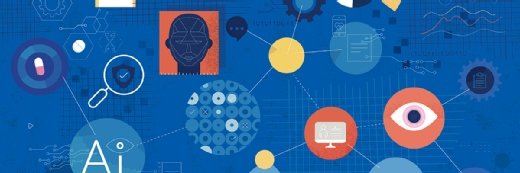
DrAfter123/DigitalVision Vectors
Eliminating Racial Bias in Algorithm Development
Artificial intelligence can assess patient risk and guide providers to optimal treatment options, but developers must ensure the technology doesn’t perpetuate existing biases.
The use of machine learning, natural language processing, and neural networking artificial intelligence technologies are exploding across the healthcare industry. From identifying early stage cancer on radiology images to calculating complex risk scores that guide providers to the best treatment options, artificial intelligence has broad implications for the future of healthcare.
However, the conclusions these technologies draw is only as good as the data used to develop them. Messy or biased data generates messy and biased results.
A recent study demonstrated this, identifying racial bias in one of the most common algorithms the industry uses.
“There’s growing concern around AI, machine learning, data science, and the risk of automation reinforcing existing biases through the use of algorithms. It was a confluence of what we know is a potential concern,” said Brian Powers, MD, MBA, physician and researcher at Brigham and Women’s Hospital and lead author of the study.
Results showed that an algorithm commonly used to identify eligibility for care management programs reduced the number of black patients identified for extra care by more than half. Removing this disparity would result in a 28.8 percent rise in black patients receiving additional services.
The bias was not intentionally introduced, Powers explained. When the algorithm was developed, healthcare costs were used as a proxy measure for health needs. Because black patients typically spend less money on healthcare, the algorithm underestimated the risk for these individuals.
In fact, Powers’ team was not originally looking for disparities when they came across the results.
“We sort of stumbled on the preliminary findings,” Powers noted. “The original project focused on risk prediction for high-risk care management programs. The algorithm is trying to solve is if there was a better way to more accurately predict risk, cost, and other variables.”
Early data identified a large discrepancy between the average risk score for black patients compared to white patients.
“It was unusual enough that it prompted us to think a little more deeply,” Powers said.
When they further investigated, Powers and his team discovered the risk score predicated for white patients was higher than for black patients at any given level of health.
“The reason for this is because at any given level of health or sickness, black patients, on average, consume fewer healthcare resources. That’s where using cost as a proxy for health breaks down,” he said.
The results were irrespective of if the patient had a chronic condition, how well their health status was controlled, or any other marker of good health.
“You can predict costs pretty well and the algorithm predicts costs irrespective of race,” Powers explained. “The algorithm does a good job predicting costs for black and white patients. Where it breaks down is whether or not cost is a good proxy for need or illness.”
The algorithm is currently used by health systems and insurers to allocate extra resources to patients and predict future healthcare costs. So, using cost as a proxy measure seemed like a logical choice.
“The health system, insurers, and society at large clearly want to focus on controlling costs. It seemed like a reasonable proxy at the time,” Powers said.
However, selecting this proxy measure had inherent bias.
“We believe it’s not unique to this algorithm,” Powers furthered. “Algorithms used by most health systems and insurance companies at this point are perpetuating existing racial biases.”
Not all hope is lost, though, Powers noted.
“It’s actually relatively easy to fix,” he explained. “You’re using cost as a label as opposed to something else like number of chronic conditions or poor control of chronic conditions.”
Powers and his team emphasized how selecting a different proxy could remove racial bias.
“Instead of looking at costs alone, you have to look at more reasonable or more proximate measures of health and healthcare needs,” he stated. “That’s the easiest solution. It requires changing the algorithm and changing the way in which they’re implemented.”
In order do this, Powers said developers need to partner with physician experts to understand the best proxy measures for the desired outcomes.
“Work with physicians to really crystallize what the prediction problem is. What is the outcome that is actually the best proxy measure for the program or allocation decision you’re making? Spend some time at that phase of development,” he recommended.
The design phase is critical when building advanced prediction algorithms.
“Actually understanding the prediction problem is a much more fundamental step,” Powers said. “For algorithm developers this means working more closely with folks involved in care delivery.”
To Powers, this problem is greater than a single algorithm.
“We do think that this is truly endemic. It’s not specific to how the algorithm is tuned. It’s a much more fundamental issue,” he said.
If you do not code for unconscious bias in the development stage, algorithms will perpetuate bias.
“There’s absolutely a place for algorithms. What this study showed us is these types of tools are really widespread and have become essentially ubiquitous without enough attention to potential downsides,” Powers articulated.
The biggest conclusion their work drew was not the inherent bias in many of the algorithms used to predict cost of care and risk, but the simplicity of eliminating this bias. Improving the efficiency, effectiveness, and equity of these tools is less daunting then it might appear.
To help developers overcome this problem, the University of Chicago announced the Center for Applied Artificial Intelligence.
“The new initiative will work with algorithm developers and health systems to help address racial bias in these algorithms,” Powers concluded.