Planning for GenAI disillusionment
Hype around GenAI will inevitably be followed by generative AI disillusionment. Experts ruminate on how to shorten the trough and prepare for the future.
The capabilities of a new wave of generative AI tools have spurred massive investment. But, even as the investment dollars keep pouring in and companies build business strategies around this emerging technology, alarm bells about a trough of disillusionment, a crash or even a third AI winter are being sounded in some quarters.
Take, for example, the misgivings of AI pioneer Gary Marcus, who recently argued that a rumored $100 billion GenAI project planned by Microsoft and OpenAI might be the second worst AI investment ever -- just behind the more than $100 billion invested in the fleets of driverless cars that have so far failed to generate meaningful returns. The root problem, according to Marcus, is the widespread false belief that GenAI is tantamount to artificial general intelligence, or intelligence that is as smart and resourceful as humans.
Marcus is not alone. Experts interviewed for this article concurred that GenAI expectations often don't match opportunities for a host of reasons. Hasty adoption without clear business objectives has resulted in misaligned efforts, inefficient resource allocation and underutilization of talent, said Udo Sglavo, vice president for applied AI and modeling R&D at SAS. In addition, neglecting trust considerations, bias mitigation and privacy safeguards has exposed companies to reputational risks and potential legal liabilities, spotlighting the imperative of ethical AI development and deployment.
"Unrealistic expectations have led to disappointment and frustration when generative AI fails as the silver bullet in solving potentially complex problems," Sglavo said.
But the gap between hype and reality is not necessarily a bad thing, Sglavo and others argued. Understanding GenAI's capabilities and limitations requires painful analysis and possible financial restructuring by investors to take advantage of new opportunities, but that is par for the course for a new technology -- and in keeping with the history of AI.
Possible winter scenarios
The history of AI has included many AI winters or periods when investment, R&D and adoption slowed down due to unmet expectations. In 1973, professor Sir James Lighthill published a scathing report for the U.K. parliament that claimed AI technology was only suitable for toy applications. Around the same time, major U.S. government funding for AI projects dried up.
In the early 1980s, innovations in expert systems helped drive renewed interest in AI built on the Lisp programming language and specialized hardware. But many of these new Lisp startups failed, curtailing R&D and investment in a second AI winter.
Could unmet expectations in GenAI lead to another crash or winter, and if so, what might it look like?
Sglavo said a potential GenAI winter could entail reduced investment, industry consolidation and strategic realignment as enterprises recalibrate their AI priorities in response to unmet expectations, economic pressures and sustainability considerations.
Anton Dam, vice president of engineering, AI and machine learning at AuditBoard, said shelfware could be a big factor in whether there is another AI winter. When enterprises implement a new piece of software, there's often a burst of enthusiasm, he explained. "But, without proper change management techniques, that initial burst of usage tailors off, and users revert to their old habits while the new software collects dust."
Right now, expectations for AI are sky-high, Dam said. While the technology itself might be able to deliver and meet expectations, current funding and change management techniques haven't kept pace. "This gap could generate another AI winter," he said.
The more useful way to think about GenAI is not in terms of AI winters past, said Rohit Kedia, chief growth officer at tech consultancy LTIMindtree, but rather in the context of any new technology.
"Every technological innovation follows a pattern similar to the Gartner Hype Cycle, characterized by an initial phase of imperceptible progress, followed by rapid acceleration and, eventually, a plateau," Kedia said.
GenAI is currently ascending the steep incline of the hype cycle with a phase of swift advancement that will inevitably level off. However, this pattern does not signify an end but a natural progression.
"As the hype cycle continues, GenAI will become integral to daily lives, much like past technological revolutions for desktop computers and the cloud," Kedia said. Similarly, GenAI will no longer be a novelty, but a standard feature of the technological landscape. While there is no way to pinpoint the precise moment of this shift, its direction is unmistakable.
Some experts believe an AI winter might be a good thing. Davi Ottenheimer, vice president of trust and digital ethics at Inrupt, a secure data-sharing platform, said a fallow period for GenAI could bring clarity and, ultimately, yield better outcomes.
