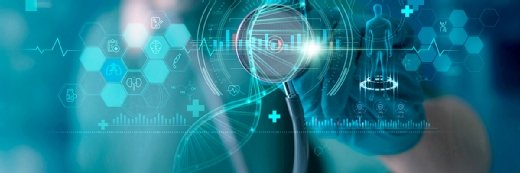
ipopba/istock via Getty Images
7 challenges of AI integration in healthcare and their remedies
The healthcare sector faces many hurdles when adopting AI. Obstacles include setting an AI strategy, dealing with fragmented data, and addressing ethics, security and compliance.
The integration of artificial intelligence in healthcare has been long coming, dating back to at least the 1980s, when expert systems were touted as a potential diagnostic tool.
Those early efforts fell far short. But some 40 years later, the mass adoption of AI in healthcare is becoming much more realistic. The technology, especially with the arrival of generative AI (GenAI), is poised to become widely integrated into the workflows of providers, payers and life sciences companies. Use cases run the gamut from clinical documentation to revenue cycle management.
That said, technology adopters must overcome several obstacles if they hope to realize the benefits of AI.
Top challenges of AI in healthcare
Key tasks ahead include establishing an AI strategy, wrangling data, providing security, overcoming users' skepticism and managing change.
1. Understanding AI and setting the strategy
The initial obstacle for healthcare organizations considering AI adoption is grasping the essentials of the technology.
A recent AI roundtable, hosted by consultancy EY, underscored that point. The event drew an audience of healthcare providers and insurers, mostly at the director level, said Kim Dalla Torre, EY's global and Americas health leader. "Where we had to start in the room was with a definition of the difference between AI, in a broad concept, and GenAI," she explained. "The challenges start with first understanding exactly what it is and how to apply it."
With that foundation established, the next step is to develop an AI strategy and vision, noted Mahmood Majeed, managing partner at consulting and technology firm ZS, who leads the company's global digital and technology services practice. A key question a healthcare organization must address when creating a strategy is whether its goal is value capture or value creation, Majeed added. In value capture, a healthcare organization harnesses AI for an existing business and seeks to reduce costs, improve decision-making or improve patient engagement, he said. Value creation, on the other hand, involves developing new sources of revenue -- for example, a healthcare company that launches a data business.
Dr. Bill Fera, a principal at Deloitte Consulting, said he encourages clients to take a portfolio view of GenAI projects and consider ROI across three dimensions: financial, experience and satisfaction. A clinical documentation project might boost physician satisfaction and patient experience, while a revenue management cycle initiative could generate a significant financial return. "Taken as a whole, across your portfolio, you should see returns in each of those buckets," Fera surmised.
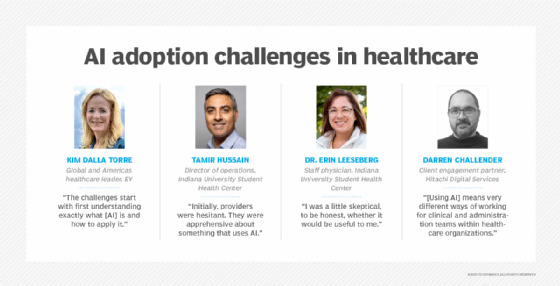
2. Creating an AI team to guide deployments
Selecting AI use cases with the greatest ROI potential requires the ability to define and gauge success. Measuring the technology has been a sticking point, however.
"Historically, organizations have not been great about measuring success from the beginning, baselining and tracking to make sure that the hypothesis behind the deployment of the AI or GenAI tool is actually realizing the value we anticipated," Fera said.
An AI governance structure can help healthcare organizations identify use cases, deploy projects and track their progress. That structure must include all the relevant stakeholders, including clinicians, business leaders and finance executives. "That multidisciplinary team," Fera explained, "is going to be important to make sure we are measuring across the important dimensions."
That team has often been called a "governance organization." But the label has become a bit problematic: AI governance has come to be seen as AI gatekeeping, emerging as one of the top AI challenges across industries. Ideally, the group responsible for governance provides structure without stifling innovation. "The balancing act," Fera noted, "is to make sure that group is nimble and is seen by the organization as an accelerator, rather than an onerous 'governance process' that is there to stop progress." Alternative names for governance teams, he added, now include "accelerators" or "catalyzers."
