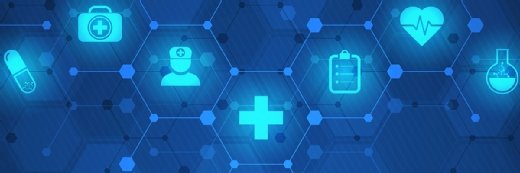
bestbrk/istock via Getty Images
Can Digital Twin Neighborhoods Help Tackle Health Disparities?
Cleveland Clinic and MetroHealth are building digital twins to better understand how patients’ neighborhoods impact their health outcomes.
Addressing health disparities and improving patient outcomes are key to achieving health equity, but tackling these issues requires health systems to understand their population’s needs and develop strategies to meet them.
Approaches to eliminate the disparities caused by a lack of health equity typically focus on the social determinants of health (SDOH), the non-medical factors that can affect a person’s health, such as where they live or work.
Research suggests that overcoming these issues requires a place-based population health approach, which asserts that improving community conditions can lead to improved health outcomes. There are multiple approaches to place-based health, as described in one 2020 JAMA article, but one promising solution proposes that health systems should focus their population health efforts on high-risk neighborhoods.
Recently, Cleveland Clinic and MetroHealth researchers received a $3.14 million grant from the National Institutes of Health (NIH) to develop a digital twin-based, neighborhood-focused model to reduce disparities in their patient populations.
To discuss the work and how it could help improve patient outcomes, HealthITAnalytics spoke with Jarrod Dalton, PhD, director of Cleveland Clinic’s Center for Populations Health Research, one of the leaders on the project.
USING DIGITAL TWINS TO UNDERSTAND HEALTH INEQUITY
McKinsey & Company defines a digital twin as “a digital representation of a physical object, person, or process, contextualized in a digital version of its environment,” allowing organizations to simulate the potential outcomes of real-world scenarios and guide decision-making.
In healthcare, digital twins can represent genomic data, such as clinical phenotypes and ribonucleic acid (RNA) expression, Dalton indicated.
The Digital Twin Neighborhoods project, however, will synthesize de-identified health information taken from electronic health records (EHRs) to build digital replicas of real communities served by Cleveland Clinic and MetroHealth.
The virtual neighborhoods will incorporate biological, social, and geographic information — including SDOH data — to better understand place-based health and disparities in those communities.
“[Cleveland Clinic’s] interest, at least in this initial project, is in applying this concept of a digital twin to understand social inequality and inequity in health outcomes,” Dalton said. “There's been a lot of research in the last decade focused on documenting social inequality and inequity in outcomes, especially as they relate to race, ethnicity, and socioeconomic position. We’ve done a large number of studies that have, in various ways, documented individual and place-based inequities in health outcomes here in northeast Ohio.”
This research builds on that work, allowing Dalton and his team to document the health disparities in these populations and study the mechanisms behind why those disparities exist and how to address them.
“We believe that simulation through digital twins is one way to get at the question, ‘What if we perturbed the healthcare environment [around these neighborhoods] in particular ways? Does that translate to improvements in health inequities?’” he explained.
But before the researchers can answer those questions, they have to build the digital twin infrastructure.
BUILDING DIGITAL TWIN NEIGHBORHOODS
“We are defining a digital twin neighborhood as the health status over time of the population of people who live in a neighborhood,” Dalton stated.
He explained that digital twins are often used in urban design and city planning, where the tools simulate physical infrastructure and environment. Doing so can help stakeholders understand how, for example, the addition of a new roadway may impact transportation patterns within a community.
The Digital Twin Neighborhood project takes a similar approach, focusing on the health profile of a community and simulating how different interventions may impact health status and outcomes over time within a neighborhood.
To do this, each digital twin neighborhood uses algorithms to generate a synthetic population with characteristics that are as closely matched to those of the population in the real-world neighborhood in question, Dalton explained.
From there, the research team applies a variety of healthcare data and other sources of information to the digital twin neighborhood to represent exposures, risks of chronic disease development, how the exposures might relate to or influence the risk of disease development, and how these disease trajectories translate to increased risk of mortality and lower life expectancy.