"Embracing the GenAI winter as a natural phase in evolution can lead to greater long-term stability and maturity in the field, much like how darkness brings real depth and richness to aspects of life. A winter could be reframed not as a setback, but as an opportunity to advance AI with better optimizations for social good," Ottenheimer said.
Shortening the trough of GenAI disillusionment
Meanwhile, enterprise execs must take a pragmatic approach to ride the wave of GenAI opportunities without crashing. Arun Chandrasekaran, distinguished vice president and analyst at Gartner, recommended enterprises adopt AI engineering practices that enable seamless scaling of pilots, such as Agile thinking, sandbox environments for rapid experimentation, composable platform architecture, FinOps and a product-centric delivery model. It's also important to put responsible AI at the heart of GenAI efforts and consider how to incorporate human feedback into GenAI applications, he said.
Companies need to appreciate that the infrastructure around GenAI is also evolving, Chandrasekaran said. Better models are certainly one aspect, as are data, governance and hardware infrastructure.
"While some level of disillusionment is inevitable, given the chasm between hype and reality, the underlying technologies are indeed progressing at breakneck speed," he said. "We will certainly see a recalibration of use cases, more emphasis on tangible business value and proactive risk mitigation steps."
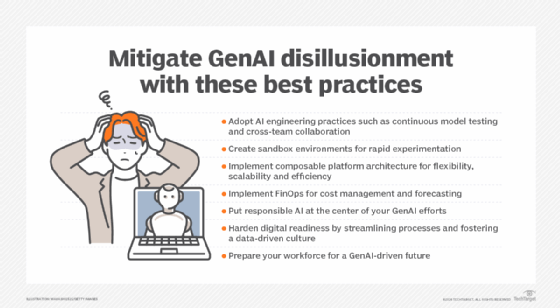
Shaping enterprise strategy
But foolproofing GenAI in the enterprise is not easy in the near term, Chandrasekaran cautioned.
GenAI adds value for most creative use cases, such as generating new marketing content or code to boost developer productivity. But GenAI systems are nondeterministic by nature. This means the AI can produce different outputs even when given the same input multiple times, leading to unpredictability in its results.
"While there are ways to boost accuracy and reduce hallucinations of these systems, it is largely a work in progress," Chandrasekaran said.
Adding yet another layer of complexity, the GenAI tools ecosystem is constantly in flux, making it difficult to choose the right tools and approaches, exacerbating supplier selection and rendering long-term technology plans obsolete.
While progress across use cases will likely shorten the trough of disillusionment, upcoming regulations and increased data acquisition and training costs at scale could lengthen it, Chandrasekaran said. In the near future, at least, Chandrasekaran sees enterprises struggling to formulate a solid GenAI strategy that aligns with business strategy, owing to a lack of understanding about evolving best practices.
Preparing for the future
Vishal Gupta, partner at Everest Group, said that maximizing the results of GenAI requires a thoughtful approach. He emphasized the need to ensure digital readiness by establishing the necessary technical maturity by streamlining processes and fostering a data-driven culture within the organization. This entails building a comprehensive data foundation and preparing the workforce for a GenAI-driven future. It is also important to establish a well-structured enterprise-wide risk and governance framework to successfully embrace GenAI, while mitigating potential risks.
Kedia pointed out that the rapid evolution of technologies like GenAI demands a collaborative approach. "Relying solely on one designated team within the enterprise will not allow it to rise to its full potential," he said.
Instead, organizations must combine the knowledge, experiences and diverse perspectives of various individuals, including partners, employees or external experts, to fuel innovation and keep ahead of the curve.
"Cultivating a culture of experimentation is equally crucial in harnessing GenAI effectively, so enterprises must be open to new approaches and embrace its possibilities," Kedia said.
George Lawton is a journalist based in London. Over the last 30 years, he has written more than 3,000 stories about computers, communications, knowledge management, business, health and other areas that interest him.