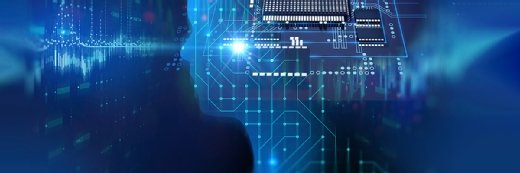
Getty Images
Healthcare Stakeholders Plan to Prioritize AI, Analytics This Year
Cedars-Sinai, Emory Healthcare, and Johns Hopkins Technology Ventures leaders share their top AI priorities in the new year.
As the new year begins, health systems are gearing up for new opportunities and challenges in the year ahead. For many, top priorities from last year — such as establishing analytics infrastructure, deploying predictive analytics, and promoting health equity — will carry over into 2024. But the question of what tools organizations will leverage and how they’ll deploy them remains.
Over the past year, rapid advancements in technologies like generative artificial intelligence (AI) have dramatically shifted the healthcare IT landscape, altering how some stakeholders are approaching their AI and analytics strategies in the year to come.
Leaders from Cedars–Sinai, Emory Healthcare, and Johns Hopkins Technology Ventures (JHTV) recently sat down with HealthITAnalytics to discuss their priorities for building on existing initiatives, deploying new projects, addressing challenges, and innovating in the AI and data analytics space.
CEDARS–SINAI
In 2023, Cedars–Sinai focused on leveraging AI in eight key areas: operating room (OR) optimization, patient deterioration monitoring, discharge planning, readmissions, physician efficiency, ambient patient monitoring, supply chain, and administration.
Mike Thompson, vice president of enterprise data intelligence at the health system, emphasized that these priorities will remain in 2024, with the addition of some new projects focused on efficiency and real-time monitoring.
Hospital flow optimization is just one of these efforts, which Thompson noted will involve leveraging predictive analytics to forecast emergency department (ED) volumes and boarding times, expected discharge time and delays, and patient length of stay.
Cedars–Sinai will also prioritize further OR optimization by utilizing AI and analytics to better understand which block times are available, which blocks are likely to be released and, therefore, could be given to other surgeons, and which surgeries will likely start and end late.
In the first half of the year, the health system is looking to incorporate generative AI in some areas. Thompson noted that large language models (LLMs) have significant potential for helping generate patient summaries. He explained that the technology could produce standard information about what’s happened with a patient since the clinician saw them last, in addition to transition summaries, which would capture what’s happened in the transition from one care team’s shift to the next.
“When generative AI first came out, [the health system] knew that everyone was going to be using ChatGPT, and we said, ‘Okay, how can this benefit healthcare?’” Thompson indicated. “So we had these AI idea-thons and got so many ideas from that.”
The health system generated 40 ideas and then narrowed them to 12 across various focus areas, including nursing, pharmacy, labs, administration, business development, strategic planning, and finance.
Despite these ambitions, deploying AI in a health system is challenging.
Thompson underscored that while these tools have significant potential, keeping a human “in the loop” at all times and establishing AI infrastructure is critical.
“The American Medical Association (AMA) just came out with principles around augmented intelligence, and one of them was focused on generative AI,” he stated. “AMA talked about needing a policy around understanding how accurate a model is… And so [healthcare stakeholders] will spend more time on that within the industry.”
“Something we started talking about at Cedars–Sinai a couple of years ago is when bringing an artificial intelligence tool into the healthcare setting, [health systems] need to have an assurance lab that vets the tool to [ask] ‘How did you train it? Was there bias? What kind of accuracy metrics do you have? What are the limitations of it? Are you using it appropriately and not inappropriately?’”
Understanding how an AI model was trained and its potential for bias is crucial, but doing so effectively and communicating those limitations to clinicians using the tools is another hurdle.
“Large language models are trained on information. Whether the information is correct or not, it was still trained on that information,” Thompson explained. “How do [health systems] surface that to a physician to say, ‘This information may or may not be accurate?’”
