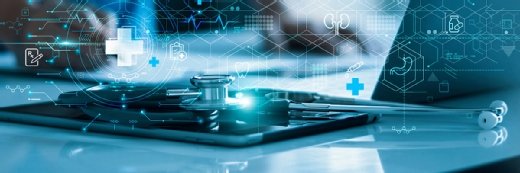
ipopba - stock.adobe.com
AI-Based Natural Language Processing Advances Precision Medicine
Washington University School of Medicine is improving precision medicine research with the adoption of artificial intelligence-based natural language processing tools.
To advance their precision medicine research, Washington University School of Medicine has adopted artificial intelligence-based natural language processing tools to assist in sorting their unstructured electronic health record data.
The school’s initial focus is on chronic diseases such as Alzheimer’s disease, breast cancer, lung cancer, diabetes, and obesity. With the AI-based tool, researchers can pull key information from electronic health records (EHRs) about diagnoses, treatments, and outcomes.
“Fundamentally, the challenge that we have with personalized medicine or precision medicine is that rather than treating patients as a function of how the average patient presents or the average patient may respond to therapy, we instead want to understand the individual features of each unique patient that contribute to both wellness and disease, and response to therapy,” Philip Payne, Washington University Associate Dean for Health Informatics and Data Science, told HealthITAnalytics.
According to Payne, there needs to be a substantial amount of both historical data and data from those currently participating in studies to develop personalized medical approaches.
Much of the work performed at Washington University around informatics is in the context of deep phenotyping. Meaning both medically discovered data and social determinants of health data needs to be extracted from electronic health records (EHRs).
“We need to be able to identify all those data, connect all those data to one another and then understand them in that multi-scale context, which is very complex from a computational standpoint. That's really what we do in the Institute for Informatics. We work very hard to discover those data sources, to integrate them, to harmonize them and understand them,” Payne said.
While extracting the clinical details is an important part of advancing precision medicine, there are some complexities. According to Payne, much of the data that is critical to understand both health and disease is not captured in discrete or structured fields in EHRs, making the data difficult to apply to machine learning methods.
To access EHR unstructured data and narrative text, Washington University used natural language processing (NLP).
“Natural language processing effectively teaches computers to learn and read. It's not entirely different when we talk about imaging data. Humans looking at an image can point to a spot or a lesion in a chest CT, for example. What we have to do is train a computer to recognize that same pattern and create a discrete field in which there is a lesion and where it is anatomically located. How large is it and how certain are we that it's there,” Payne continued.
“That's about teaching computers to interpret pictures. A lot of what we do is teach the computer to read or teach the computer to interpret pictures so we can get those structured features back out and put them into our predictive models.”
According to Payne, Washington University has adopted the use of AI and NLP tools to advance its biomedical practices. This includes machine learning and deep learning to identify patterns in data and predictive analytics to determine patient outcomes.
“There's great promise there in that these types of algorithms allow us to identify these high order patterns in data that more traditional statistical modeling and testing approaches do not allow us to identify. We know that in both health and disease, the patterns that exist around the interaction of genes, gene products, clinical features, people's behaviors, and their environments are very complex,” Payne continued.
“There’s unlikely to be sort of a single indicator that will tell us whether or not a patient is or is not going to experience a disease state, but rather it's a confluence of all these different indicators that help us to predict outcomes, and that's where the power of machine learning and other AI methods become all that much more important.”
The real challenge with these systems is while providers are very excited about the promise of AI-based methods, they still need to be subjected to rigorous examination and training, according to Payne. Additionally, algorithms must be build using diverse populations to reflect all individuals served to predict outcomes.
“There’s both the promise and the challenge of AI. The promise is understanding these complex patterns that may predict outcomes, we can and should explore that. The challenge is making sure that we are empirically and rigorously testing those algorithms to make sure they're appropriate to use for clinical decision making. That's the area where a lot more work is needed, and we’re engaged in that work now,” Payne said.