3. Overcoming data fragmentation
Health IT experts often point to data as being among the leading AI challenges in healthcare. "The one unifying principle that bubbles very much to the top of the list is the issue of data fragmentation across systems, locations and formats," said Dr. Scott Schell, chief medical officer at IT consulting and outsourcing services company Cognizant.
This fragmentation makes data difficult to use in AI models and can lead to poor results. "AI depends on reliable and replicable data to be adequately trained so it can perform reliably and avoid hallucinations," Schell said.
The varied data formats in the healthcare sector are particularly vexing. Providers, payers, pharmacies and testing laboratories, for example, employ a multitude of standards to house data. International Classification of Diseases (ICD)-11; Logical Observation Identifiers, Names, and Codes (LOINC); and Systematized Nomenclature of Medicine - Clinical Terms (SNOMED-CT) are just a few of the formats in use.
Amid the babel of technical dialects, healthcare organizations expend enormous effort gathering, cleaning and harmonizing their data so AI can make sense of it, said Shrikanth Shetty, chief growth officer and global head for life sciences and healthcare industries at IT consultancy HCLTech. "You have to have data in a shape and form that AI can consume," he reasoned. "Otherwise, it will be junk in, junk out."
One way forward is the adoption of a healthcare data harmonization model. Schell said he sees the Observational Medical Outcomes Partnership (OMOP) Common Data Model as one of the more promising examples. "Using OMOP as a template to rank and order the data," he explained, "you can then funnel the data out of these existing [data] infrastructures into this regulated format."
Other models in use include the Clinical Data Interchange Standards Consortium (CDISC) Foundational Standards and National Patient-Centered Clinical Research Network (PCORnet) Common Data Model.
4. Dealing with ethics, compliance and security
Trust and ethical issues are huge concerns for AI in healthcare, Majeed noted. Ethical AI uses aims to ensure AI models don't reflect bias that could skew data to the detriment of racial or ethnic groups. Majeed put the question to AI adopters: "How can you not inherit biases from your training data that could lead to disparity in diagnoses [and] to inequity in treatment?"
In addition to ethical considerations, healthcare organizations must factor regulatory compliance into their AI projects. HIPAA, FDA's Part 11 regulations, and the EU's GDPR and AI Act are just some of the compliance regimens. "As a regulated industry," Majeed added, "there's a lot of focus to make sure data is protected and secured and used in the right way."
The security and privacy of AI models call for appropriate boundaries. "You need to have models that live inside your organization and create boundaries where access to outside public models isn't possible," Schell said, "because that obviously represents an opportunity for data leakages or privacy breaches."
Proactive security is also critical. That means baking security and patient data privacy into AI systems as they're being built rather than after the fact. "We need to focus on these things as part of the initial design, included in the core DNA, and move away from a reactive or post-implementation deployment," Schell said. "The time of design is the easiest opportunity to find the holes and vulnerabilities.
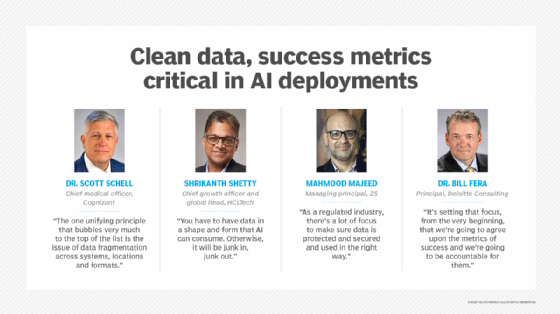
5. Addressing adoption concerns
AI deployments, no matter how well managed, will ultimately fail if employees don't use the tools. Healthcare providers are generally wary of any technology that could disrupt patient care. AI is no different.
But healthcare organizations that cultivate early adopters can overcome initial uncertainty. Indiana University's Student Health Center began offering an AI-based clinical documentation application to its providers in the spring of 2024, said Tamir Hussain, the center's director of operations. The application, called Sunoh, is a product of Sunoh.ai. "Initially, providers were hesitant," he noted. "They were apprehensive about something that uses AI."
