, including the first Food and Drug Administration-cleared smartwatch for monitoring epileptic seizures.
However, most of these wearables are strictly for research, and they are purposely designed so that researchers can “open the hood” and access the raw data, which cannot be done with most other wearables, Picard noted. Currently, research related to these wearables is ongoing in areas such as neurology, psychiatry, oncology, and cardiology.
The researcher-friendly design is why most of these devices are not commercialized, Picard explained.
“[The wearables] require some real handholding on the back end that researchers know how to do, but the general public doesn't,” she stated.
Only the smartwatch for epilepsy has been made available to the public because it is both FDA-cleared and has a user-friendly interface, she continued.
In contrast, wearables to assess depression, like those used in Picard’s current research, could be refined to have patient-facing interfaces, but they would still be limited by other factors that prevent them from being viable for submission to the FDA.
THE LIMITS OF AI AND WEARABLES FOR DEPRESSION MANAGEMENT
The wearables used in Picard’s research can forecast depression and estimate severity of symptoms using electrodermal activity, but in this case, forecasting is not enough.
For example, the wearable that forecasts seizures can do so effectively, which allows patients or caregivers to seek medical attention or prepare for the seizure using evidence-based strategies recommended by healthcare providers. With a depression-focused wearable, translating the data and forecasting into action is not so straightforward.
“Even though we can track Hamilton Depression Rating Scale scores with a wearable, and an AI now, and one doctor's visit, we don't have a way to treat [patients] with [the wearable] yet,” Picard explained. “We really don't have a way to help them know what to do with the data yet.”
She added that the team could design a wearable that provided generic depression support to users, but as researchers, the team would rather focus on providing evidence-based support through the device and avoid rushing to commercialize it. By being sensitive to the burden faced by individuals with depression, the team hopes to gain a better understanding of their lived experiences and improve the devices significantly before even considering commercialization.
On top of these considerations, Picard also highlighted the importance of clinician input.
“You want the doctor in the loop, and the way you optimize the AI when you know the doctor's in the loop is different than when you think [the AI is] going to be trying to do something on its own… there's so little it can do on its own. It's always a very narrow thing,” she said. “So, even the Hamilton Depression Rating Scale score monitoring that we've got an AI predicting right now, it depends upon getting some baseline information from an expert doctor interview, and then once we have that, the machine learning is looking to forecast changes around that — to estimate changes based on just what it measures from your smartphone and wearable at that point.”
Even with clinician input and trust in the device, any patient-facing device, including the depression assessment wearable, would require users’ trust as well, which is a unique patient engagement challenge.
Despite the many hurdles for those who want to see effective implementation of AI and wearables in healthcare, efforts to create frameworks for analyzing wearables data, outline ethical AI standards, and implement evidence-based AI development and deployment approaches show that innovation in this area is ongoing and unlikely to wane in the near future.