One approach that Cedars–Sinai is investigating involves using generative AI to assess patient messages and present clinicians with a potential response, but this requires significant model training. Further, it raises the question of how the health system will alert patients that AI is being utilized, which is something Thompson indicated that his team is working on in 2024.
EMORY HEALTHCARE
Emory made significant investments in AI in 2023, including a wide-reaching initiative to bolster research in these technologies across multiple disciplines.
“We've announced the AI.Humanity initiative, and the goal of that is to focus on bringing together Emory University and Emory Healthcare to help improve human health research, generate economic value, and promote social justice,” explained Joe Depa, who serves as the chief data and analytics officer for both institutions.
As part of the initiative, Emory launched the Empathetic AI for Health Institute (Emory AI.Health) in November, which is set to focus on developing accessible and equitable health AI to improve care while reducing costs.
Depa noted that to support the AI.Humanity initiative, the university has hired 39 new faculty members, with plans to raise this number to 60 in the coming year.
“The goal of the faculty in that initiative is to inject AI-related research and teaching into diverse disciplines, like liberal arts, healthcare, business, law, and ethics. Those programs should all have some components of AI,” he explained.
“Thinking about the healthcare system in general, that's going to be included in how we teach our upcoming medical school students and the next generation of providers and nurses as well,” Depa continued. “So, it's a really important time for Emory to make sure that we're taking all the learnings that we have across our university, our healthcare, our research, and we're using that to hopefully also train our next generation of students.”
Looking at 2024, Emory wants to enable data analytics more effectively across the university and the health system.
Depa has five areas he’s focusing on to make this happen: prioritizing “no regret moves,” enacting cross-enterprise data governance, breaking down data silos, recruiting talent, and leveraging a unified data platform.
Prioritizing “no regret moves” emphasizes that in a health system like Emory’s, there are essential tasks and use cases that “we just have to get right,” Depa noted.
“I look at Emory — we've made some significant technology investments, and we have some amazing technology — but we just haven't leveraged that technology to harness the value of that data,” he continued. “So, we're going to make sure that we're taking those technology investments, and we're going to drive adoption across our system so that that data can be used.”
His cross-enterprise data governance approach is two-pronged, focusing on ensuring that data is treated securely at all times while designing a system to harness the value of that data.
“Whether it's a provider being able to give better access to care, or somebody from our operations teams looking at the wait time and length of stay metrics to determine if there are workflow issues, [cross-enterprise data governance] can help reduce or increase the operational efficiency of our hospital system.”
Breaking down data silos, at least in part, is a question of the mindset and culture within an institution, Depa indicated. He highlighted that many academic health institutions traditionally have a university side, a healthcare side, and a research side, which results in data silos.
Until the invention of advanced technologies like cloud computing and AI, there was little incentive to undertake the laborious effort of breaking down these silos. Now, however, these large datasets are extremely valuable.
“Connecting that integrated data — whether it's genomic data, research data, outcome data — is super powerful,” Depa stated. “I want to unlock this data and make sure that we're putting technology in place to do so in a safe, secure manner while also giving our practitioners, providers, nurses, researchers, and faculty tools that they can use to leverage that data.”
In terms of recruiting talent, Emory is focused on developing pipelines to source undergraduate talent, secure senior talent, and promote data literacy for existing faculty and staff.
These four strategic priorities are all supported by the fifth.
“This is all powered by a unified data platform,” Depa explained, which enables end users with high-quality data elements that are trustworthy and secure.
“I am a strong believer in responsible AI, so that platform has to be leveraged in a way that it's going to enable access, but do so in a way that's going to treat the data in a very responsible and ethical manner,” he continued.
In addition to these efforts, Depa also indicated that the health system aims to leverage ambient listening, with patient consent, during clinical encounters. This allows patient–provider conversations to be recorded and transformed into clinical documentation that can be used in the electronic health record (EHR) or revenue cycle management.