Tamir organized 5- to 10-minute demos for providers, and the first adopters began using the cloud-based product. One early user is Dr. Erin Leeseberg, a staff physician at the center. "I was a little skeptical, to be honest, whether it would be useful to me," she said.
Leeseberg decided to try Sunoh in the summer, when patient loads are lighter. She found that the AI tool concisely transcribed her conversations with patients and reduced the time she spent typing clinical notes. She now uses Sunoh to document all her patient encounters.
Early users recommended the AI transcriber to their peers, Hussain reported, and adoption took off from there. More than 30 providers at the center now use the tool, including physicians, nutritionists and physical therapists.
Integrating AI into a well-established workflow helps with adoption. Note-taking is one example, according to Fera. "Physicians have been historically comfortable with the workflow of dictating," he said.
Adding AI to a health plan's call center workflow is another case in point. "Having that digital assistant on the call with the call center agent as well as the member -- again that's part of their normal workflow," Fera said. "Where they would be using a computer to find information while on the phone, now the information is being brought to them while they are on the phone."
6. Expanding AI capabilities
An AI offering might address most users' needs out of the box, but there's likely to be room for improvement in an emerging technology field.
Sunoh's AI transcription tool is "already fantastic," Leeseberg said, but she hopes the vendor will make it work better for certain types of patients. The product excelled at taking notes on patients with straightforward medical histories, she explained, but it wasn't as effective when documenting longer, more complicated histories. For those patients, she said, the tool's history-of-present-illness notes amounted to three or four sentences. To add more detail, she re-recorded a patient's history and used the second transcription to update her notes.
Leeseberg said she anticipates many new versions of the product down the road and believes her feedback -- and input from other customers -- will improve the tool's AI capabilities.
7. Helping employees through the AI transition
AI and workflow automation change how employees do their jobs. "It means very different ways of working for clinical and administration teams within healthcare organizations," said Darren Challender, client engagement partner at Hitachi Digital Services.
New ways of doing business come with fears of obsolescent skills or vastly changed -- or eliminated -- roles. Against that backdrop, training and change management are key to the process of integrating AI, Challender said.
But organizations must consider the effects of AI on patients and their families -- as well as healthcare workers. Patient outcomes could be at stake if AI disrupts the daily interactions among the parties. "If we deploy AI and these are lost," Challender cautioned, "we will impact the delivery of care."
Majeed said healthcare organizations need to invest in a range of training and talent initiatives, citing AI literacy programs, hands-on training and industry certification to name a few. Ideally, organizations should instill a culture of continuous learning that's personalized for employees. "There are journeys for every person in the company," Majeed said.
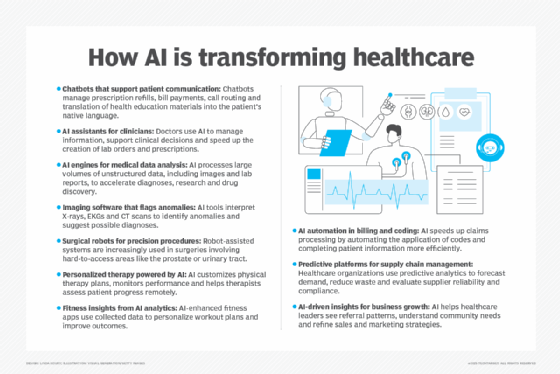
Strong medicine?
Adopting AI can potentially help healthcare organizations cut costs, improve patient care and relieve providers of manual tasks such as documenting patient visits. That's if all goes well. Poorly managed AI deployments could result in several dangerous side effects. The list includes biased data, security breaches, patient privacy violations and disrupted staff-patient communications. From a financial standpoint, ill-conceived and hastily executed projects are unlikely to generate ROI.
But providers, payers and life sciences companies can mitigate the risks of AI integration. A focus on ethics, security, data quality and user adoption can keep initiatives on track. And a strategy that incorporates clearly stated goals and ways to track progress puts a project on solid ground from the start.
Such practices aren't a secret, but it's up to the AI adopters to embrace them. "It's setting that focus from the very beginning that we're going to agree upon the metrics of success and we're going to be accountable for them," Fera said. "I think people know what to do. They just haven't always done it."
John Moore is a writer for Informa TechTarget covering the CIO role, economic trends and the IT services industry.