The digital twins also utilize place-based information, as these factors play a significant role in community health.
“We think of neighborhood environments as a combination of several types of environments,” Dalton indicated, noting that there’s a social environment related to factors such as social support and partner status; the chemical environment, which includes the contaminants to which people are exposed and the weather patterns in the community; the built environment, which encapsulates access to transportation and other infrastructure; and the economic environment, which describes the job profiles, income, and housing status of community members.
Understanding these environments through digital twins can help researchers better understand the needs of the community they’re studying.
“We try to use simulation modeling to get a representative depiction of the exposures, health status, and outcomes of real people in the community. Although, our modeling is all based on synthetic or artificially generated individuals,” Dalton stated.
Using these digital twin neighborhoods, the research team is undertaking several projects to answer critical population health questions and guide further development of the digital twins.
PROJECTS USING THE DIGITAL TWIN NEIGHBORHOODS
“Right now, [the Digital Twin Neighborhoods] project focuses on establishing the methods, computational approaches, and infrastructure for producing these synthetic or digital twin populations,” Dalton said.
However, the researchers have proposed some initial projects to help anchor their thinking about developing the models and tackle the most relevant population health concerns.
The first of these projects seeks to assess regional mental health initiatives on a neighborhood level, particularly their impact on depression.
“Healthcare in the United States focuses on individualized care, and most of that is appropriately so, but that relies on patients that access healthcare systems and assumptions that prescribed interventions are equally effective across populations, that there are uniform barriers to carrying through with prescribed intervention, which we know is not the case,” Dalton underscored.
He further explained that in Cleveland, neighborhood disparities can be severe, disproportionately impacting some neighborhoods’ health outcomes more than others. In particular, areas with low socioeconomic positions and predominantly Black populations in northeast Ohio also often have poorer health status and experience hurdles to adhering to prescribed interventions.
“This depression-focused study is asking, ‘If we look at neighborhood-level interventions, beyond what might be ordinarily done in a person-to-person healthcare environment — improving access to medications and psychotherapy or expanding the role of virtual appointments — how might these modify the mental health profile and access to services in communities that have significant barriers to those things?’” he noted.
A second project focuses on evaluating the potential impacts of introducing new services on heart disease burden in communities. The initiative focuses on modifiable cardiovascular risk factor reduction.
“[Cleveland Clinic] and others have done studies that show significant neighborhood-level disparities in cardiometabolic disease,” Dalton said. “One of the most impactful modifiable cardiovascular risk factors is blood pressure, and we have significant problems in hypertension in low-income communities here in northeast Ohio.”
To help address this, the research team will use the digital twin neighborhoods to build a dynamic model for cardiovascular health status, which will help paint a clearer picture of how people's risk factors change across their lifespan. From there, the researchers will simulate the effects of real interventions to improve access to cardiovascular event prevention.
The complexity of these projects would make them costly, difficult, and resource-intensive to undertake on the ground in the real world, but digital twins can help researchers overcome some of those obstacles.
The digital twin neighborhoods allow Dalton and his team to model multiple outcomes that co-evolve over time within patients and across patient populations.
“We use dynamic, probabilistic models that we [develop] from electronic health records, and we do that in a way that looks at specific conditions and points in the life course, to build hundreds of models that try to represent risk of outcomes across the life course,” he explained.
Those models are then used to simulate life course outcomes in a given synthetic population. Dalton indicated that researchers might run anywhere from 30 to 1,000 of these simulations.
From there, model accuracy under known conditions, like neighborhood exposures and existing resources available in the community, is assessed to determine whether the tools can reproduce the outcomes observed within a population’s EHRs.
“This is how we try to begin to target mechanisms so that we can reproduce the phenomena that we're observing in our patient populations through mechanistic modeling,” Dalton stated. “Because once [researchers] have a mechanistic understanding, that's when they can go back to the healthcare systems, the insurers, and the relevant stakeholders to hopefully provide information that can be helpful to them in planning neighborhood-level and population health interventions.”
In the future, the research team intends to make the Digital Twin Neighborhoods database publicly available so that other researchers can leverage it to inform population health improvement initiatives.