The technology’s implementation is set to reduce clinician burnout while making clinical notes more accurate. At the time of writing, Depa stated that responses to this effort from the health system’s staff have been largely positive.
JOHNS HOPKINS TECHNOLOGY VENTURES
JHTV serves to help Johns Hopkins University researchers translate and commercialize their technologies and products. The technology development arm of JHTV focuses heavily on scouting high-value research happening within the university and facilitating the commercialization process, including for healthcare-related technologies.
“Particular to healthcare, there's a lot of different research and work around large data sets and how to do more with them, [especially] things like vital signs, lab data, and imaging data,” explained Christina DeMur, director of technology development at JHTV. “There's a bigger need to unlock the power of that data, and we're seeing lots of different groups try to tackle that.”
She underscored that “AI” has become a buzzword, and startups are eager to incorporate the technology into their products. But, at their core, AI and machine learning (ML) tools simply enable the ingestion of large amounts of data and help users make sense of that data.
As sensors and other technologies have improved, so has the ability to capture high-fidelity data, which leads to opportunities to leverage that data in industries like healthcare, DeMur indicated.
However, data integrity and sharing are major concerns faced by many innovators, including researchers at Johns Hopkins. DeMur and her team play a crucial role in helping those researchers determine the impact of their data and what can be accomplished with it.
“[AI technology is] almost like a new shiny tool: ‘Oh, look at my hammer!’ [and] everything is a nail,” she said.
She sees this trend continuing in 2024 but notes that as AI becomes more ubiquitous, people have become more aware of how these technologies can be researched and implemented to demonstrate their values.
In the coming year, DeMur and her team hope to help the university gain a more unified understanding of AI, ML, and how to leverage the data available to researchers.
“What we're seeing is all the different divisions coming together [to understand] the best way to use all these datasets and move forward with them, to understand the quality of the data,” explained Golnoosh Kamali, technology development associate at JHTV.
Not only having a wealth of data but also being able to mine it and gauge whether it truly represents a patient population is critical. Doing so requires bringing in relevant stakeholders, such as faculty from the university’s Bloomberg School of Public Health, and combing over health equity considerations.
However, these efforts are not without their challenges.
Variability in data sources, data quantity and diversity, and computational resources can all present obstacles to health AI innovation.
“If [health AI researchers are] trying to do something in imaging, like recognizing a tumor or finding a bone of some sort, there's a lot of different ways that those images get captured, that the patients get positioned, and in workflows,” DeMur emphasized. “So, they've developed an algorithm that works well in the ‘home base’ data acquisition environment — the scanners, workflow, and type of patients.”
However, that algorithm may not work well across other patient groups due to differences in data acquisition. She likened this phenomenon to driving two different types of cars: while the two may be similar, they most likely won’t handle the same way while driving.
“If you want [an algorithm] to work broadly across all different types of patients, you have to expose it to multiple data,” DeMur stated. “You have to know that you don't know the whole puzzle.”
Kamali further emphasized that many people underestimate the volume of data needed to train a health AI tool effectively and the computational resources required to run an algorithm of that size.
She noted that these challenges are not exclusive to the commercialization side of algorithm development but are also present on the health system side.
“The pathway for [health AI] research to evolve into a product, an approved product by the FDA, and then a commercial product that [healthcare facilities] can buy and integrate into their workflow, takes a long time. There's some frustration around that,” Kamali indicated, noting that on the one hand, health systems want solutions that can help boost efficiency and improve care, but on the other, those solutions must work for their populations and workflows.
“Both sides of the equation have the same challenges,” she continued.
For those looking to develop and deploy healthcare AI in 2024, DeMur and Kamali underscored that paying attention to not only how a tool might perform in a specific use case but also how easily that tool can be integrated into clinical workflows and what hurdles to adoption it might face are critical.