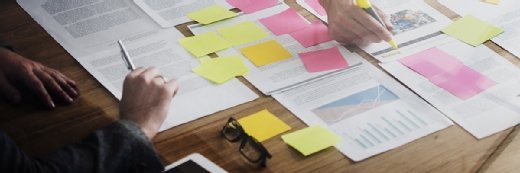
Rawpixel.com - stock.adobe.com
Exploring the National Academy of Medicine’s AI Code of Conduct
How does the National Academy of Medicine’s Artificial Intelligence Code of Conduct fit into the growing patchwork of healthcare AI governance efforts?
The question of how the healthcare industry can leverage artificial intelligence (AI) ethically and responsibly has become a hot topic in recent years.
Throughout 2022 and 2023, industry professionals, policymakers, and regulatory authorities launched a host of collaborative efforts to guide AI regulation in the right direction, including the White House Blueprint for an AI Bill of Rights and Biden’s Executive Order on Trustworthy AI. While these broad guidelines touch on various industries, including healthcare, more specific efforts are underway to help govern health AI.
The National Academy of Medicine (NAM) is spearheading one such initiative with the publication of its Artificial Intelligence Code of Conduct (AICC). The AICC is the result of collaboration between healthcare, patient advocacy, and research groups to detail the national architecture needed to support the responsible and equitable use of AI in the healthcare industry.
To discuss the AICC and how it fits into the current health AI governance landscape, HealthITAnalytics sat down with Michael McGinnis, the Leonard D. Schaeffer executive officer of the National Academy of Medicine.
THE GROWING ROLE OF HEALTHCARE AI
AI, machine learning (ML), and other computerized technologies are rapidly becoming a core feature of healthcare, making governance infrastructure desirable and necessary to ensure patient safety and promote health equity.
“[AI] is already used to accelerate processes for tracking and monitoring services provided in various clinical settings,” McGinnis noted. “But what's especially important about the technology at this stage is its application as a routine part of clinical care, both to collect information that's part of the clinical process and to analyze and introduce projected scenarios for treatment.”
He explained that the development of large language models (LLMs) and other types of generative AI expands the potential of healthcare AI in two main ways.
“One is a ‘universe of information.’ Meaning that the use of AI in healthcare in the past might've been focused on a fairly constrained set of information available to a single department or a healthcare institution, but now the theoretical boundaries are now virtually limitless,” he stated.
McGinnis elaborated that the advent of LLMs enables large-scale pooling of existing data, meaning that in the future, these pooling capabilities will likely become both more advanced and accessible for users.
In healthcare, this could lead to the growth of the information bases that providers use to make clinical intervention recommendations. This could enhance care by allowing providers to access a wealth of data points to help guide treatment decisions rather than relying strictly on their memory or potentially incomplete information from a patient’s chart.
The second dimension of generative AI’s potential lies in its ability to not only pool large amounts of data, but also make that information understandable for different audiences.
“Generative AI is essentially a thinking machine that teaches itself how to communicate and process. It allows for potential for substantially enhanced access to credible information,” McGinnis said.
He explained that this “self-teaching” and “self-learning” can enable users to query well-developed responses from an LLM that range from simple to highly complex.
This allows the structure and complexity of the information to be tailored based on education level, age, or other factors, which can help providers, patients, and families engage with that data in a more meaningfully to support care delivery.
However, for healthcare AI to reach its potential, stakeholders have to work out some of the persistent challenges plaguing the technology’s adoption.
HEALTHCARE AI DEVELOPMENT AND DEPLOYMENT HURDLES
AI can theoretically pool and tailor credible information for use in healthcare, but ensuring that these technologies actually do so requires oversight.
McGinnis pointed out that generative AI, in particular, is susceptible to a phenomenon called ‘hallucination,’ which occurs when a model generates false or manufactured information.
A June 2023 paper published in The American Journal of Medicine highlights the potential challenge this creates in healthcare – cautioning clinicians and biomedical researchers to not ask the LLM ChatGPT for sources, references, or citations because of the model's tendency to hallucinate.
While generative AI developers are working to tackle issues like hallucination and the credibility of an LLM’s responses, these problems have been persistent across models and development companies.
McGinnis indicated that these issues will likely be resolved in the future, but in the meantime, more education around AI is key, particularly in healthcare.
“It's important for us, as a society, to know what goes into these models, what goes into training them, that they are well tested, that they are proven to be safe, and that they're trained on the right audiences,” he explained. “People differ, and it's important that the algorithms that are developed accommodate those differences.”
One of the projects currently underway at NAM originated from this idea after researchers found that algorithms used to develop clinical predictive models were drawing upon narrower information bases than necessary to treat different groups of people, potentially leading to significant patient harm.
This necessitated the development of the AICC framework.
THE AI CODE OF CONDUCT
McGinnis emphasized that despite its name, the AICC is more of a flexible framework than a rigid code of conduct, as the rapid evolution of AI in healthcare requires a more dynamic approach to governance than some other technologies.
“Things are changing so rapidly that the notion of a concrete code of conduct that would be durable over time and unchanging is shortsighted and impractical,” he underscored.
Thus, the framework is designed for use in the processes related to developing content for algorithms and the algorithms themselves. Further, since the AICC was created around the same time as the advent of generative AI and LLMs, it also incorporates implications and considerations from the rise of those technologies.
“The first step in [the framework] is to develop the key categories for assessing the algorithms that go into developing an artificial intelligence application,” McGinnis indicated, explaining that part of this involves anticipatory work from stakeholders to determine how an AI is applied to clinical care and how it might be applied in the future.
This is where the flexibility provided by the framework can help, as technologies like generative AI have a wide variety of potential applications. Developing a hypothetical set of “down the line” applications that can change as time passes allows stakeholders to better understand and conceptualize an AI’s real-world applications while leaving room for change as the technology advances.
The next step is to consider the kinds of programs that must be developed for each of the categories of a given AI application and to explore how those programs must be tested depending on said application.
This involves screening what goes into the structure of an algorithm and deciding what information will populate the elements of the structure. In both parts of the process, McGinnis emphasized that transparency is critical.
“There needs to be transparency in the structure, in the content that the algorithm is being populated with, and in the process that's used to test for safety, because, especially with generative AI, the technology's new, dynamic, and progresses quickly,” he said.
He further noted that at this stage, AI is an assistant to providers, but it has significant potential if governed appropriately.
“This technology is democratizing at some level because it allows access to a similar richness of the body of information, evidence, and data to everybody. It will be able to filter that information in a fashion that is understandable to the appropriate target.”
McGinnis also highlighted that allowing care teams to have better access to a wider breadth of high-quality information enables them to take a more collaborative approach to care.
“The way medical care works best is if the patient, the clinician, and the family are real partners, dealing more from a level playing field, even though the experience of the clinician is going to be able to add the nuance that's so fundamentally important as [healthcare organizations] tailor care to individual circumstances,” he explained.
For this to happen, though, safeguards like those outlined in the AICC, the White House Blueprint for an AI Bill of Rights, and Biden’s Executive Order on Trustworthy AI must be enacted in healthcare. To that end, NAM is working with multiple government agencies and other healthcare stakeholders to help put together the pieces of the healthcare AI governance puzzle.
“Our fundamental goal is to help this technology reach its full potential for improving the human condition, which means that it has to be safe and effective and tailored to every individual,” McGinnis stated.